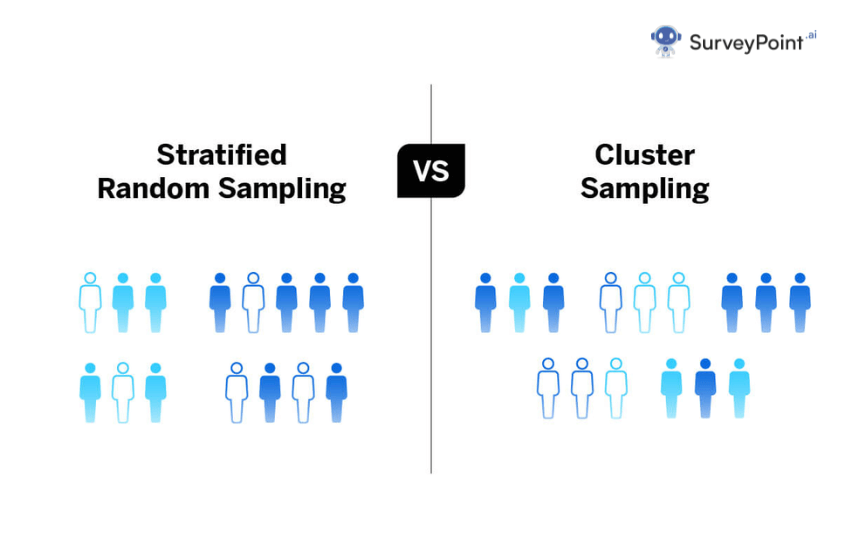
Sampling is a crucial technique in statistics and research, enabling scholars, businesses, and organizations to make generalizations about a population without needing to study every individual. Among the various sampling methods, stratified random sampling and cluster sampling are two of the most commonly used techniques. While they both aim to ensure that a sample is representative of the larger population, they do so in fundamentally different ways.
In this blog, we will explore the differences between stratified random sampling and cluster sampling, their advantages and disadvantages, and when to use each approach.
What Is Stratified Random Sampling?
Stratified random sampling is a technique used to ensure that specific subgroups (strata) within a population are adequately represented in the sample. The process involves dividing the population into distinct, non-overlapping subgroups based on a characteristic of interest, such as age, income, gender, education level, or geographic location. Once the strata are defined, a random sample is taken from each subgroup in proportion to the size of the subgroup within the population. This approach is particularly useful when the researcher believes that the variability within the population is better understood when examined through these subgroups.
Steps in Stratified Random Sampling:
- Define the Population: Identify the entire population from which the sample will be drawn.
- Identify Strata: Divide the population into different strata based on relevant characteristics (e.g., income levels, education, or geographical location).
- Determine Sample Size per Stratum: Decide how many individuals will be sampled from each stratum. This can be proportional (in proportion to the stratum’s size in the population) or equal (an equal number from each stratum, regardless of size).
- Random Sampling: Within each stratum, a simple random sample is selected.
- Combine Samples: The samples from each stratum are then combined to form the final sample.
Advantages of Stratified Random Sampling:
- Improved Precision: Since each stratum is proportionally represented, the resulting sample tends to be more precise and reliable in estimating the overall population characteristics.
- Better Representation of Subgroups: This method ensures that small or minority subgroups are not overlooked in the sampling process, leading to a more balanced and diverse sample.
- Reduced Sampling Variability: By controlling for known variables (strata), this technique can reduce variability and result in a sample that better reflects the population.
Disadvantages of Stratified Random Sampling:
- Complexity in Stratification: Determining the appropriate strata and ensuring accurate classification can be complex and time-consuming.
- Data Availability: Stratified random sampling requires detailed knowledge of the population and the characteristics of each stratum, which may not always be available or easily obtainable.
- Inflexibility: If the strata are incorrectly defined or irrelevant to the research, the results may be skewed or misleading.
What Is Cluster Sampling?
Cluster sampling is another method of sampling that divides the population into clusters, typically based on geographical areas or natural groupings. Instead of sampling individuals from every cluster, the researcher randomly selects a few clusters and then collects data from all the individuals within those selected clusters. This method is often used when it is difficult or impractical to create a complete list of all individuals in the population, or when populations are geographically dispersed, and a more cost-effective solution is needed.
Steps in Cluster Sampling:
- Define the Population: Identify the overall population to be studied.
- Divide the Population into Clusters: The population is divided into clusters, which may be based on geography, organization, or any natural grouping (e.g., schools, cities, neighborhoods).
- Randomly Select Clusters: A random selection of clusters is made.
- Collect Data from Selected Clusters: All individuals within the selected clusters are included in the sample.
- Combine the Data: The data from all the sampled clusters are combined to form the final sample.
Advantages of Cluster Sampling:
- Cost-effective: It can be far cheaper and logistically easier than other methods, especially when the population is spread out geographically.
- Practical: Cluster sampling works well when it is difficult or costly to gather information from the entire population, such as in large, dispersed populations.
- Efficiency: Researchers can collect large amounts of data from fewer locations or clusters, which can lead to significant time savings.
Disadvantages of Cluster Sampling:
- Less Precision: Since the sample is drawn from entire clusters, it can sometimes be less precise and have higher sampling error than other methods.
- Risk of Homogeneity: If the clusters are too similar to each other, the sample may not capture the full variability of the population.
- Potential for Bias: If the selected clusters are not representative of the population, the results can be biased and not generalizable.
Key Differences Between Stratified Random Sampling and Cluster Sampling
While both stratified random sampling and cluster sampling aim to improve the representativeness of a sample, they do so using different techniques. Below are the primary distinctions between these two methods:
Aspect | Stratified Random Sampling | Cluster Sampling |
---|---|---|
Division of Population | Divides the population into subgroups (strata) based on characteristics. | Divides the population into clusters, which may be based on geography or organization. |
Sampling Unit | Individuals within each stratum are randomly selected. | Entire clusters are randomly selected, and all individuals within selected clusters are included. |
Goal | To ensure all subgroups are represented proportionally. | To reduce costs and logistical complexity by focusing on clusters. |
Sample Composition | Each stratum is represented in the sample based on its proportion in the population. | Entire clusters are represented in the sample, leading to a more homogeneous sample if the clusters are similar. |
Sample Size | Typically larger, as individuals are sampled from each stratum. | Usually smaller, since entire clusters are sampled. |
Data Availability | Requires detailed information to divide the population into strata. | Can be used when the population is geographically dispersed or difficult to access. |
Precision | Generally more precise, as it accounts for variability across strata. | May have higher variability due to the homogeneity of selected clusters. |
When to Use Stratified Random Sampling?
Stratified random sampling is particularly advantageous when the researcher wants to ensure that certain subgroups of the population are well-represented in the sample. It is ideal for:
- Ensuring Representation of Subgroups: If there are small or underrepresented subgroups (such as specific age groups, ethnicities, or income brackets) that are of particular interest to the study, stratified random sampling ensures these groups are included.
- Studies Focused on Comparisons Between Groups: If the goal is to compare the characteristics of different strata (e.g., comparing income levels, educational backgrounds, or regions), stratified sampling provides more precise estimates.
- Reducing Sampling Error: When the variability within each stratum is smaller than the variability across the entire population, stratified random sampling can significantly reduce sampling error.
When to Use Cluster Sampling?
Cluster sampling is often chosen when the researcher faces logistical or financial constraints. It is best suited for:
- Large or Geographically Dispersed Populations: If the population is spread across a wide area or is difficult to access, cluster sampling allows the researcher to sample a few specific areas or groups, saving both time and money.
- Limited Access to Population Lists: In cases where it is difficult to access a full list of individuals in the population but clusters (e.g., schools, neighborhoods, or organizations) can be easily identified, cluster sampling is ideal.
- Preliminary Studies or Exploratory Research: Cluster sampling can be used in the early stages of research when a more in-depth study is not yet feasible, as it provides a cost-effective way to gather data.
Example Scenarios
- Stratified Random Sampling:
Suppose a research team is conducting a national survey on educational attainment in the U.S. They might stratify the population by region (North, South, East, and West) to ensure that each region is appropriately represented in the sample. Within each region, random samples of individuals are then selected, ensuring that the final sample reflects the population’s geographic diversity. - Cluster Sampling:
A health organization wants to survey the prevalence of smoking among teenagers in a large country. Rather than trying to gather information from all schools across the country, they may divide the country into regions and then randomly select a few schools (clusters) from each region. They would then survey all students in the selected schools, which reduces the cost and complexity of data collection.
Conclusion
Both stratified random sampling and cluster sampling are invaluable tools for researchers looking to create representative samples from a larger population. While stratified random sampling aims for precision by ensuring proportional representation of various subgroups, cluster sampling prioritizes cost-effectiveness and convenience, often at the expense of precision. The choice between the two methods depends largely on the research objectives, the nature of the population, the available resources, and the level of accuracy required in the study’s findings.
By understanding the strengths and limitations of each technique, researchers can make more informed decisions about which sampling method best suits their study, leading to more reliable, valid, and generalizable results.