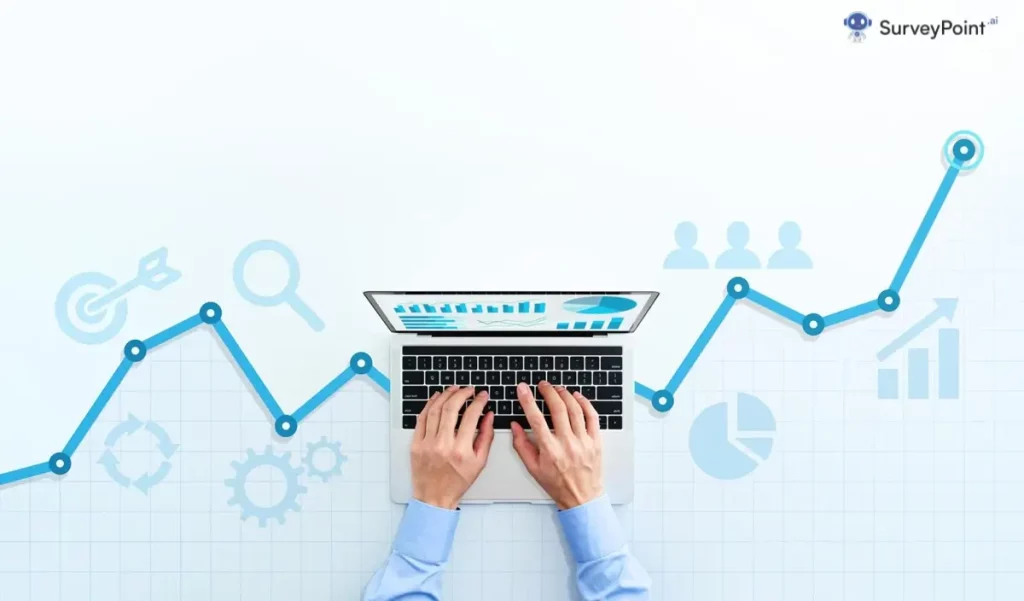
Smart methodology data analysis is a structured approach to analyzing data that helps businesses make informed decisions. It involves using a set of criteria to ensure that objectives are clearly defined and attainable within a certain timeframe. Smart methodology data analysis is a powerful tool that can help businesses improve their performance by providing insights into customer behavior, market trends, and other key metrics.
Smart Methodology Data Analysis
Smart methodology provides a framework to make your data analysis focused and result-oriented. It involves setting Specific goals that clearly state what you want to accomplish from the analysis such as improving conversion rates or reducing customer churn. The goals must be Measurable with quantifiable metrics so you can track progress over time. The analysis should lead to Actionable insights that can drive business decisions rather than just produce reports. The goals and analysis should be Relevant to your core business objectives. Finally, defining a Timeline creates a sense of urgency and ensures the analysis outputs are delivered on time to drive decisions. Using this methodology leads to targeted analysis that provides tangible and timely insights to improve business performance.
Smart Methodology Data Analysis Example
Here is an example of how smart methodology can be applied for data analysis:
Specific Goal: Improve the conversion rate of landing page A from current 2% to 5% in 3 months
Measurable Metric: Actual conversion rate over time
Actionable Insight: Identify drop-off points in the conversion funnel through user analytics to understand pain points and devise fixes
Relevant to: Increasing leads and revenue from key marketing channel
Time-bound: Analysis to be done over next month and fixes to be implemented over 3 months to improve conversion rate from 2% to 5%
As we can see, the SMART framework helped clearly define what the analysis aims to achieve, what metrics to track, how insights will be actioned, relevance to business goals and setting a deadline to accomplish the goal. This focuses the analysis leading to targeted insights.
You Must Know How to Master MCSE Data Management and Analytics Certification: 15 Expert Tips to Excel in Your Career 5 Simple Steps to Run a SQL Query for Data Analysis: A Beginner’s Guide for Data Analysts
Smart Goals for Data Analyst Examples
Here are some examples of smart goals that data analysts can set to ensure their analysis adds maximum business value:
Increase predictive accuracy for churn model from 80% to 85% by end of Q1 to better retain customers
Identify top 3 usage drivers of our app by correlating behaviours to usage patterns by end of month to enhance customer experience
Reduce wait time for weekly sales reports by 2 days in 2 months through automation to enable quicker business decisions
Deliver dashboard visualising key customer acquisition metrics (conversion rates, CAC etc.) in 15 days to align marketing efforts to goals
Analyse impact of running special promotions on festive season sales by end of November to optimize marketing spending
Correlate ratings/reviews by location to identify priority areas for app improvement in 45 days to drive growth
Identify next 3 likely customer segments to target by analyzing our best customers in 60 days to support expansion plans
As we noticed, these examples clearly outline the specific end outcome, measurable targets, timeframe, and relevance which will enable focused analysis to derive insights that align to business priorities.
Smart Methodology, Questions That Encourage Change
The SMART methodology emphasizes asking the right questions to encourage evidence-based change. Some examples of such questions when analyzing data are:
How can we increase sales by 10% in six months based on revenue trend data and growth drivers?
What are the trends in customer complaints over the past year and how can we reduce them by 50%?
What customer segments have the highest churn rates currently and how can we halve churn in 12 months?
How can improving our Net Promoter Score (NPS) by 20 points in 9 months increase customer lifetime value?
What are the demographic and behavioural patterns of our most valuable customer segment we should target more?
Which three product features are driven maximum negative feedback we should improve in four months?
What operational areas have the highest process failure rates we should optimise in 3 quarters to reduce costs by 15%?
Smart Goals for Data Management
Here are some examples of smart goals that data analysts can set for better data management:
Implement processes for continuous data validation to ensure overall 95% data accuracy by Q3
Build automated scripts by Q2 to reduce time taken for sales data reconciliation by over 20% enabling quicker insights
Reduce missing values in customer database from 18% to 5% by Q4 through better data collection processes to drive targeted campaigns
Create metadata documentation for all internal datasets detailing data sources, business meaning & update frequencies by year end for unified understanding
Establish a cloud-based Master Data Management (MDM) system linking disparate systems by Q3 enabling single source of truth for key business entities
Implement data monitoring, usage analytics & controls by halfway Q2 to increase data security, privacy & optimize storage costs
These smart goals will enable better data collection, accuracy, documentation, security and ultimately drive more impactful decisions through precise, complete data leveraging automation and modern data management platforms.
Structured Thinking in Data Analysis
Structured thinking provides the right mental framework when analyzing data to derive meaningful insights rather than getting overwhelmed by details. Key aspects include:
Framing the Right Questions: Structured thinking starts by asking questions like who will use the insights, how will it impact decisions, what hypotheses need validation about markets, customer behaviour etc. This grounds the analysis to delivering actionable insights.
Organizing Datasets: Think what historical datasets are needed, how they need to be processed, correlated together into structures like tables, matrices to identify patterns. This enables methodical analysis.
Evaluating Relationships: Evaluate causal relationships in the structured data like how usage drives revenues, one behavior affects another, product issues affect ratings etc. These core drivers enable predictive analysis.
Synthesizing Insights: Structure the insights so they are connected to business outcomes intended, easy for decisions makers to understand and include clear recommendations. This drives change through data.
FAQs: Addressing Common Queries
How does smart methodology differ from traditional data analysis?
Smart methodology incorporates advanced technologies like AI and machine learning, enabling more accurate predictions and proactive decision-making compared to traditional methods.
Can small businesses benefit from data analysis?
Absolutely! In fact, data analysis can be a game-changer for small businesses, helping them identify growth opportunities, understand customer needs, and optimize operations.
How do I ensure the security of sensitive data in the analysis process?
Implement robust encryption methods, access controls, and regularly update security protocols to safeguard sensitive data throughout the analysis process.
Is data analysis a one-time effort, or should it be ongoing?
Data analysis should be an ongoing effort. Regularly analyze new data to adapt to changing market conditions and ensure your strategies remain relevant and effective.
Can data analysis improve employee productivity?
Yes, by identifying inefficiencies, streamlining processes, and providing insights for better decision-making, data analysis can contribute to increased employee productivity.
How can businesses without a dedicated IT department implement data analysis?
Consider outsourcing or investing in user-friendly analytics tools that require minimal technical expertise, making data analysis accessible to businesses of all sizes.
Conclusion
In conclusion, adopting the SMART methodology can hugely enhance the quality of business data analysis by making it specific, measurable, actionable, relevant and timely. This focuses the analytical effort towards business priorities and ensures better tracking and ROI. Setting SMART data analysis goals creates structured thinking for deriving insights by correlating the right datasets and evaluating relationships to address the right questions. This ultimately leads to higher business performance through data-driven, evidence-based decisions on priorities like growth, costs, customer retention. As data becomes increasingly critical, companies that master leveraging analytics through methodologies like SMART will gain sustained competitive advantage.