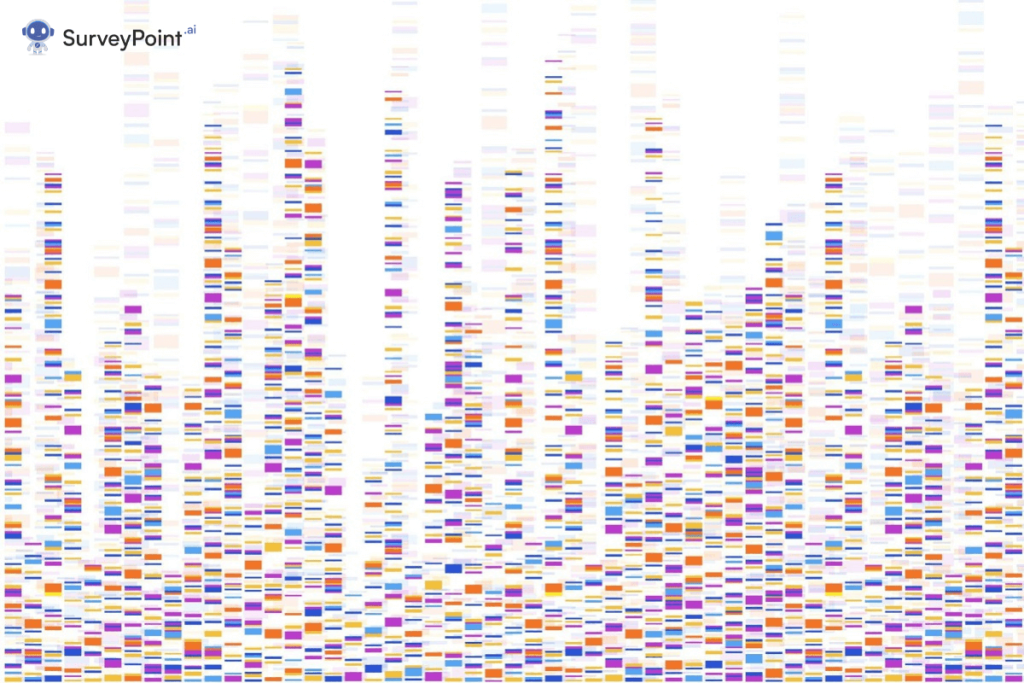
Statistical tests are essential tools in the data analyst’s toolkit. They allow you to draw meaningful conclusions from datasets and quantify relationships between variables.
In this guide, we’ll explore some of the most commonly used statistical tests and their powerful applications in data analysis. Unlock the secrets to data analysis success with our comprehensive guide! Learn how to expertly select the ideal statistical tests for your research, ensuring accurate and meaningful results every time.
Overview of Statistical Testing
Statistical tests check if patterns in data reflect a real effect or are merely due to chance. By determining statistical significance, these tests help answer questions like:
– Is there a relationship between variables in my data?
– Did this process or treatment drive a real change in the metric I am analyzing?
– Are there statistically significant differences between groups in my sample?
Statistically significant results give you confidence that the effect is real and not just random noise in data.
Common Types of Statistical Tests
Some widely used categories of statistical tests include:
Hypothesis Tests – Assess assumptions about data by checking the likelihood of results under a null hypothesis. Common examples are t-tests, ANOVA, and chi-square tests.
Correlation Tests – Measure the strength of association between two variables. Pearson’s and Spearman’s tests are commonly used for quantitative and ordinal data respectively.
Regression Analysis – Quantifies relationships between independent and dependent variables. Simple linear regression is the most common approach.
Non-Parametric Tests – Used when data does not meet assumptions of parametric tests. Examples are the Wilcoxon signed-rank test and Kruskal-Wallis H-test.
Key Benefits of Statistical Testing
Applying statistical tests offers several advantages:
– Validates relationships and differences – Tests tell you if connections in data are statistically significant and not mere coincidences.
– Provides mathematical rigor – Statistical significance gives you an objective, quantified assessment of results.
– Allows drawing inferences – Significance testing enables making conclusions that can be extended to wider populations.
– Helps determine predictive models – Identifying significant relationships aids in developing predictive analytics models.
– Compares results over time – Determine if changes between testing periods are statistically significant.
– Guides business decisions – Significance testing enables data-driven decision making and strategy.
You Must Like: Understanding Method vs. Methodology: A Comprehensive Guide
Examples of Statistical Tests in Action
Some examples of using statistical tests for key analyses:
– Run an ANOVA test to compare average sales across different regional markets and determine if differences are statistically significant.
– Use a chi-square test to assess if prospect-to-customer conversion rates differ significantly before and after a website redesign.
– Apply regression analysis to identify key marketing channels that most influence revenue. Develop predictive models based on significant drivers.
– Leverage A/B testing to determine if introducing a new product feature creates a statistically significant lift in customer engagement.
Tips for Applying Statistical Tests
When using statistical tests, keep these tips in mind:
– Check assumptions of each test about data distribution, sample size, etc.
– Avoid overusing tests – apply only where scientifically relevant.
– Correct for multiple simultaneous testing to avoid false positives.
– Use tests suitable for your data types like ordinal, time series, or categorical data.
– Consider probability and confidence thresholds when determining significance.
– Test one variable relationship at a time.
– Revalidate models and relationships over time as new data comes in.
You Must Read Data Visualization: Meaning, Tools and Techniques Understanding Method vs. Methodology: A Comprehensive Guide
Key Takeaways
Statistical testing is invaluable for extracting meaningful insights from data. Matching proper test types to your objectives, variables, and data allows mathematically quantifying relationships to derive actionable decisions confidently.
With a strong grasp of statistical testing, you can elevate your data analysis and business thinking to new levels of rigor, precision, and impact.