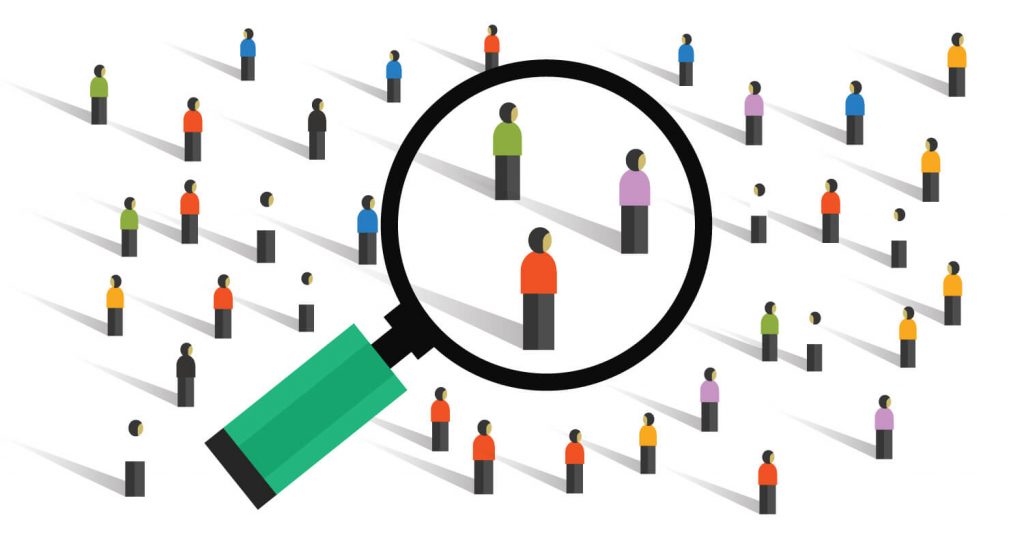
Sampling aims to measure any value related to people as accurately as possible. But, even if we use the most advanced sampling methods, there will always be some difference between our sample value estimate and the actual value in the population. The difference between a sample’s value and the whole population’s value is usually called an error. This mistake can be put into two main groups: sampling error and non-sampling error. In this note, we will discuss sampling errors.
It is essential to point out that the actual value of the population can hardly be known. If we knew the true value of the people, we wouldn’t need a sample. This also means we can’t tell how big an error is for any given example. However, one can figure out the sampling error.
Let’s learn more about sampling error and how it works. For instance, we could take an infinite number of samples from a population. Then, under specific conditions, the average of all these samples will be the same as the population value. Still, each sample will have a different sample value than the population value. Sampling error hence can be defined as the difference between the value of a population sample and what we would expect that value to be. We can figure out how big the sampling error is on average if we keep extracting random samples from the population.
Based on the values in a single sample, we can estimate the average, called the standard error. We can then use the standard error to determine the margin in the error. One might think that the margin of error tells us how much our sample differs from the population at most. But, we can’t figure out exactly where the true population value lies. This is because we’re estimating the sampling error in the long run based on repetitive samples. In the long run, it’s always possible for a value to be significantly small or large. We can say that the value of the population will be between specific limits. This information is usually shown in a confidence interval. With a confidence interval, we can say that if we continuously keep taking samples, the true population value won’t be more than the margin of error away from the sample value in a specific percentage of those samples.
Sampling error is linked to sample size. As the sample size goes up, so does the sampling error. The amount of variation in the population can also change sampling error. If a population’s property of interest differs significantly, then a given sample size can lead to very different sample values. When there is more variation in a population, the sampling error will be more precise.
Sources of Sampling Error
Two leading causes of sampling errors are:
Chance- this means that specific uncommon/variant units are randomly picked, as the selection is always possible. This mistake can be avoided by increasing the sample size to reduce the chance of selecting unusual or variant units.
Tendency to favor units with particular features- Selection bias is the most obvious form of sampling bias. This happens when specific units are unlikely to be included in the sample.
Steps to Reduce Sample Errors
It is easy to identify sampling errors. These are some simple steps that can be taken to reduce sampling errors:
- One way to reduce sample error is to increase the sample size. A larger sample size correlates to a more accurate result, as the study is closer to the actual population.
- One can also use a random sample to test groups based on population size.
- Further, to reduce sampling bias, and ensure that you have a study with this demographic. One can learn about your population. You can identify the demographics that use your product or service to target only those most relevant.
Kultar Singh – Chief Executive Officer, Sambodhi