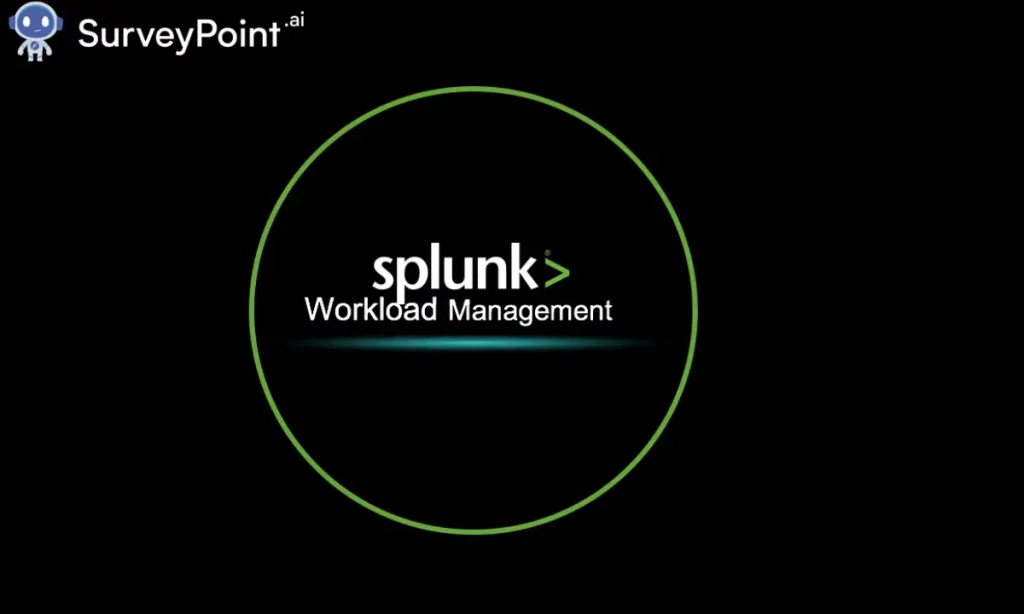
In the ever-evolving landscape of data analytics, Splunk stands out as a powerhouse for handling massive volumes of data. This comprehensive guide explores the best practices in Splunk Workload Management, delving into the intricacies of efficient data handling and the importance of comprehensive training. Additionally, we’ll unravel the concept of Splunk Workload Pools, providing insights into how they contribute to optimal performance.
Understanding Splunk Workload Management: Navigating the Data Deluge
1. Defining Splunk Workload Management Splunk Workload Management (WLM) is a strategic approach designed to optimize the performance and efficiency of data processing within the Splunk platform. It involves thoughtful resource allocation, prioritizing critical tasks, and ensuring seamless data ingestion and analysis.
2. The Significance of Workload Management in Splunk As organizations deal with escalating data volumes, effective Workload Management becomes crucial. It ensures that critical data processes are prioritized, preventing resource bottlenecks and optimizing the overall performance of the Splunk environment.
Splunk Workload Management Best Practices: Strategies for Optimal Efficiency
1. Prioritize Critical Workloads Identify and prioritize critical workloads based on business requirements. Allocate resources accordingly to ensure that essential tasks receive the necessary processing power and storage.
2. Implement Resource Quotas Establish resource quotas for different workloads to prevent any single process from monopolizing system resources. This helps maintain a fair distribution of resources and prevents performance degradation.
3. Leverage Indexer Clustering Utilize indexer clustering to distribute data processing across multiple nodes. This not only enhances scalability but also contributes to efficient workload distribution.
4. Regularly Monitor and Adjust Implement a robust monitoring system to keep a close eye on system performance. Regularly analyze the data to identify potential bottlenecks or areas for improvement, and adjust workload management strategies accordingly.
Splunk Training: Empowering Your Team for Success
1. The Role of Training in Workload Management Investing in Splunk Workload Management training is essential for ensuring that your team understands the intricacies of the platform and can implement best practices effectively. Training empowers teams to make informed decisions and navigate complex data scenarios.
2. Key Training Components
- Understanding Splunk Architecture: Trainees should have a solid understanding of Splunk architecture, including the roles of indexers, search heads, and forwarders.
- Resource Allocation: Training should cover the principles of resource allocation and how to effectively manage resources for optimal performance.
- Query Optimization: Equip team members with the skills to optimize queries, ensuring efficient data retrieval and analysis.
- Troubleshooting Techniques: Provide training on troubleshooting common issues related to workload management, enabling teams to address challenges swiftly.
3. Continuous Learning and Updates Given the dynamic nature of technology, continuous learning is key. Regularly update training programs to incorporate the latest features and best practices in Splunk Workload Management.
Unveiling Splunk Workload Pools: A Strategic Approach to Resource Allocation
1. Defining Workload Pools Splunk Workload Pools enable the classification and allocation of resources based on the specific needs of different workloads. By grouping similar workloads together, administrators can efficiently manage and allocate resources to meet performance objectives.
2. Benefits of Workload Pools
- Resource Isolation: Workload Pools provide a level of resource isolation, preventing one workload from negatively impacting others.
- Improved Performance: By tailoring resource allocations to the specific needs of workloads, overall system performance is optimized.
- Enhanced Scalability: Workload Pools contribute to improved scalability, allowing organizations to handle growing data volumes effectively.
Conclusion: Orchestrating Success with Splunk Workload Management
As we navigate the complexities of data analytics, Splunk Workload Management emerges as a beacon of efficiency. By embracing best practices, investing in comprehensive training, and strategically utilizing workload pools, organizations can orchestrate success in their data processing endeavors.
In the evolving landscape of data analytics, where efficiency is paramount, mastering Splunk Workload Management is not just a choice—it’s a strategic imperative. As you embark on this journey, remember that the key to success lies in a holistic approach that combines best practices, training, and innovative strategies to unlock the full potential of Splunk for your organization’s data analytics needs.