Statistical tests allow analysts to derive powerful insights from data. However, choosing and applying the right statistical tests can be challenging.
This guide provides a structured step-by-step framework to help you master statistical testing. Follow these steps to conduct seamless tests and unlock deeper data insights.
Step 1: Determine Your Analysis Goal
First, clearly define the objective of your analysis such as:
– Comparing group means
– Assessing correlation between variables
– Predicting outcomes through modeling
– Testing differences between periods
The goal guides which statistical test will be most appropriate.
Step 2: Check Assumptions
Before applying a test, verify your data meets the required assumptions:
– Sample size – Is there enough data for statistically valid results?
– Data distribution – Is the data normal, skewed, categorical, ordinal?
– Variable type – Are the variables continuous, discrete, dichotomous?
– Random sampling – Is the data randomly sampled or biased?
– Independence – Are data points independent or related?
Failing to meet assumptions may require transforming data or opting for a different test.
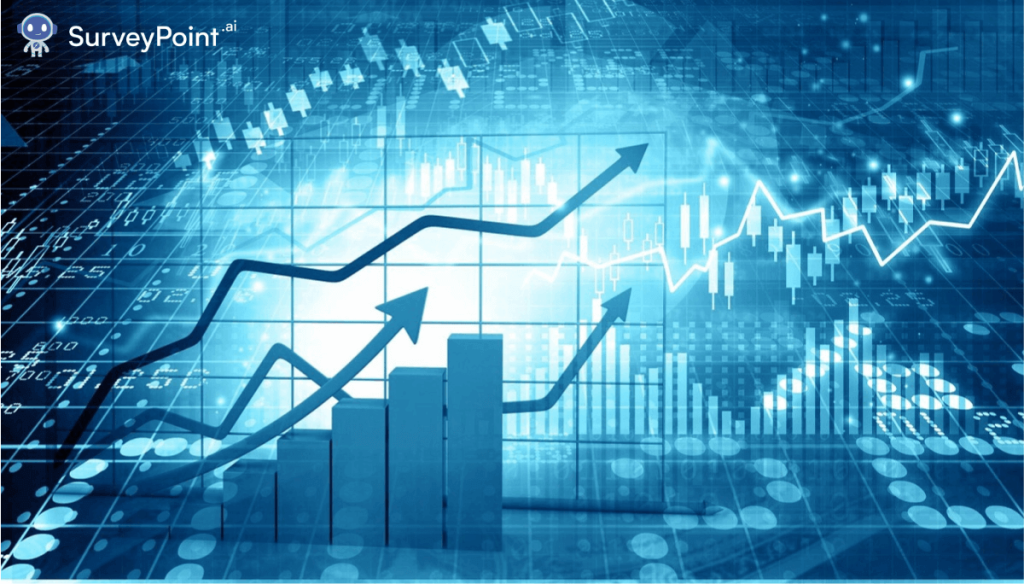
Step 3: Select the Right Statistical Test
With your goal and data characteristics in mind, pick the appropriate test:
– T-test – Compare means of two groups or populations
– ANOVA – Compare means of three or more groups
– Chi-square – Test independence between categorical variables
– Regression – Model and predict continuous response variables
– Non-parametric tests – For data that violates assumptions
There are dozens more tests for specialized analysis needs.
Step 4: Determine Parameters
Set parameters like confidence level, significance threshold, and power based on standards for your domain. Typical parameters are:
– Confidence level: 95%
– Significance: 0.05 alpha level
– Power: 80%
These determine the standards used to assess statistical significance.
Step 5: Run the Test
Use statistical software like R, SAS, SPSS, or analysis packages in Python/RStudio to run the selected test on your dataset.
Follow the proper syntax and inputs for the test in your chosen tool.
You Must Read How to Choose the Perfect Statistical Tests for Your Data Analysis Success Data Visualization: Meaning, Tools and Techniques
Step 6: Analyze Results
The test outputs key metrics to interpret:
– P-value – Assess against your significance threshold
– Effect size – Magnitude of differences or relationships
– Statistical power – Validity of conclusions based on sample size
Determine if results are statistically significant or if the null hypothesis stands.
Step 7: Document Findings
Summarize your test approach, parameters, results, and conclusions in a report. Visualizations and tables help convey key data points.
Documentation helps justify findings and allows revisiting work later.
Step 8: Take Action
Only act on statistically validated, significant results rather than taking direction from noise in data.
Use findings to guide business decisions, optimize processes, allocate resources efficiently and more.
Key Takeaways
Applying this structured 8-step statistical test process allows deriving meaningful, credible insights from your data.
It equips you to:
– Choose the right tests aligned to your goals
– Verify test assumptions are met
– Analyze and interpret test results accurately
– Take data-driven actions based on statistically significant findings
With rigorous use of statistical testing, you can elevate your data science skills to make smarter business decisions powered by data.