Businesses use data science to learn about consumer and market behavior. Regardless of the fact that it has numerous other applications,
In higher education, you can specialize in a wide range of areas, from data systems administration and security to machine learning and artificial intelligence. A master’s in data science can prepare you for a wide range of technology-related occupations, including data engineering, data architecture, and computer programming.
But if you are still unsure as to whether or not data science is for you, this comprehensive guide will provide you with all the information you need to make an informed decision.
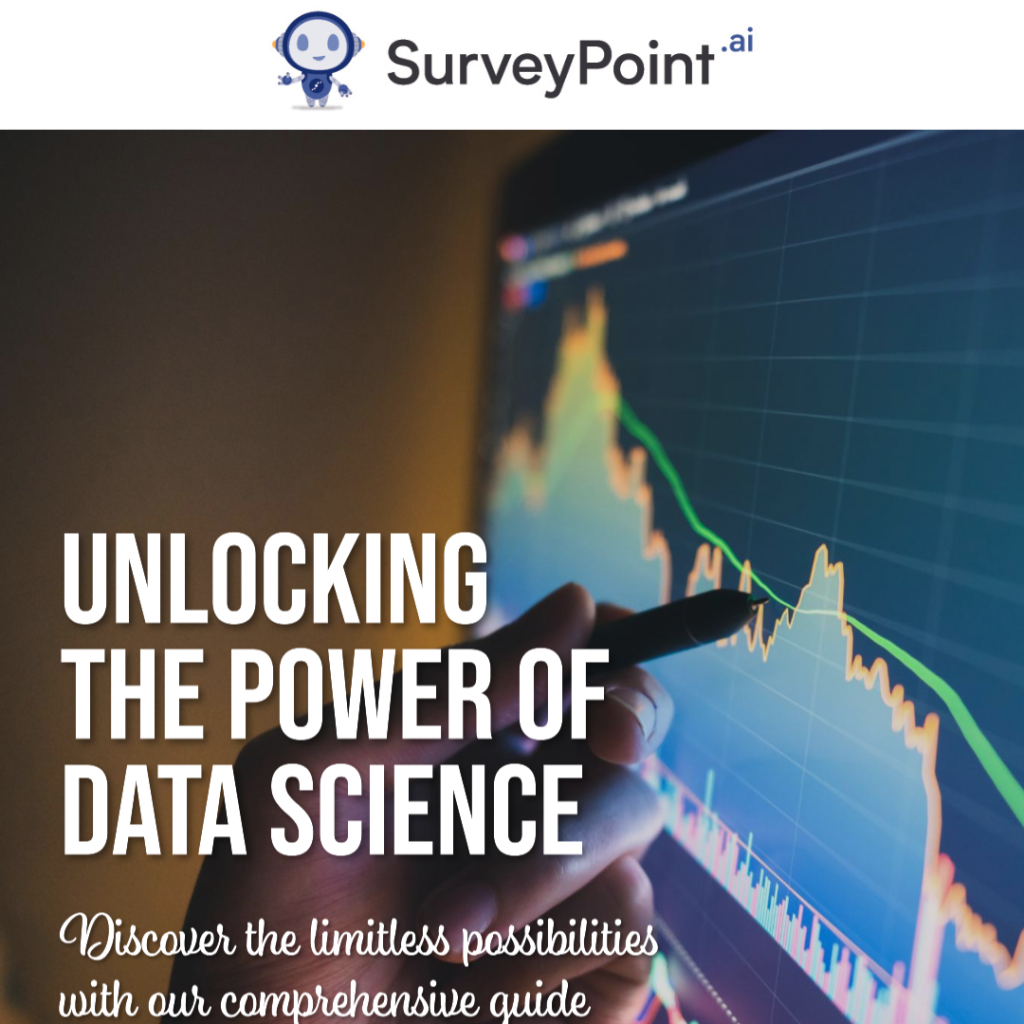
Let’s begin with the basics of data science, including what it is, why it’s useful, and what forms of data science there are.
What exactly is data science?
Obtaining useful information for a company’s operations is the focus of data science. It’s a method for analyzing massive datasets that borrows ideas from several disciplines, including math, statistics, AI, and computational science.
What happened, why it happened, what will happen, and what can be done with the results are all questions this analysis helps data scientists answer.
What are the positive aspects of studying data science?
Data science is important because it combines different approaches, techniques, and technologies to extract insights from data. Businesses today have to sort through a lot of data because there are so many devices that can automatically collect and store information. In e-commerce, medicine, and finance, online systems and payment portals collect more information about consumers, patients, and customers. We have access to a huge amount of information in the form of text, audio, video, and pictures.
Definition of Big Data Analytics
Big data analytics aims to help businesses make more informed decisions by uncovering actionable insights, trends, and patterns in massive amounts of data. This data can be accessed rapidly and effectively, allowing businesses to respond quickly to market changes and adjust their strategies.
Companies can use their structured and unstructured data better thanks to technologies like business intelligence (BI) tools and systems. To gain insight into the workings and performance of a company, users (typically employees) input queries into these tools.
The four data analysis techniques are used in big data analytics to find helpful information and arrive at workable conclusions.
Data science in statistics: what is it?
Statistics and data science are at the heart of today’s data analytics, which use this intelligence to guide decision-making and address complex issues. Economics, medicine, the social sciences, and even ecology can all benefit from this approach.
What are the various data science tools available?
Professionals in the field of data science use sophisticated tools like:
AI uses machine learning models and associated software for both predictive and prescriptive analysis.
- Computing in the cloud: This technological advancement has provided data scientists with the processing power and adaptability they need for more complex data analytics.
- The term “Internet of Things” (IoT) describes a wide range of connected devices that can connect to the web without human intervention. Data science efforts make use of the information that these devices collect. They produce reams of valuable information for data extraction and mining.
- Computing on the quantum level: complex calculations can be carried out quickly. Competent data scientists use them in the creation of complex quantitative algorithms.
Data science applications
- Data science has the potential to find connections and patterns in seemingly unrelated data, allowing for inferences and forecasts to be made.
- Tech companies that collect user information can use data analytics and other methods to mine this data for insights.
- Advances in data science have also benefited the transportation industry with applications such as driverless cars. A simple solution for reducing traffic accidents is to use driverless cars. In the case of autonomous vehicles, for instance, data Science methods are used to analyze training data, such as the speed limit on the highway, busy streets, etc.
- Data science applications enable better therapeutic individualization based on genetic and genomic analysis.
What distinguishes data science from data analytics?
Data analytics is a branch of data science, although the two terms are often used interchangeably. From data collection and modeling to analysis and insights, data science encompasses it all. In contrast, the main focus of data analytics is mathematical and statistical analysis. While data science concerns the bigger picture of an organization’s data, data analysis focuses on the details.
Data analysts and data scientists typically collaborate to achieve organizational objectives. Data analysts can devote most of their time to routine analysis and report writing.
For example, A data scientist might plan the infrastructure for collecting, processing, and analyzing information.
A data scientist’s role is to create new methods of processing data that analysts can use, whereas a data analyst’s job is to make sense of existing data.
Relationship between AI and Data Science
Now that you are familiar with Data Science and AI, we can discuss the interplay between these two disciplines and how Data Engineering fits into the picture.
There is a strong connection between Data Science and AI. Data Extraction, Transformation, and Storage (ETS) is the first step in Data Engineering. To be effective, AI in Data Science applications requires that the raw data be Engineered first.
Python’s Usefulness in the Field of Data Science
Python’s accessibility, vibrant community, robust data analysis and visualization libraries, and excellent machine-learning libraries have made it a go-to language for data scientists.
In terms of domains of use, the following Python modules are most popular among data scientists:
- Analyzing the Data
- Charts and graphs
- Inferencing Machines
- In-Depth Learning
- Processing images
- Electronic Eyes
- NLP stands for “natural language processing.”
Summary Information will continue to be crucial to businesses’ future success. Data is the knowledge that can be put into action, and that knowledge can be the difference between success and failure for a business. Companies can plan for the future, anticipate challenges, and make educated decisions by implementing data science techniques.