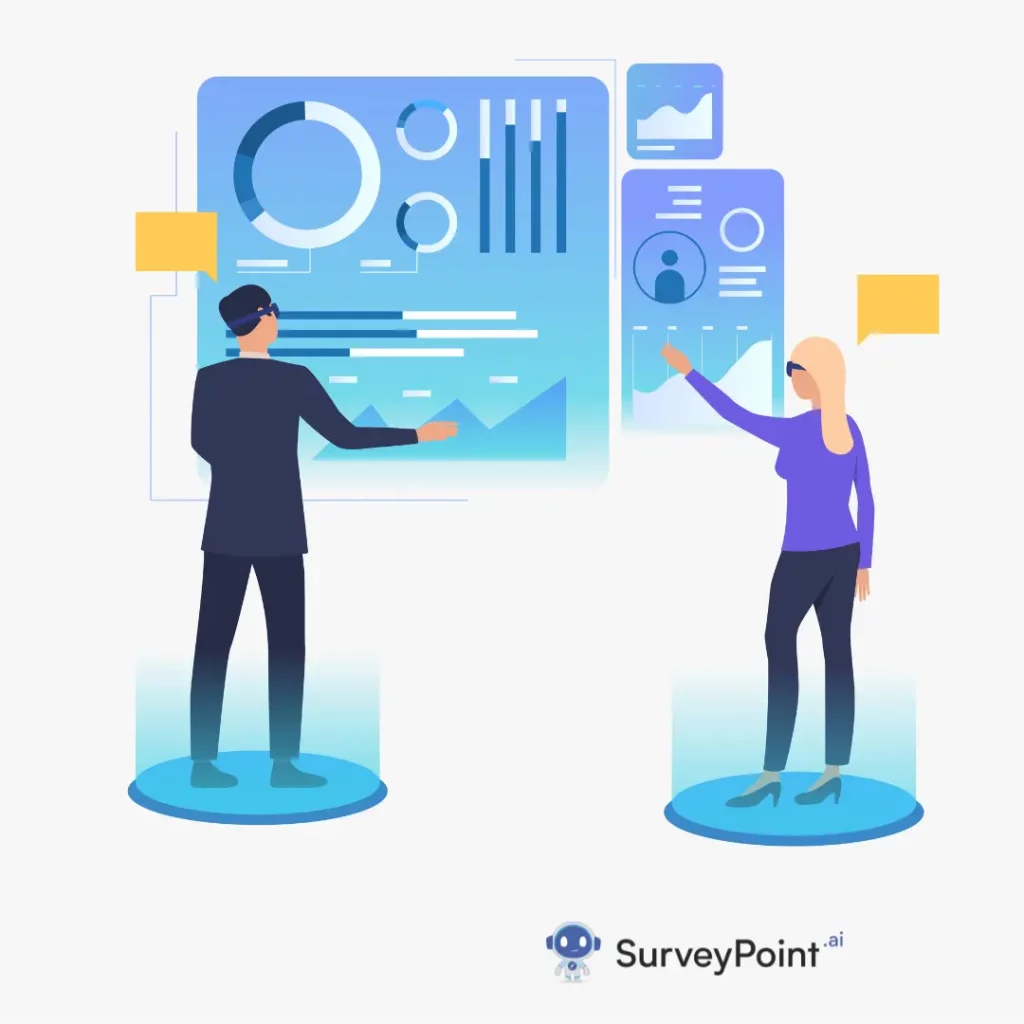
Data analysts and scientists must use the right tools to extract valuable insights as data becomes more crucial and intricate. In this article, we will thoroughly explore the distinguishing features, use cases, and considerations for Snowflake and Databricks, aiming to shed light on which platform might be the optimal choice for your specific data needs.
Understanding the Core Objectives
- Snowflake: The Elastic Data Warehouse
Snowflake, a best-in-class cloud data warehouse, focuses on delivering instant elasticity and the unique separation of storage and computing. Its architecture allows users to scale resources dynamically, optimising performance while ensuring cost-effectiveness.
Snowflake’s commitment to simplicity and efficiency has earned it a reputation as a go-to solution for organisations seeking a robust data warehousing platform in the cloud.
- Databricks: Unified Analytics Powerhouse
In contrast, Databricks initially emerged as a cloud service centred around Apache Spark. Over time, it evolved into a unified analytics platform, aiming to be a comprehensive solution for data engineering, analytics, and machine learning.
Databricks provides a collaborative environment where data professionals can seamlessly integrate their work, fostering teamwork and efficiency across various data-related tasks.
Decoding Differences: Snowflake vs. Databricks
Architectural Approach
- Snowflake: multi-cluster elasticity, allowing independent scaling of compute resources.
- Databricks is a unified platform architecture built around Apache Spark for collaborative analytics.
Use Case Specialisation
- Snowflake specialises in data warehousing, excelling in storing and analysing structured and semi-structured data.
- Databricks is a comprehensive analytics and machine learning platform covering data engineering to advanced analytics.
Integration Capabilities
- Snowflake: Focus on data warehousing, with fewer integrations for collaborative analytics tools.
- Databricks: Extensive integration capabilities with popular data science libraries and BI tools.
Scalability Approach
- Snowflake: elastic scaling for dynamic resource adjustment based on workload demands.
- Databricks: horizontal scaling to efficiently handle large datasets and parallel processing.
Cost Structure
- Snowflake: transparent cost structure, aligning with dynamic scaling and resource usage.
- Databricks: Unified platform costs cover various analytics and machine learning capabilities.
Navigating Databricks vs. Snowflake: Key Considerations for Platform Selection
Scalability
- Snowflake leverages elastic scaling for adaptive resource allocation based on workload demands.
- Databricks offers horizontal scaling for efficient handling of diverse datasets.
Performance Metrics
- Snowflake: Instant elasticity and separation of storage and computing contribute to optimal performance.
- Databricks employs Apache Spark’s distributed computing for powerful large-scale data processing.
Cost Management
- Snowflake: A transparent and adaptable cost structure aligns with usage patterns during active processing.
- Databricks: Unified platform costs cover comprehensive analytics and machine learning capabilities.
Use Case Specialisation
- Snowflake excels in data warehousing, particularly for structured and semi-structured data.
- Databricks is a unified platform catering to data engineering, analytics, and machine learning.
Collaboration and Integration
- Snowflake primarily focuses on data warehousing, with less emphasis on collaborative analytics.
- Databricks fosters collaboration, seamless integration, and teamwork across diverse data-related tasks.
Budgetary Impact
- Snowflake: Costs are influenced by scaling and resource usage, depending on the dynamics.
- Databricks simplifies budgeting with unified costs for various analytics capabilities.
Workload Adaptability
- Snowflake is adaptable to fluctuating workloads, ensuring resource optimisation.
- Databricks: Offers a cohesive team environment, potentially streamlining overall costs.
Organisational Goals
- Snowflake is suitable for organisations with a primary focus on data warehousing.
- Databricks are ideal for organisations seeking a unified platform for diverse data-related requirements.
Final Takeaways
In conclusion, Snowflake and Databricks emerge as formidable players in the cloud data platform arena, each with strengths and specialisations. The choice between the two ultimately hinges on your organisation’s specific needs and priorities.
Consider Snowflake if:
- You prioritise instant elasticity and the separation of storage and computing.
- Your primary focus is data warehousing, particularly for structured and semi-structured data.
- Scalability and cost-effectiveness are critical factors in your decision-making process.
Consider data bricks if:
- You seek a unified platform for data engineering, analytics, and machine learning.
- Collaboration and seamless integration of diverse data-related tasks are paramount.
- Your organisation requires a comprehensive solution that covers a wide range of analytics and machine learning capabilities.
Ultimately, the choice between Snowflake and Databricks depends on your organisation’s goals and priorities. Both platforms have proven themselves in their respective niches, offering robust solutions for working with data in the cloud.
Consider your organisation’s unique requirements, workload characteristics, and desired scope of functionalities when deciding. Whether you prioritise data warehousing excellence or a unified analytics powerhouse, both Snowflake and Databricks have the potential to elevate your data endeavours in the cloud.