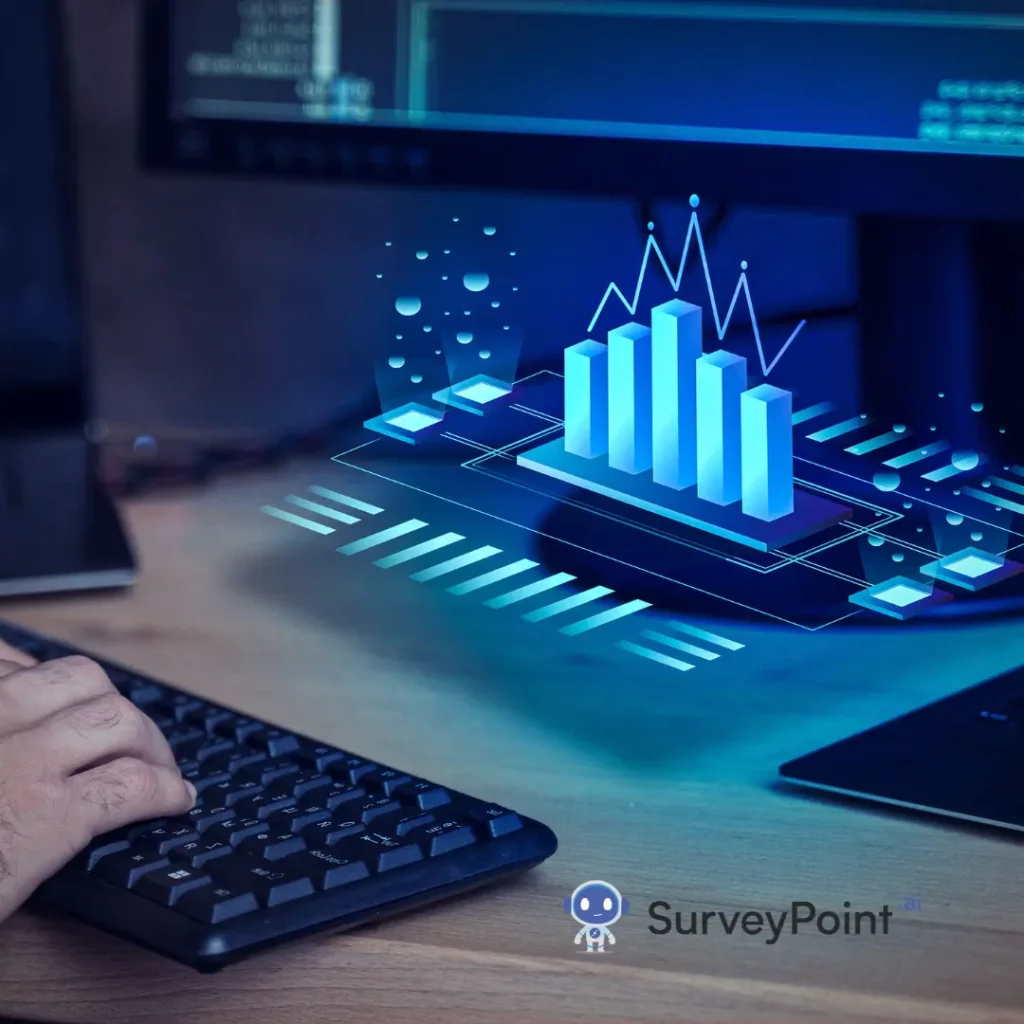
In the digital era, strategic data management has become the lifeblood of businesses, driving decisions and fueling innovation. However, managing vast amounts of data can be a daunting task without a well-defined strategy in place.
In this comprehensive guide, we will delve into the essential steps to creating a robust data management strategy, enabling organisations to harness the power of their data for better insights and informed decision-making.
1. Understanding Your Data Landscape:
Data Classification:
- Categorising data based on sensitivity, importance, and regulatory requirements is an essential aspect of data management. By assigning different classifications to various types of data, organisations can prioritise their efforts and allocate resources effectively.
- Understanding the lifecycle of each data type ensures that data is appropriately handled from creation to deletion, minimising risks and compliance issues.
Data Inventory:
- Cataloguing the types of data your organisation handles involves creating a comprehensive inventory. This process includes identifying databases, applications, and external feeds that contribute to your data ecosystem.
- Recognising the sources of your data is crucial for understanding where it originates and ensuring accurate documentation of its flow within your organisation.
Importance:
- A clear understanding of your data landscape forms the foundation for effective data management. It allows you to prioritise efforts, allocate resources strategically, and ensure compliance with data regulations.
2. Defining Data Governance Policies:
Data Quality Standards:
- Defining standards for data accuracy, completeness, and consistency is a foundational element of data governance. Establishing clear criteria for what constitutes high-quality data guides data management practices across the organisation.
- Implementing processes for data validation and cleansing ensures that data quality standards are consistently met, contributing to the reliability of analytics and decision-making processes.
Data Security Measures:
- Implementing access controls and encryption to protect sensitive data is paramount for maintaining data security. Organisations must define who has access to what data and establish encryption protocols to safeguard against unauthorised access.
- Establishing protocols for data sharing and collaboration ensures that security measures are not compromised during collaborative efforts, maintaining the integrity of sensitive information.
Data Ownership and Accountability:
- Assigning responsibility for different data sets within the organisation is crucial to ensuring accountability. Clearly defining roles and responsibilities helps maintain data quality and accuracy.
- Designating individuals or teams accountable for specific data sets fosters a culture of ownership, promoting proactive data management.
Importance
- Effective data governance policies instill confidence in stakeholders by ensuring data accuracy and security. This, in turn, facilitates better decision-making and compliance with industry regulations.
3. Implementing Data Integration Solutions:
Monitoring and Maintenance:
- Implementing monitoring tools for proactive issue detection is essential for maintaining the health of data integration workflows. Regularly monitoring data movements helps identify and address issues before they impact operations.
- Regularly updating and optimising integration processes ensures that they remain aligned with the evolving needs of the organisation. This includes incorporating new data sources and adjusting workflows to accommodate changing business requirements.
Establishing integration workflows:
- Creating seamless workflows for data movement and synchronisation involves mapping out the entire data integration process. This includes identifying the sequence of data movements and ensuring that they align with business requirements.
- Ensuring real-time or batch integration based on business requirements allows organisations to choose the most suitable approach for their specific use cases.
Choosing Integration Tools:
- Evaluating tools that align with your organization’s needs is a critical step in implementing data integration solutions. Considerations include scalability, ease of use, and support for diverse data formats to ensure compatibility with your existing infrastructure.
- Factors such as the learning curve associated with each tool and the level of support available should also be taken into account during the selection process.
Importance
- Efficient data integration streamlines operations, reduces manual data handling, and provides a more holistic view of organisational data.
4. Ensuring Data Quality and Compliance:
Data Compliance Measures:
- Staying abreast of industry regulations and compliance requirements is crucial for avoiding legal and reputational risks. Organisations should designate individuals or teams responsible for monitoring changes in data regulations.
- Implementing protocols for data anonymization and encryption is a proactive measure to ensure compliance with data protection regulations. This involves applying techniques to protect sensitive information and prevent unauthorised access.
Data Quality Assessment:
- Implementing tools to assess the accuracy and completeness of data involves leveraging data quality assessment tools. These tools analyse data against predefined quality standards, identifying discrepancies and anomalies.
- Establishing benchmarks for acceptable data quality standards sets the bar for data accuracy and completeness. Organisations must define what level of data quality is deemed acceptable for various types of data.
Importance
- High data quality enhances the credibility of your insights and ensures compliance with regulations, safeguarding your organisation from legal and reputational risks.
5. Establishing a Data Lifecycle Management Strategy
Data Archiving and Deletion:
- Establishing criteria for archiving and deleting outdated data involves defining when data becomes obsolete and should be archived or deleted. This process helps optimise storage resources and maintain a streamlined dataset.
- Implementing automated processes for archival and deletion involves leveraging tools and scripts to automate the lifecycle management of data. Automation reduces the manual effort required for data maintenance and ensures consistency in data management practices.
Data Usage and Analysis:
- Ensuring data availability for analysis and reporting involves creating processes that make data accessible to relevant stakeholders. This includes implementing version control for evolving datasets to track changes and updates.
- Implementing version control for evolving datasets ensures that organisations can trace modifications made to datasets over time. This capability is valuable for maintaining data lineage and understanding the evolution of data.
Data Creation and Ingestion:
- Defining processes for data creation and initial storage involves establishing protocols for how data is generated within the organisation. This includes ensuring that data is created in a standardised format and validated upon ingestion.
- Implementing protocols for data validation upon ingestion involves conducting checks to verify the accuracy and completeness of incoming data. This step is crucial for preventing the ingestion of erroneous or incomplete data.
Importance
- A well-defined data lifecycle management strategy optimises storage costs, ensures data availability when needed, and mitigates the risks associated with retaining unnecessary data.
Conclusion
Creating a data management strategy is not a one-time effort but an ongoing process that evolves with the organization’s needs and the data landscape. By following these five key steps, businesses can establish a robust foundation for effective data management, unlocking the full potential of their data assets. As data continues to play a pivotal role in decision-making, a well-crafted data management strategy becomes a competitive advantage in today’s data-driven landscape.