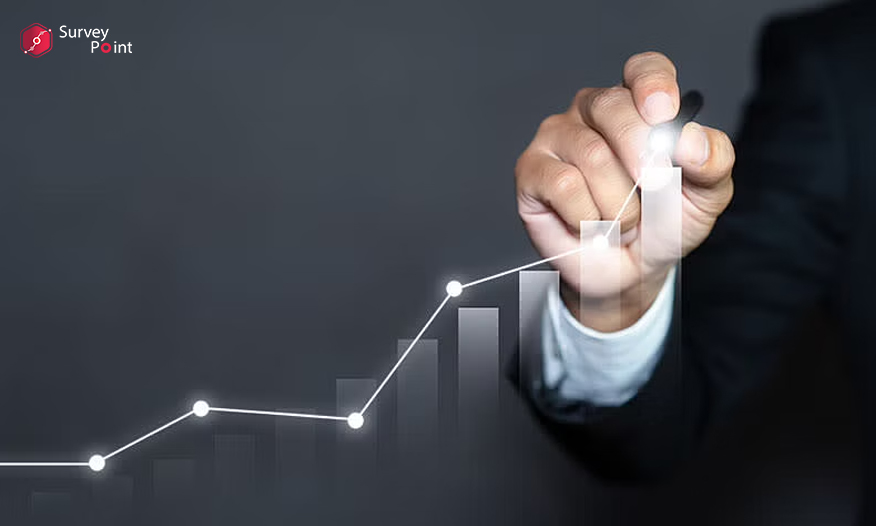
Descriptive statistics, as the name implies, describe sample characteristics. The selection of the appropriate type of data analysis for research is a critical part of analyzing surveys.
In order to analyze data, first and foremost, statistics need to be used to describe the data.
The Importance of Descriptive Statistics
Descriptive statistics allow us to show the data more meaningfully, making it easier to understand. It helps make sense of data and provides insights about data that can be further validated using inferential statistics and advanced analytics.
Let’s talk about a few of the most common descriptive statistics used in quantitative research.
Frequency And Percentage
Frequency and percentage tables are an excellent place to start. It shows counts and percentages for each unique value in a variable, usually a categorical variable. A frequency is the number of times each score appears in each data set, while a percentage represents a fraction of 100.
One usually starts with tables describing gender, religion, and ethnicity. The best way to describe these tables is by making a table of frequency and percentages. The frequency and percentage tables should be aligned with the research objective when working on them.
Further, one can also do sub-group analysis using other variables as part of cross-tabulation. As a next step, we should determine the central tendency.
A Measure of Central Tendency
The next stage is to look for a measure of central tendency. A central tendency is a single number describing a data set by figuring out where the middle point is and is also known as summary statistics. Even though there are several ways to measure central tendencies, researchers commonly use the arithmetic mean and the median.
The arithmetic mean is the first way to measure central tendency. It is calculated by dividing the number in the series by the total number. The arithmetic mean is sometimes called the average or just the mean in the series. Before using the arithmetic mean, one must meet several assumptions, i.e., at least one interval scale is used to measure the variable. The data should be spread out typically, and there shouldn’t be any significant outliers.
A researcher can assess the first assumption using the question type, while the second assumption can be evaluated using a Normal Q-Q plot histogram or other statistical tools.
Box plots can be used to check the third assumption. One should not use the arithmetic mean for nominal or ordinal values because it doesn’t make sense to add up the data for these variables.
Also, the arithmetic mean is very sensitive to outliers. In the case of at least one outlier, the arithmetic mean does not accurately represent the whole data set.
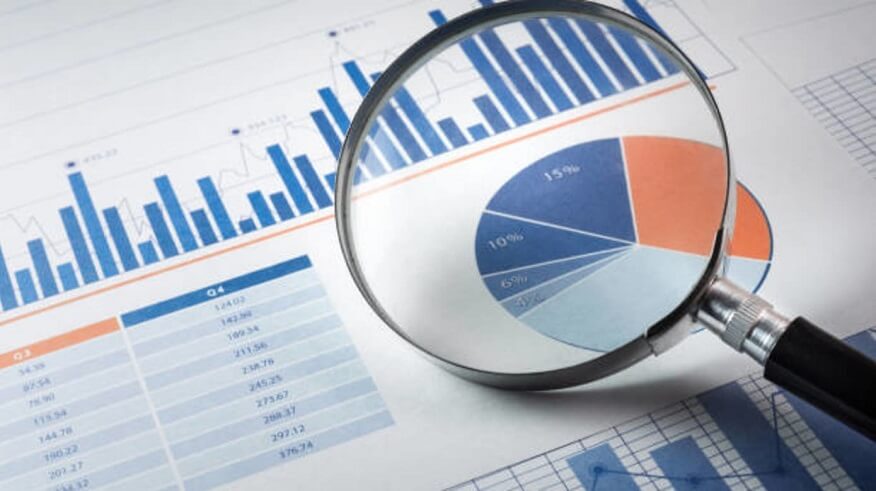
Median
This is the middle score of a data set sorted by size. Outliers and skewed data don’t have as much of an effect on the median.
Before using the median, you only need to ensure that the variable is at least ordinal. Median should be used in the case of non-normally distributed data or where there are a lot of outliers.
A mode is defined as the most frequent value in a dataset. Although it isn’t used as often in primary research, it plays a significant role in production and manufacturing decisions.
Variability
Another critical measure while using descriptive statistics is to assess variability. A measure of variability is also known as dispersion or spread. It describes how different things are in a group or sample. In general, it is used to describe a group of numbers in combination with a measure of central tendencies like the mean or median.
Data variability is a measure of how well the mean represents the data. For instance, in a large data set, the mean tells us less about the data than it would if there were a small range of data. A large spread suggests that individual scores have significant differences. Big spreads are often considered good in research. If there isn’t much difference between each data group, the respondents are similar.
Minimum, Maximum, Range, and Quartiles
The data value distribution can be determined using minimums, maximums, ranges, and quartiles. The distribution range is calculated by subtracting the lowest and highest values. Further, the quartiles represent one-fourth and three-quarters of the way through a range of values.
Spread and interquartile range are fundamental measures of the spread of data distribution. They indicate how big the gap is between the top and bottom quartiles of the data distribution.
Standard Deviation and Variance
The standard deviation is the initial method for measuring dispersion. In relation to the mean, the standard deviation measures what degree of dispersion the data exhibit. To obtain it, one takes the square root of the standard deviation.
The standard deviation is used using the arithmetic mean to determine the central tendency. Increasing the distance between the data points and the mean will increase the standard deviation.
Alternative methods for measuring dissimilarity include the interquartile range. As a general rule, the interquartile range represents the area between the third and first quartiles. It indicates the distance between the center-half of the scores.
There is a common belief that the interquartile range and the median are the best ways to measure the spread and central tendency, respectively. A standard deviation is a valuable tool when dealing with skewed or outliers data. If the interquartile range is high, then the variation is also high.
An alternative method of measuring variability is variance. It is a way to measure how far an estimate is from the hypothetical mean that could come from doing multiple surveys.
Which Measure to Use
In short, one must ascertain what statistical tool to use to describe your data set. One can use a frequency and percentage table if you have a nominal variable.
Further, one can use an ordinal frequency and percentage table if you have an ordinal variable. A median and interquartile range can be used when data are not normally distributed, have outliers, or both. Researchers can use the mean and standard deviation in the case of normally distributed intervals and ratios.
Kultar Singh – Chief Executive Officer, Sambodhi