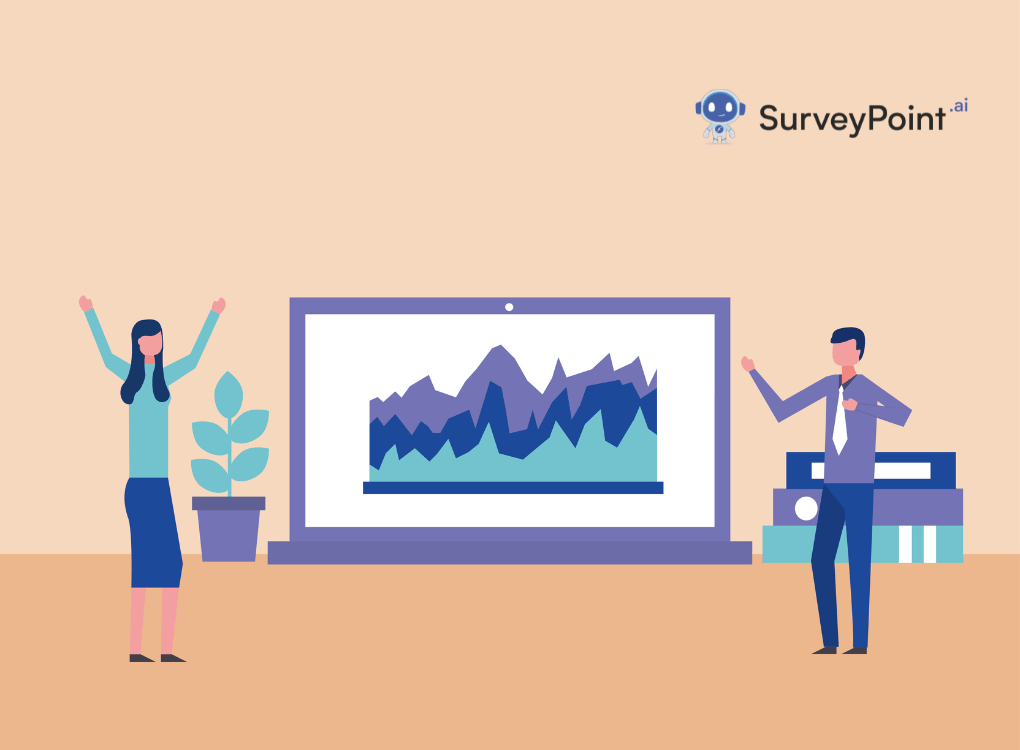
In an era where data reigns supreme, the presentation of information holds immense power. However, with great power comes great responsibility. Misleading data visualization has become a prevalent issue in various fields, ranging from journalism to academia to corporate presentations. This blog aims to shed light on the deceptive techniques often employed in data visualization, exploring examples that highlight the pitfalls to avoid.
What is Considered Misleading Data Visualization?
Misleading data visualization encompasses various techniques that distort or misrepresent information, leading to inaccurate interpretations. This can include manipulating scaling to exaggerate or minimize differences between data points, truncating axes to amplify trends, or cherry-picking data to support a particular narrative while disregarding contradictory information. Improper labeling, inappropriate visualization types, and exaggerated visual elements also contribute to misleading representations.
Omitting context, manipulating data, overlaying multiple variables, and using misleading visual cues further compound the issue. Misleading visualizations erode trust in the integrity of data and those presenting it, potentially leading to misinformed decision-making, wasted resources, and brand damage. By being aware of these techniques and adhering to principles of transparency, accuracy, and clarity, practitioners can mitigate the risk of misleading data visualization and ensure that information is conveyed truthfully and responsibly.
Impact of Misleading Data Visualization:
The consequences of misleading data visualization extend far beyond simple misinterpretation. They can erode trust, lead to poor decision-making, and perpetuate misinformation. In fields where data-driven decisions are critical, such as public policy and healthcare, the implications can be dire.
Certainly, here are the top five impacts of misleading data visualization:
- Misinformed Decision-Making: Misleading data visualizations can lead to decisions based on flawed interpretations, resulting in ineffective strategies, misallocated resources, and missed opportunities.
- Loss of Trust and Credibility: Misleading visualizations undermine trust in the reliability of data and the integrity of those presenting it, damaging reputations and eroding confidence in future data-driven initiatives.
- Wasted Resources: Organizations may allocate resources based on faulty insights from misleading visualizations, resulting in wasted time, money, and effort on initiatives that fail to deliver desired outcomes.
- Reputational Damage: When deceptive data visualization techniques are exposed, it can negatively affect the standing of the individuals, groups, or institutions involved, which can affect how stakeholders perceive each other and their relationships with them.
- Impact on Public Perception and Policy: Misleading visualizations can shape public opinion and influence policy decisions, leading to misinformation and hindering evidence-based policymaking, with implications for societal well-being and progress.
Avoiding Misleading Data Visualization for Better Decisions:
To combat misleading data visualization, practitioners must adhere to principles of transparency, accuracy, and clarity.Consider implementing the following strategies to avoid misleading data visualization and ensure better decisions:
- Transparency and Clarity: Clearly communicate the purpose and context of the data visualization. Provide detailed explanations of the methodology used and any assumptions made in the analysis.
- Use Appropriate Visualization Techniques: Choose visualization formats that best represent the data accurately and effectively. Different types of data may require different visualization methods, such as bar charts for comparing discrete categories or line graphs for showing trends over time.
- Consistent Scaling: Maintain consistent scaling across all axes to prevent distortion and ensure accurate comparisons between data points. Avoid truncating axes or using exaggerated scaling that may skew the perception of the data.
- Avoid Extraneous Visual Elements: Minimize unnecessary clutter and distractions in the visualization. Remove elements such as unnecessary gridlines, background imagery, or excessive annotations that do not contribute to understanding the data.
- Labeling and Annotations: Clearly label data points, axes, and units to provide context and aid interpretation. Use annotations to highlight important trends or outliers and explain any anomalies in the data.
- Provide Context: Present data in the context of relevant benchmarks, historical trends, or external factors that may influence interpretation. Avoid presenting data in isolation and provide a comprehensive view of the broader picture.
- Verify Data Integrity: Ensure the accuracy and integrity of the data used in the visualization. Verify sources, cross-check data points, and conduct thorough data validation to mitigate the risk of errors or inaccuracies.
- Sensitivity Analysis: Conduct sensitivity analysis to assess the impact of different assumptions or parameters on the results. Explore various scenarios and sensitivity ranges to understand the robustness of the findings.
- Peer Review and Feedback: Seek feedback from peers, subject matter experts, or stakeholders to validate the accuracy and effectiveness of the visualization. Incorporate constructive criticism and iterate on the design to improve clarity and understanding.
- Educate and Train Users: Provide training and educational resources to users on interpreting data visualizations effectively. Empower them with the knowledge and skills to critically evaluate visualizations and make informed decisions based on the data presented.
By implementing these strategies, organizations can enhance the integrity and effectiveness of their data visualizations, leading to better-informed decisions and insights.
Conclusion
In a world inundated with data, the responsibility to present information accurately and ethically is paramount. By understanding the techniques of misleading data visualization and the impact they can have, we can strive for greater transparency and integrity in how we communicate data. Let us embrace the power of visualization as a tool for enlightenment rather than deception, ensuring that our data tells the true story it intends to convey.