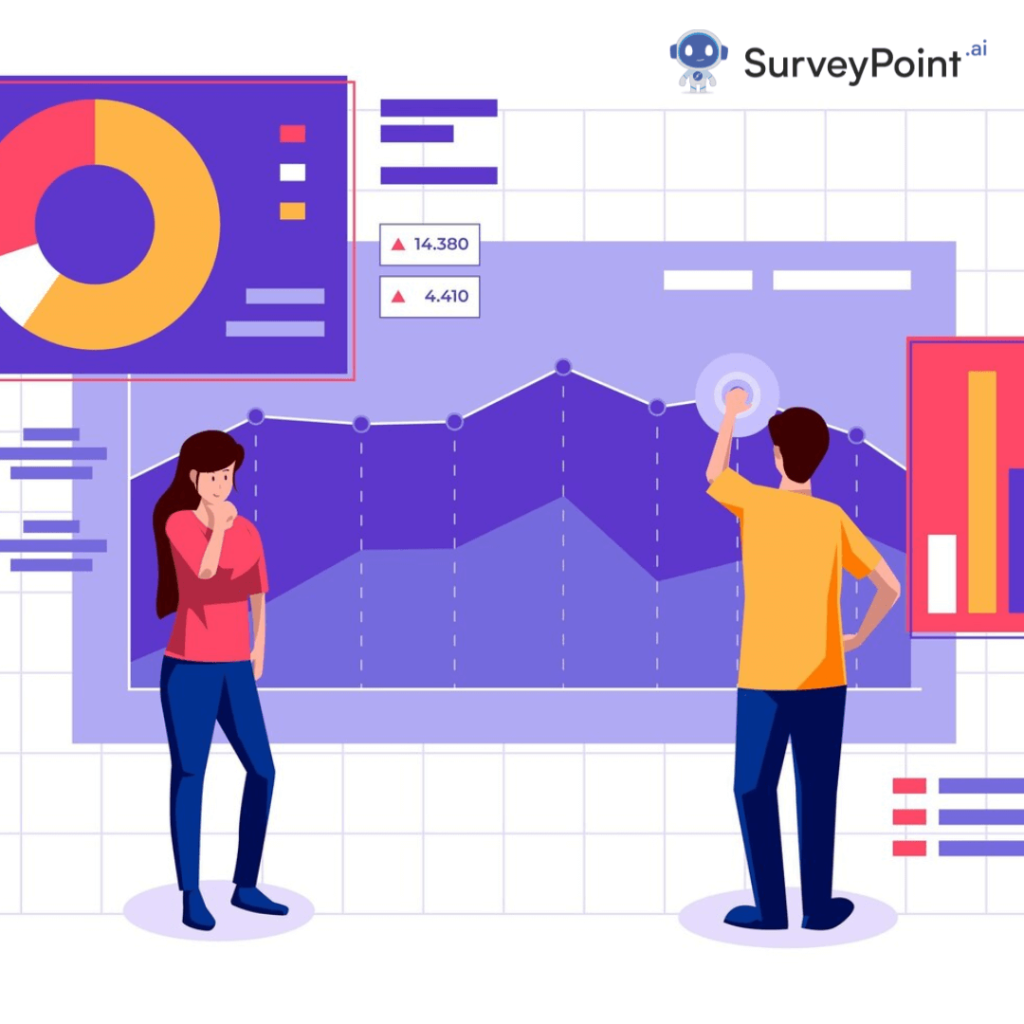
Quantitative data analysis is a cornerstone of research, business intelligence, and decision-making across various fields. Whether in the social sciences, healthcare, marketing, or economics, understanding and interpreting quantitative data helps researchers, analysts, and professionals extract valuable insights, test hypotheses, and predict future trends. In this comprehensive guide, we will explore the fundamental aspects of analyzing quantitative data, from data collection to visualization, statistical methods, and interpretation.
What is Quantitative Data?
Quantitative data refers to data that can be measured and expressed in numerical terms. This type of data involves quantities or amounts that can be counted, measured, or identified using numbers. It is essential for analysis because it provides a solid foundation for making objective, reliable conclusions. Quantitative data is often categorized into two main types: discrete data and continuous data.
- Discrete Data refers to data that can only take specific, distinct values, often whole numbers. Examples include the number of students in a class, the number of products sold, or the count of events that occur within a specific time frame.
- Continuous Data refers to data that can take any value within a given range. This type of data is measured with great precision and can be represented on a scale. Examples include weight, height, time, and temperature.
Understanding the Importance of Quantitative Data Analysis
Quantitative data analysis allows researchers and decision-makers to objectively interpret numerical data, identify trends, test theories, and make informed predictions. By applying statistical methods, quantitative analysis helps transform raw numbers into actionable insights. For example, in business, companies use quantitative data analysis to understand customer behavior, optimize marketing strategies, and forecast sales trends. In healthcare, quantitative data analysis helps identify treatment efficacy, patient outcomes, and patterns of disease spread.
The Process of Quantitative Data Analysis
The process of analyzing quantitative data is a systematic and structured approach that helps researchers and analysts gain meaningful insights. Whether you are conducting an academic research project, business analysis, or any other data-driven task, following a structured approach ensures that your results are reliable, accurate, and actionable.
Define Your Research Question
The first step in any quantitative analysis is to clearly define your research question or hypothesis. What is the purpose of your analysis? Are you testing a specific theory, comparing two or more variables, or making predictions based on historical data? A well-defined research question guides the entire data analysis process, helping you choose appropriate methods, select the right data sources, and focus on the key elements that will provide useful insights.
Data Collection
The next step is to gather the data necessary for analysis. Data collection is critical to the validity and reliability of your results. The accuracy of your conclusions depends on the quality of the data collected, so it is essential to use rigorous methods to ensure that your data is relevant, reliable, and valid.
Quantitative data can be collected through a variety of methods:
- Surveys and Questionnaires: These are common tools for collecting quantitative data, especially in social research. Researchers design structured questionnaires with closed-ended questions that respondents answer using numerical scales or fixed responses.
- Experiments: In experimental research, quantitative data is often collected as part of an experiment to test hypotheses. This can involve controlled conditions where researchers manipulate variables and observe the outcomes.
- Observational Studies: Data can also be gathered through systematic observations. For example, in a field study, researchers might count occurrences of a particular behavior or event.
- Existing Databases: Sometimes, quantitative data is not collected directly but obtained from secondary sources, such as government reports, industry databases, or previous research studies.
Data Cleaning and Preparation
Once data has been collected, it must be cleaned and prepared for analysis. Data cleaning involves removing or correcting errors in the dataset, such as:
- Missing data: Missing data points can occur due to errors in data collection or respondents skipping questions. It is essential to handle missing data appropriately to avoid biasing your results.
- Outliers: Outliers are data points that fall far outside the expected range. These can be legitimate values or errors. Outlier detection and treatment are crucial, as they can significantly skew analysis results.
- Data normalization: In some cases, data may need to be transformed or normalized to make it suitable for analysis, especially when comparing data across different scales.
Data preparation also involves organizing the data into a format that can be easily analyzed. This might mean creating tables, categorizing variables, or converting raw data into summary statistics.
Exploratory Data Analysis (EDA)
Before diving into complex statistical analyses, it’s useful to conduct Exploratory Data Analysis (EDA). EDA involves using statistical graphics, plots, and other visualization techniques to understand the structure of the data and identify patterns, trends, and potential relationships between variables. Some common tools in EDA include:
- Histograms: To visualize the distribution of a single variable and identify its shape, central tendency, and spread.
- Boxplots: To show the distribution of data and highlight potential outliers.
- Scatter Plots: To visualize relationships between two continuous variables.
- Correlation Matrices: To identify correlations between multiple variables and determine potential associations.
EDA helps you gain a preliminary understanding of the data, which can guide your decisions regarding the appropriate statistical methods to use.
Statistical Analysis
Once you have a solid understanding of your data, the next step is to apply statistical analysis techniques to draw conclusions. The choice of statistical methods depends on the research question, the nature of the data, and the relationships you wish to investigate. Some common statistical analyses used in quantitative data include:
- Descriptive Statistics: Descriptive statistics summarize and describe the main features of a dataset. They provide a concise overview of the data, including measures such as the mean (average), median (middle value), mode (most frequent value), range, variance, and standard deviation. These statistics help to summarize the data and understand its central tendencies and spread.
- Inferential Statistics: Inferential statistics are used to make inferences or generalizations about a population based on a sample. Techniques like hypothesis testing, confidence intervals, and regression analysis are employed to draw conclusions beyond the immediate dataset.
- Hypothesis Testing: This involves testing a null hypothesis (H0) against an alternative hypothesis (H1) using statistical tests such as t-tests, chi-square tests, or ANOVA (Analysis of Variance). The goal is to determine whether there is enough evidence to reject the null hypothesis in favor of the alternative hypothesis.
- Regression Analysis: Regression analysis explores the relationship between one or more independent variables and a dependent variable. Linear regression, logistic regression, and other advanced regression techniques help identify patterns, predict outcomes, and understand the impact of variables.
- ANOVA (Analysis of Variance): ANOVA tests whether there are statistically significant differences between the means of three or more groups. It is used when comparing multiple categories or treatments.
- Chi-Square Test: This test is commonly used for categorical data to assess whether there is a significant association between two categorical variables.
- Multivariate Analysis: In cases where you are dealing with multiple variables, multivariate analysis techniques like Factor Analysis, Principal Component Analysis (PCA), and Multivariate Regression can help identify complex relationships and reduce dimensionality.
Data Visualization
Data visualization is an essential part of quantitative data analysis because it enables researchers to communicate findings clearly and effectively. Visualizations can help uncover patterns, trends, and insights that might not be immediately apparent in raw data.
Common data visualization techniques include:
- Bar Charts and Histograms: Used to display the frequency distribution of discrete or continuous data.
- Line Graphs: Useful for showing trends over time, especially for time series data.
- Scatter Plots: Display relationships between two continuous variables and help identify correlations.
- Heatmaps: Used to visualize the intensity of variables, often used in multivariate data to show correlations or clustering.
- Pie Charts: While often criticized, pie charts can still be used to display parts of a whole when there are only a few categories.
Effective visualizations allow for clearer communication of complex data, enabling stakeholders to grasp insights quickly and make data-driven decisions.
Interpretation of Results
The final step in analyzing quantitative data is interpreting the results. This involves:
- Contextualizing the Findings: The findings of your analysis should be understood in the context of your research question, hypothesis, or business objectives. Were the results consistent with your expectations? Do the findings suggest a new direction for future research or inquiry?
- Assessing Statistical Significance: Statistical significance tells you whether the observed results are likely due to chance or represent a real effect. Typically, a p-value of less than 0.05 indicates statistical significance, but this threshold can vary depending on the field of study and specific research design.
- Drawing Conclusions: After evaluating the statistical significance, you can draw conclusions based on the results. Are your hypotheses supported by the data? What are the practical implications of the findings? How can these insights be applied in real-world scenarios?
Communicating Results
Once the analysis is complete, it is crucial to present the findings in a clear and concise manner. This may involve writing research papers, presenting reports, or creating dashboards for stakeholders. Effective communication of quantitative results should focus on key insights, backed by appropriate visualizations and statistical evidence, and avoid overwhelming the audience with excessive details.
Conclusion
Analyzing quantitative data is a powerful tool for uncovering insights, validating hypotheses, and making informed decisions across various domains. By following a structured approach—defining your research question, collecting quality data, applying the right statistical techniques, and interpreting the results carefully—you can turn raw numbers into valuable knowledge. As technology continues to evolve, the tools and methods for analyzing quantitative data will only become more sophisticated, making data analysis an increasingly vital skill for researchers, analysts, and decision-makers in every field.
Incorporating sound data analysis practices into your work not only improves the accuracy of your findings but also provides a foundation for future investigations, enabling continuous learning and progress. Whether in a corporate setting, academic research, or healthcare study, quantitative data analysis serves as a crucial process for understanding and solving complex problems.