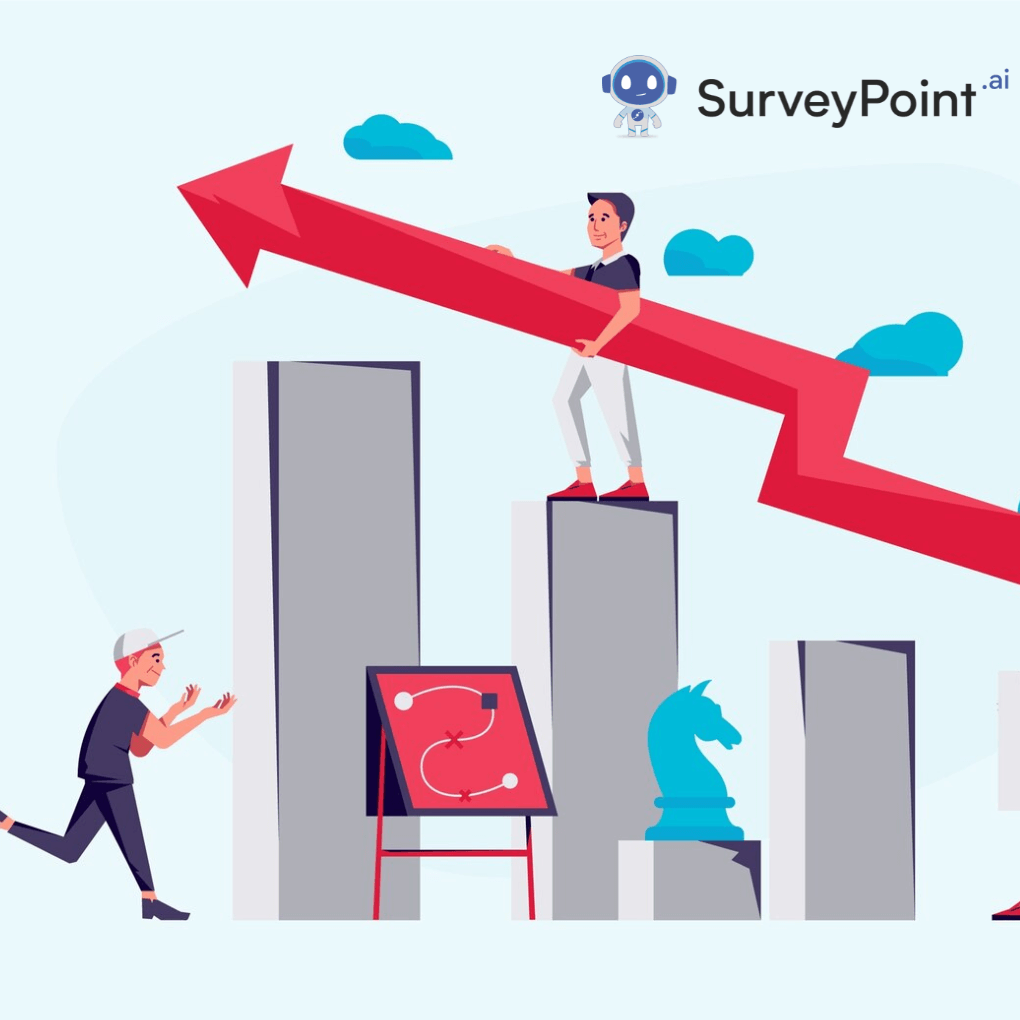
In the field of statistics, accurately interpreting data is critical for making informed decisions. Two fundamental concepts that play a vital role in this process are margin of error and confidence interval. Both of these terms help quantify uncertainty in data, particularly when analyzing survey results and experimental data.
This blog aims to demystify these concepts, exploring their definitions, differences, calculations, applications, and implications in real-world scenarios. By the end, you’ll have a solid understanding of how to apply these concepts effectively in your research and data analysis.
Understanding Margin of Error
What is Margin of Error?
margin of error is a statistic that expresses the amount of random sampling error in a survey’s results. It provides a range around a survey estimate to indicate where the true value is likely to lie. For example, if a poll indicates that 60% of respondents favor a particular policy with a margin of error of ±3%, it means that the true support is expected to be between 57% and 63%.
The margin of error is commonly expressed as a percentage and is crucial in surveys, polls, and other statistical studies where a sample is used to represent a larger population. It helps convey the reliability of the results, allowing readers to understand the potential variation.
Factors Influencing Margin of Error
Several key factors influence the margin of error, including:
1. Sample Size
One of the most significant determinants of margin of error is the sample size. Generally, larger sample sizes lead to smaller margins of error. This is because larger samples tend to more accurately represent the population, reducing variability in the results. For instance, a survey of 1,000 respondents will usually yield a smaller margin of error compared to a survey of 100 respondents.
2. Population Variability
The inherent variability within the population being studied also impacts the margin of error. If the population has diverse opinions or characteristics, the margin of error will likely be larger. For example, in a survey assessing the average height of a population with varied physical characteristics, the margin of error might be greater than in a more homogenous group.
3. Confidence Level
The confidence level chosen by researchers also affects the margin of error. Common confidence levels are 90%, 95%, and 99%. A higher confidence level indicates greater certainty about the results, but it also results in a larger margin of error. For example, a 95% confidence level might yield a larger margin of error than a 90% confidence level, as researchers aim to cover more potential variability.
Real-World Examples
To illustrate the concept of margin of error, consider a recent survey conducted during a political campaign. The survey reports that 55% of likely voters support Candidate A, with a margin of error of ±4%. This means that the actual support for Candidate A could realistically range from 51% to 59%. The margin of error thus provides essential context for interpreting the survey results, allowing stakeholders to gauge the reliability of the findings.
Another example could be a market research study assessing customer satisfaction with a product. If the study finds that 70% of customers are satisfied, with a margin of error of ±3%, it indicates that the true satisfaction level is likely between 67% and 73%. This information can significantly influence marketing strategies and product development.
Understanding Confidence Interval
What is Confidence Interval?
A confidence interval is a range of values derived from sample data that is likely to contain the population parameter. It provides an estimate of uncertainty around a point estimate (such as a sample mean) and is expressed with a certain confidence level, typically 90%, 95%, or 99%.
For example, if a study reports a 95% confidence interval of 100, 120 for the mean height of a population, it suggests that researchers can be 95% confident that the true mean height lies within this range.
Calculation of Confidence Interval
Calculating a confidence interval involves several steps:
1. Determine the Sample Statistic
Begin by calculating the sample mean or proportion, which serves as the point estimate.
2. Calculate the Standard Error
The standard error (SE) measures the dispersion of the sample statistic and is calculated as follows:
[SE = \frac{s}{\sqrt{n}}
]
where ( s ) is the standard deviation of the sample and ( n ) is the sample size.
3. Determine the z-Score
Choose a confidence level and find the corresponding z-score from the z-table. For example, a 95% confidence level typically corresponds to a z-score of approximately 1.96.
4. Calculate the Margin of Error
The margin of error (ME) is calculated by multiplying the standard error by the z-score:
[ME = z \times SE
]
5. Construct the Confidence Interval
Finally, construct the confidence interval using the sample statistic and the margin of error:
[\text{Confidence Interval} = \text{Point Estimate} PM \text{Margin of Error}
]
Practical Applications
Confidence intervals have numerous applications across various fields. In clinical trials, for example, researchers might report the effectiveness of a new drug with a 95% confidence interval of (1.5, 2.5). This indicates that researchers are 95% confident that the true treatment effect lies within this range.
In market research, a company might survey customers about a new product, reporting a 90% confidence interval for customer satisfaction of (70%, 80%). This information is invaluable for understanding potential market acceptance and guiding marketing strategies.
Comparing Margin of Error and Confidence Interval
Key Differences
While margin of error and confidence intervals are often used interchangeably, they are distinct concepts with specific roles:
- Margin of Error: This term quantifies the uncertainty associated with survey results. It typically indicates how far the sample estimate is likely to be from the true population parameter.
- Confidence Interval: A confidence interval provides a range of values that is likely to contain the population parameter. It combines the margin of error with the point estimate to give a broader perspective on the estimate’s reliability.
When to Use Each
When reporting survey results, it’s essential to present the margin of error to provide context for the findings. For example, a political poll might state that 52% of voters support a candidate with a margin of error of ±3%. This helps convey the uncertainty around the estimate.
In contrast, confidence intervals are particularly useful in hypothesis testing and inferential statistics, as they provide a range within which the true population parameter is expected to fall. For instance, in a clinical trial, researchers might report that the mean reduction in symptoms is 4 units, with a 95% confidence interval of (2, 6). This conveys a more comprehensive understanding of the treatment’s effectiveness.
Misconceptions and Common Mistakes
A common misconception is that a smaller margin of error always equates to better survey results. While a smaller margin indicates more precise estimates, it’s crucial to consider sample size and variability. Additionally, some may misinterpret confidence intervals, viewing them as guarantees rather than ranges of probable values. It’s important to communicate clearly that confidence intervals provide a level of certainty, not an absolute measure.
Statistical Significance and Its Relation
Understanding Statistical Significance
Statistical significance is a determination of whether the results of a study are likely to be due to chance. It is often expressed through p-values and helps researchers decide whether to reject the null hypothesis. Margin of error and confidence intervals are critical in this context, as they help define the boundaries for what is considered statistically significant.
Importance in Research
In research, margin of error and confidence intervals are vital for establishing the validity of findings. If a confidence interval for a treatment effect does not include zero (the null hypothesis), it suggests that the results are statistically significant. For instance, if a drug shows a mean improvement of 4 units with a confidence interval of (1, 7), researchers can infer that the drug likely has a positive effect.
In contrast, if a confidence interval for a proportion includes the null value, it indicates that there is no statistically significant difference. This understanding helps researchers communicate findings accurately and avoid drawing misleading conclusions.
Case Studies and Practical Examples
Case Study 1: Political Polling
Consider a political polling scenario in which a survey indicates that 48% of likely voters support Candidate A, with a margin of error of ±3%. This means that the actual support could realistically range from 45% to 51%. If the poll also reports a 95% confidence interval of (45%, 51%), it provides further context, reinforcing the idea that Candidate A has a viable chance in the election.
Political analysts can use this information to gauge the candidate’s standing and inform campaign strategies. If subsequent polls show a consistent margin of error and confidence interval, it builds confidence in the data’s reliability.
Case Study 2: Market Research
In market research, a company might conduct a survey assessing customer satisfaction with a new product. If the survey finds that 70% of customers are satisfied, with a margin of error of ±5%, the confidence interval would be (65%, 75%). This information is crucial for the company to make strategic decisions about product improvements and marketing efforts.
If the confidence interval is significantly above a predetermined satisfaction threshold (e.g., 60%), the company may decide to promote the product heavily. Conversely, if customer satisfaction falls below this threshold, the company might invest in product enhancements.
Conclusion
Understanding the differences between margin of error and confidence intervals is essential for interpreting data accurately. While both measures convey uncertainty in estimates, they serve distinct purposes in statistical analysis. The margin of error provides insight into the precision of survey results, while confidence intervals offer a broader range of likely values for population parameters.By mastering these concepts, researchers and analysts can improve the reliability of their findings and make more informed decisions in various fields, including healthcare, marketing, and social sciences.