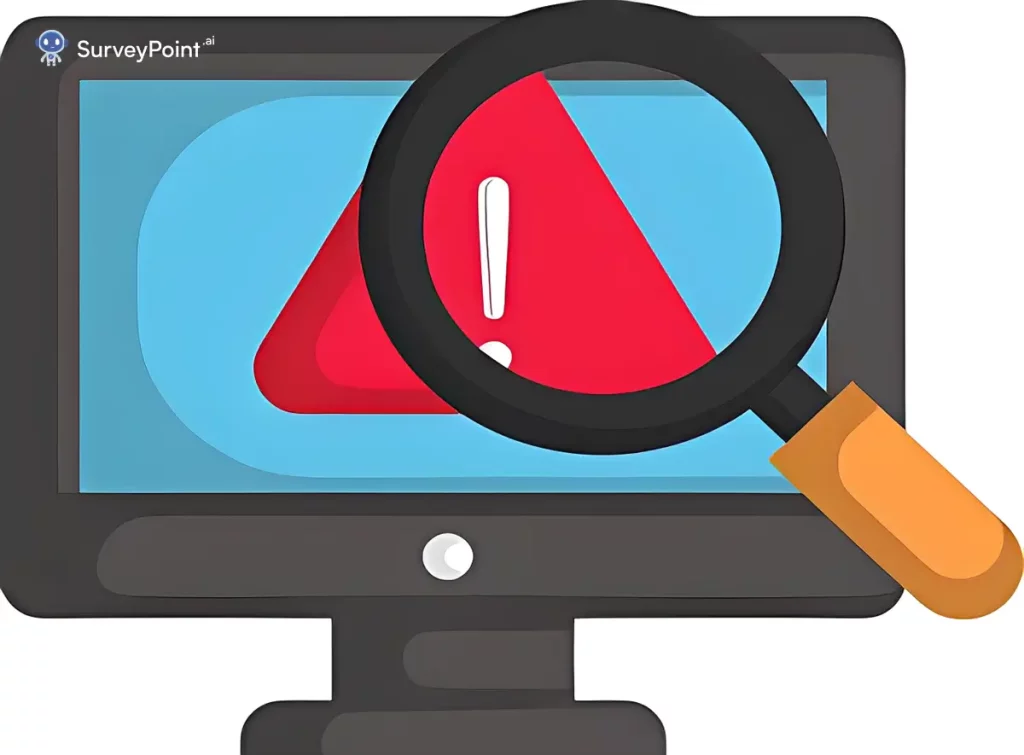
Unlock the secrets to effective research! Learn how to reduce sampling errors and enhance your research quality with these 5 simple techniques. Dive into expert insights that guarantee reliable results.
If you are a researcher, you know how important it is to collect and analyze data that accurately represents your population of interest. However, you also know how challenging it can be to achieve this goal, especially when you are working with a sample instead of the entire population.
A sample is a subset of the population that you select for your study. Sampling is a common and efficient method of data collection, as it allows you to save time, money, and resources. However, sampling also introduces the possibility of sampling errors.
A sampling error is a deviation or difference between a statistic obtained from a sample and the actual population value it estimates. Simply put, it is an error that arises because we are using a sample rather than the entire population. A sampling error does not indicate a mistake or flaw in the research design. It is an inevitable and unavoidable consequence of sampling.
Sampling errors can affect the validity and reliability of your research results, as they can introduce bias and uncertainty in your estimates. Therefore, it is essential to understand what causes sampling errors and how to minimize them as much as possible.
In this blog, we will discuss the following topics:
- What are the main types of sampling errors and how do they occur?
- How can you measure and report sampling errors in your research?
- What are some simple and effective ways to minimize sampling errors and maximize your research quality?
Types of Sampling Errors
There are different categories of sampling errors, depending on the source and nature of the error. In general, sampling errors can be placed into four categories: population-specific error, selection error, sample frame error, or non-response error.
Population-Specific Error
A population-specific error occurs when a researcher does not understand who to survey. This can happen when the researcher has a vague or incorrect definition of the target population, or when the researcher does not have enough knowledge or information about the population characteristics. For example, if a researcher wants to study the opinions of college students in India, but does not specify the criteria for selecting the students, such as age, gender, location, or field of study, the researcher may end up with a sample that does not reflect the diversity and heterogeneity of the population.
Selection Error
Selection error occurs when the survey is self-selected, or when only those participants who are interested in the survey respond to the questions. This can happen when the researcher uses a non-random or convenience sampling method, such as online surveys, voluntary response, or snowball sampling. For example, if a researcher posts an online survey on a social media platform and asks people to share their views on a political issue, the researcher may end up with a sample that is biased towards the opinions of those who are more active, vocal, or passionate about the issue.
Sample Frame Error
A sample frame error occurs when a sample is selected from the wrong population data. This can happen when the researcher uses an outdated, incomplete, or inaccurate list of potential participants, such as a phone directory, a voter registration list, or a membership database. For example, if a researcher wants to study the preferences of smartphone users in a city, but uses a phone directory that does not include the latest numbers or the types of phones, the researcher may end up with a sample that does not represent the current population of smartphone users.
Non-Response Error
A non-response error occurs when some of the selected participants do not respond to the survey or provide incomplete or inaccurate answers. This can happen when the researcher faces difficulties in contacting or reaching the participants, or when the participants are unwilling or unable to participate in the survey. For example, if a researcher conducts a mail survey and sends out questionnaires to a random sample of households, but only receives a low response rate, the researcher may end up with a sample that is biased towards the opinions of those who are more likely to respond, such as those who have more time, interest, or motivation to complete the survey.
You Must Know The Future of Survey Design: 7 Amazing Trends You Need to Know Python vs R for Data Analysis: Which One Should Learn in 2024?
Measuring and Reporting Sampling Errors
Sampling errors can be measured and reported using various statistical methods and indicators, such as the standard error, the confidence interval, the margin of error, and the coefficient of variation. These methods and indicators help the researcher to quantify the degree of uncertainty and variability in the sample estimates, and to communicate the level of confidence and precision in the research results.
Standard Error
The standard error is a measure of the variability or dispersion of the sample statistic around the population parameter. It is calculated by dividing the standard deviation of the population by the square root of the size of the sample. The standard error indicates how much the sample statistic is likely to differ from the population parameter due to sampling error. The smaller the standard error, the more accurate and reliable the sample estimate is.
Confidence Interval
The confidence interval is a range of values that contains the population parameter with a certain level of confidence. It is calculated by adding and subtracting a margin of error from the sample statistic. The margin of error is the product of the standard error and the Z-score value, which is based on the confidence level. The confidence level is the probability that the confidence interval covers the population parameter. The higher the confidence level, the wider the confidence interval, and vice versa. The confidence interval indicates how precise and certain the sample estimate is.
Coefficient of Variation
The coefficient of variation is a measure of the relative variability or dispersion of the sample statistic. It is calculated by dividing the standard error by the sample statistic and multiplying by 100. The coefficient of variation expresses the standard error as a percentage of the sample statistic. It is useful for comparing the variability of different sample statistics, especially when they have different units or scales. The smaller the coefficient of variation, the more consistent and stable the sample estimate is.
Ways to Minimize Sampling Errors
Sampling errors are unavoidable, but they can be minimized by using some simple and effective strategies, such as the following:
- Define your target population clearly and precisely, and make sure that your sample is representative of the population characteristics and features.
- Use a random or probability sampling method, such as simple random sampling, stratified sampling, or cluster sampling, and avoid using a non-random or convenience sampling method, such as online surveys, voluntary response, or snowball sampling.
- Use a large and adequate sample size, and make sure that your sample size is proportional to the population size and the level of variability in the population.
- Use a reliable and updated sample frame, and make sure that your sample frame covers the entire population and does not include any duplicates or errors.
- Increase your response rate, and make sure that your survey design and administration are appealing, accessible, and convenient for the participants.
- Check and validate your data quality, and make sure that your data collection and analysis are accurate, consistent, and error-free.
FAQs
Q: Can sampling errors be completely eliminated?
A: While complete elimination is challenging, strategic measures can significantly minimize the impact of sampling errors, enhancing research reliability.
Q: How do I choose the right sampling technique for my study?
A: Consider the nature of your research, available resources, and the level of precision required. Tailor your sampling technique to align with these factors.
Q: Is technology foolproof in eradicating sampling errors?
A: While technology is a potent ally, proper implementation and validation are crucial. It complements human oversight but doesn’t replace it entirely.
Q: What role does statistical analysis play in minimizing sampling errors?
A: Statistical analysis serves as a guardian against sampling errors, providing a robust framework to identify, quantify, and mitigate discrepancies.
Q: Can a small sample size yield reliable results?
A: Small sample sizes may suffice for certain studies, but they demand meticulous precision. Larger samples generally enhance the reliability of research outcomes.
Q: Why is continuous validation essential in research?
A: Continuous validation ensures that data integrity is maintained throughout the research process, identifying and rectifying errors promptly.
Q: What are the two types of sampling error?
A: There are two main types of sampling error: random sampling error and systematic sampling error. Random sampling error is the error that arises due to the natural variation in the sample. Systematic sampling error is the error that arises due to the bias or flaw in the sampling method.
Q: What is the most common sampling error?
A: One of the most common sampling errors is selection error, which occurs when the sample is not representative of the population or is self-selected by the participants. This can lead to biased and inaccurate results.
Q: What is sampling and non-sampling error?
A: Sampling error is the error that occurs when a sample is used to estimate a population parameter. Non-sampling error is the error that occurs due to other factors, such as measurement error, data processing error, or human error. Non-sampling error can affect both samples and populations.
Q: What is sampling error PDF?
A: Sampling error PDF is a document that explains the concept, types, causes, and effects of sampling error in statistics. It may also provide formulas, examples, and solutions to minimize sampling error.
Q: What is sampling error formula?
A: The sampling error formula is used to calculate the degree of uncertainty and variability in the sample estimate. It is given by: \\text {Sampling Error}=Z\\times\\frac {\\sigma} {\\sqrt {n}}, where Z is the Z-score value based on the confidence level, \\sigma is the population standard deviation, and n is the sample size.
Q: What is the cause of sampling error?
A: The cause of sampling error is the difference between the sample and the population. This difference can arise due to the natural variation in the sample, or due to the bias or flaw in the sampling method.
Q: What is sampling error and examples?
A: Sampling error is the deviation or difference between the sample statistic and the population parameter. For example, if the mean height of a population is 170 cm, but the mean height of a sample of 100 people is 165 cm, then the sampling error is 5 cm.
Q: What is the symbol for sampling error?
A: The symbol for sampling error is usually denoted by E or SE, which stands for error or standard error.
Q: What are the different types of sampling method?
A: There are different types of sampling methods, depending on the way the sample is selected from the population. The main types are probability sampling and non-probability sampling. Probability sampling is when each element of the population has a known and equal chance of being selected. Non-probability sampling is when the selection is based on convenience, judgment, or other criteria.
Q: What are the 4 types of non-sampling errors?
A: The four types of non-sampling errors are measurement error, processing error, coverage error, and non-response error. Measurement error is the error that occurs when the data collected is inaccurate or inconsistent. Processing error is the error that occurs when the data is coded, entered, edited, or analyzed incorrectly. Coverage error is the error that occurs when the sample frame does not match the target population. Non-response error is the error that occurs when some of the selected participants do not respond or provide incomplete or inaccurate answers.
Q: What are the 4 types of sampling?
A: The four types of sampling are simple random sampling, stratified sampling, cluster sampling, and systematic sampling. Simple random sampling is when each element of the population has an equal chance of being selected. Stratified sampling is when the population is divided into homogeneous groups and a random sample is taken from each group. Cluster sampling is when the population is divided into heterogeneous groups and a random group is selected and surveyed. Systematic sampling is when a fixed interval is used to select the elements from the population.
Q: What are common non-sampling errors?
A: Some of the common non-sampling errors are:
Poorly designed or unclear questions that confuse or mislead the participants.
Inappropriate or biased wording or order of the questions that influence the participants’ responses.
Lack of training or supervision of the data collectors or interviewers that affect the quality of the data.
Missing or incorrect data due to human or technical errors.
Low response rate or high non-response rate that reduce the representativeness of the sample.
Q: How do you control sampling errors?
A: Some of the ways to control sampling errors are:
Define the target population clearly and precisely, and make sure that the sample is representative of the population characteristics and features.
Use a random or probability sampling method, and avoid using a non-random or convenience sampling method.
Use a large and adequate sample size, and make sure that the sample size is proportional to the population size and the level of variability in the population.
Use a reliable and updated sample frame, and make sure that the sample frame covers the entire population and does not include any duplicates or errors.
Increase the response rate, and make sure that the survey design and administration are appealing, accessible, and convenient for the participants.
Check and validate the data quality, and make sure that the data collection and analysis are accurate, consistent, and error-free.
Conclusion
Sampling errors are inevitable and unavoidable in any research that involves sampling. However, sampling errors can be minimized and controlled by using some simple and effective strategies, such as defining your target population clearly and precisely, using a random or probability sampling method, using a large and adequate sample size, using a reliable and updated sample frame, increasing your response rate, and checking and validating your data quality.
By minimizing sampling errors, you can maximize your research quality and ensure that your results are valid, reliable, and generalizable to your population of interest. Sampling errors are not a threat to your research, but an opportunity to improve your research.
We hope that this blog has helped you to understand what sampling errors are, how to measure and report them, and how to minimize them. If you have any questions or comments, please feel free to share them with us. We would love to hear from you.