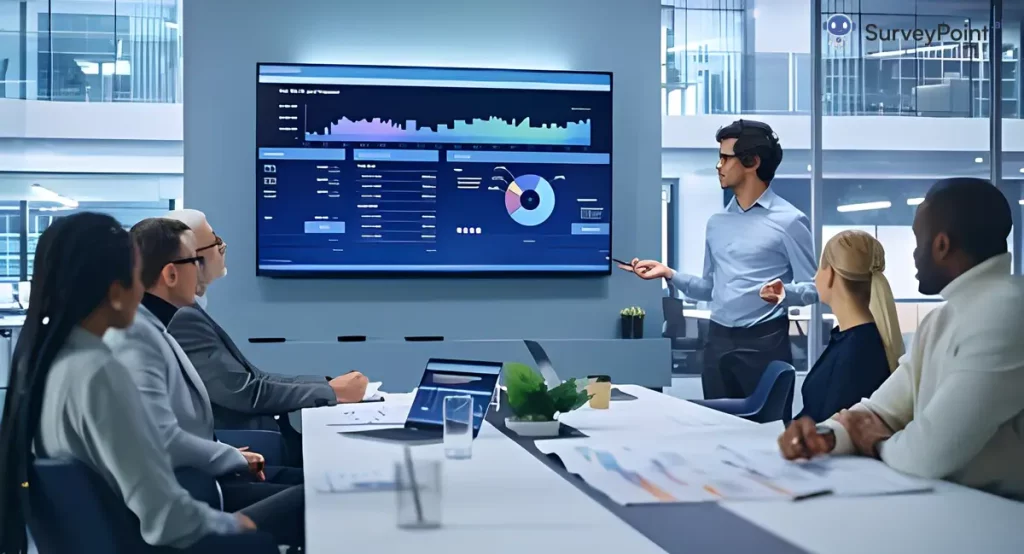
Explore the concept of sampling error in statistics and research. Understand how it can impact the accuracy of your findings and learn strategies to minimize its effects.
Are you confident in the accuracy of your data? As researchers, analysts, or decision-makers, we heavily rely on data to inform our choices and guide our actions. But what if there’s an underlying flaw that we often overlook? Enter sampling error, a crucial yet often misunderstood concept that impacts the integrity of our data. In this article, we will delve into the depths of sampling error, its causes, its effects on data analysis, and how you can mitigate its impact.
What is Sampling Error?
Sampling error refers to the discrepancy between the characteristics of a sample and the characteristics of the entire population it represents. When we collect data, it is often impractical or impossible to survey an entire population. Instead, we take a representative sample, and sampling error comes into play when the sample fails to fully mirror the population.
Factors Contributing to Sampling Error
Several factors can contribute to sampling error:
Sample Size
The larger the sample size, the smaller the sampling error tends to be. Larger sample sizes provide a more accurate representation of the population as a whole, reducing the impact of random variations.
Sampling Method
The manner in which we select our sample can influence sampling error. Random sampling methods, such as simple random or stratified sampling, tend to yield more representative samples and lower sampling error compared to convenience or voluntary sampling methods.
Population Variability
The greater the variability within a population, the higher the potential for sampling error. If a population has diverse characteristics or is subject to significant variations, it becomes more challenging to capture its true representation in a sample.
The Impact on Data Analysis
Sampling error can have profound implications for data analysis, leading to biased results, erroneous conclusions, and poor decision-making. Understanding the effects of sampling error is crucial for ensuring the accuracy and reliability of your findings.
Accuracy of Estimates
Sampling error introduces uncertainty into our estimates. The sample statistics may deviate from the population parameters, significantly affecting the accuracy of our estimates. It’s important to recognize that any statistical analysis is only as good as the sample it is based on.
Representativeness
Sampling error affects the representativeness of the sample. If the sample is not truly representative of the population, the conclusions or generalizations we draw from the data may not be applicable beyond the sample itself. This limitation can hinder the external validity of our findings.
Statistical Significance
When conducting hypothesis tests or inferential analysis, sampling error influences the p-value and statistical significance. Failing to account for sampling error may lead to false positives or false negatives, rendering the results misleading or inconclusive.
Sampling Bias
Sampling error can introduce sampling bias, where certain segments of the population are overrepresented or underrepresented in the sample. This bias can distort the results, obscuring the true relationships or patterns within the data.
Minimizing the Impact of Sampling Error
While sampling error is an inherent part of the data collection process, there are strategies we can employ to minimize its impact and enhance the accuracy of our findings.
Increase Sample Size
As mentioned earlier, larger sample sizes generally lead to smaller sampling errors. By increasing the sample size, you capture a more comprehensive representation of the population, reducing the influence of random variations.
Employ Probability Sampling Techniques
Utilizing probability sampling techniques, such as simple random sampling or stratified sampling, promotes randomness and ensures each element of the population has an equal chance of being selected. This approach enhances the representativeness of the sample, minimizing bias and improving the accuracy of the findings.
Understand the Population
Thoroughly understanding the population you are studying is crucial. By examining the characteristics, variability, and potential biases within the population, you can design a sampling strategy that better encompasses its diversity. This understanding allows you to address and mitigate potential sources of sampling error.
You Must Like Solid Waste Management Questionnaire: How to Create a Surprising Survey in 10 Minutes Data Analyst or Project Manager: Which One Has a Best Salary and Job Satisfaction in 2024? How to Become Certified Project Officer in 2024: The Ultimate Guide for Success
Statistical Techniques
Statistical techniques, such as margin of error calculations and confidence intervals, provide insight into the potential range of error in your estimates. By incorporating these techniques, you can express the uncertainty associated with your findings, promoting transparency and assisting in decision-making processes.
Frequently Asked Questions
Q: Can Sampling Errors Be Completely Avoided?
Sampling errors can’t be entirely avoided, but their impact can be minimized through careful sampling techniques.
Q: How Does Sample Size Affect the Accuracy of Data?
Larger sample sizes generally result in more accurate data, reducing the likelihood of sampling errors.
Q: Are Sampling Errors Common in Scientific Research?
Yes, sampling errors are common in scientific research, emphasizing the need for meticulous study design.
Q: Can Advanced Statistical Tools Eliminate Sampling Errors?
Advanced tools can help mitigate sampling errors, but they can’t eliminate them entirely.
Q: What Role Does Confidence Level Play in Sampling Error?
The confidence level reflects the likelihood of the true population parameter falling within the calculated interval—higher confidence levels reduce sampling errors.
Q: Is Sampling Error the Same as Sampling Bias?
While related, sampling error and sampling bias are distinct concepts. Sampling bias results from a non-random sample selection, contributing to errors.
Conclusion
Sampling error is a silent yet powerful force that can impact the credibility and accuracy of your data. By understanding its causes, effects, and implementing strategies to mitigate its impact, you can unlock the secrets hidden within your data. Remember, data analysis is a journey that begins with the thoughtful consideration of sampling error. So, embrace your role as a meticulous explorer of data, ensuring that the insights you uncover provide a solid foundation for informed decision-making.