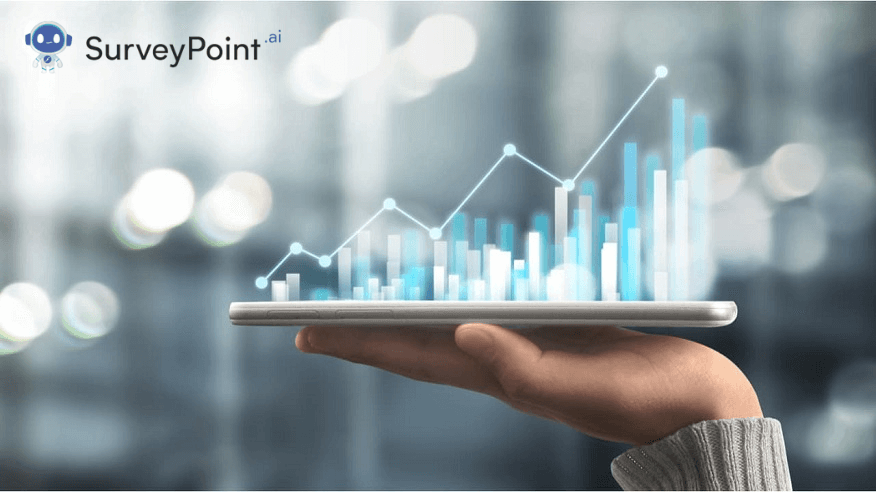
Inferential statistics are an essential cornerstone of statistics used for decision-making. As the name implies, it is all about inferring conclusions, not concluding them. Scientific data enables us to infer information based on numbers.
Usually, this question follows with a discussion of the difference between quantitative and descriptive statistics. In its simplest form, descriptive statistics describe data.
By determining the average, the standard deviation, the positive or negative skew of the data, and then looking at its range, we can better understand the data. However, if we are analysing a population sample, we should project our findings to the entire population. Several numbers indicate whether or not an extrapolation can be made based on population size. As a result, inferential statistics are necessary to test the significance of the hypothesis and results. P-values represent chance probabilities. Statistical significance requires a probability of at least 95% that reflects the reality on the ground, not random chance.
Estimation and hypothesis testing are the two conventional forms of statistical inference. The purpose of estimation is to predict the parameter of a population. In contrast, hypothesis testing is to either prove or disprove the null hypothesis that the researcher formulated.
Estimation
The purpose of estimation is to assign a value to a population parameter based on the data collected from a subset of the entire population.
An estimate of a population parameter is the value put on the whole population from a small sample. The estimation process involves:
- Selecting a sample.
- Collecting the relevant data.
- Computing the value of sample statistics.
- Assigning a value to the relevant parameter.
Two types of estimates are commonly employed to estimate population parameters:
- point estimates, which provide an estimated value, and
- interval estimates, which offer a range of values.
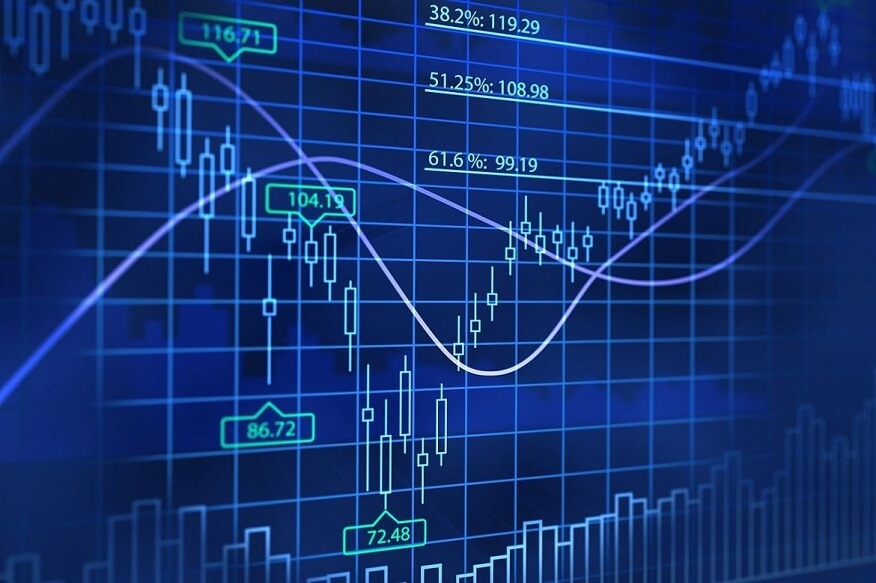
A) Point Estimation
A point estimate is one of the most accurate population parameter estimates. Researchers can select a sample to obtain statistics and a corresponding population parameter estimate.
The arithmetic mean of all population members can be used for unbiased estimation of a population parameter by calculating the mean of a randomly selected subset of that population. Hence, the sample mean can be defined as the arithmetic mean of the sample’s components. Aside from mean and standard deviation, other population metrics, such as proportion, can also be estimated using sample statistics.
As mentioned before, a point estimate represents a single number, but the number may not represent the parameter’s true value. A margin of error must always accompany a point estimate, so interval estimation is more valuable.
B) Interval Estimation
As its name suggests, an interval estimate provides an interval along with a computed likelihood of capturing the parameter. An interval encircles a point estimate instead of assigning a single value to a population parameter.
Each interval formed based on a specific confidence level is known as the confidence interval. An interval’s confidence level reveals how certain one can be that the interval contains the genuine population parameter.
The researcher can also calculate confidence intervals for proportions, correlations and comparable mean correlations, and mean confidence intervals.
Hypothesis Testing
A hypothesis is a supposition regarding a population parameter. In this case, we intend to test the null hypothesis, which suggests that the actual and hypothesis values do not differ. The testing of the hypothesis begins with the assumption of “no differences.”
Strategies for Checking Hypothesis
Researchers typically apply the following steps sequentially when testing the hypothesis to accept or reject the null hypothesis.
- Create a null hypothesis and an alternative hypothesis.
- Choosing an appropriate critical value, for example, a 95% confidence level, would be appropriate.
- Assessing the significance of each region and determining the location of the crucial region.
- To define the critical region’s bounds, select a relevant test statistic from the test statistics table.
- Calculate the observed value of the chosen statistic using the test statistics and sample observations.
- Examine the tabulated value of the chosen statistics compared to the sample value. Unless the calculated statistics are in the critical region, the researchers must reject the null hypothesis and adopt an alternative hypothesis. Otherwise, they can indicate insufficient evidence to reject and adopt an alternative theory.
Types of Inferential Statistic Tests
Inferential tests can be used for various purposes, whether to compare one group to a hypothetical value or with other groups. Tests can be further classified into broad categories when comparing across groups.
- Compare two groups (Unpaired groups, Paired groups) and
- Compare three or more groups (Matched groups, Unmatched groups).
Depending on the type of variable, an inferential test can be
- parametric (for ratio variables) or,
- non-parametric (for nominal and ordinal variables).
The means, medians, and ranks of two or more groups are compared to calculate inferential results. It is possible to use a wide range of statistical tests to compare data, including t-tests, ANOVA, the Chi-square test, binomial tests, and Friedman tests.
Another option for making an inference is to test for correlation or regression. Furthermore, Spearman’s r is appropriate for intervals and ratios when data do not fit the normal distribution. Pearson’s r is the most accurate test when determining if two variables are related.
The only valid statistical test for use with nominal variables is the chi-square test of independence. A regression test assesses whether a change in one predictor variable caused a variance in outcome. Various regression tests, such as linear and logistic regression, can be used based on the number and types of predictors and outcomes.
Take the Hassel Out From Your Survey Creation with SurveyPoint.ai
Creating surveys doesn’t have to be difficult, especially if you have the right expertise, digital solutions, and templates at your disposal. Sign up for FREE at SurveyPoint and take the hassle out of data collection and analysis.
Kultar Singh – Chief Executive Officer, Sambodhi