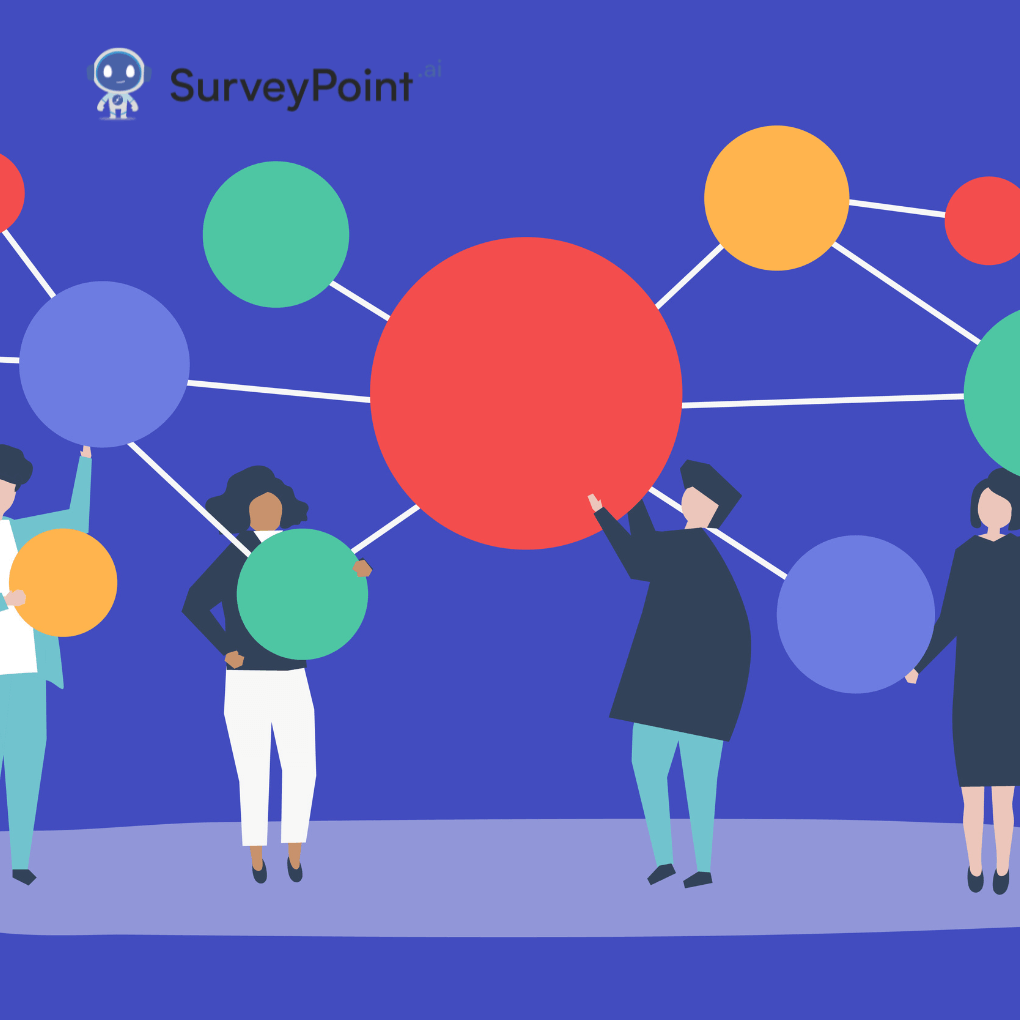
Sampling is a cornerstone of research and data analysis, providing insights into larger populations without the time and cost of examining each individual. While simple random sampling is widely known, methods like stratified and cluster sampling are often preferred in specific situations where the population is large and complex. Each of these sampling methods has its own unique approach, strengths, and weaknesses, and selecting the right one can greatly impact the quality of insights gathered.
Here, we’ll explore stratified and cluster sampling, examining their differences, when to use each, and practical examples to illustrate their applications.
Understanding Strategic Sampling
Stratified sampling is a sampling technique in which a population is divided into distinct subgroups known as strata based on specific characteristics. These characteristics could include age, gender, income level, education, or any other factors relevant to the research question. Once the population is divided, researchers randomly select samples from each stratum, ensuring that each subgroup is proportionately represented in the sample.
The main purpose of stratified sampling is to improve the precision of the results by ensuring that all key subgroups within the population are represented. By drawing samples from each stratum, researchers can make comparisons and analyze data with greater accuracy and reliability.
Key Features of Strategic Sampling
- Diverse Population: Stratified sampling is ideal when the population is diverse and contains distinct subgroups that need to be individually analyzed.
- Proportional Representation: This method allows researchers to ensure each subgroup is represented proportionally, reducing sampling bias.
- Improved Accuracy: Because stratified sampling captures the diversity within the population, it often results in higher accuracy in the data analysis process.
Example of Stratified Sampling
Let’s say a university wants to conduct a survey on student satisfaction. The university’s student population is composed of students across different levels (freshmen, sophomores, juniors, and seniors). Using stratified sampling, the university could divide the student body into these four strata and then randomly select an equal or proportional number of students from each group.
By ensuring that each academic level is represented, the university can gather accurate insights that reflect the perspectives of all students, not just those at a specific stage in their academic journey. This way, stratified sampling allows for more meaningful comparisons between different groups and provides a comprehensive picture of student satisfaction.
When to Use Stratified Sampling
Stratified sampling is particularly effective in cases where:
- The population has distinct subgroups. If there are identifiable subgroups within a population that could affect the research outcome, stratified sampling helps ensure all groups are represented.
- Precise comparison is needed between subgroups. When the goal is to compare results across subgroups, stratified sampling enables accurate, subgroup-specific insights.
- The sample needs to reflect the population accurately. For research aiming to represent the entire population accurately, stratified sampling reduces bias and improves the generalizability of the results.
Advantages and Disadvantages of Stratified Sampling
Advantages:
- Higher Precision: Stratified sampling ensures a precise representation of all subgroups, which enhances data accuracy.
- Reduced Bias: By selecting from each stratum, stratified sampling reduces the likelihood of sampling bias, leading to more reliable results.
- Effective Comparisons: Stratified sampling allows researchers to compare different subgroups within the population effectively.
Disadvantages:
- Complexity: Stratified sampling can be complex to implement as it requires identifying relevant strata and dividing the population accordingly.
- Time-Consuming: Stratification and random sampling from each stratum can be time-consuming, especially with a large population.
- Data Requirements: To conduct stratified sampling, researchers need comprehensive data on the entire population to categorize it into meaningful strata.
Understanding Cluster Sampling
Cluster sampling is another sampling technique where the population is divided into clusters, usually based on geographical location or other natural groupings. Unlike stratified sampling, where samples are drawn from every stratum, cluster sampling involves randomly selecting entire clusters and including all individuals within those clusters in the sample.
Cluster sampling is often used when the population is widely spread out geographically, and accessing the entire population would be logistically difficult or costly. It’s especially useful in large-scale studies where it’s impractical to reach every individual, as it enables researchers to focus on specific clusters.
Key Features of Cluster Sampling
- Geographically Dispersed Population: Cluster sampling is ideal for populations spread out across large geographical areas.
- Random Selection of Clusters: Instead of sampling individuals, researchers randomly select entire clusters, which can simplify data collection.
- Cost-Effective: By sampling clusters, researchers can significantly reduce travel and data collection costs.
Example of Cluster Sampling
Consider a nationwide health study that aims to measure the health outcomes of residents across the country. The population is too large and geographically dispersed for a simple random sample to be feasible. Instead, researchers could use cluster sampling by randomly selecting several cities or regions (clusters) across the country and surveying all individuals within these selected clusters.
By focusing on specific clusters, the researchers can still obtain relevant data on health outcomes while minimizing the logistical challenges and costs associated with a large-scale survey.
When to Use Cluster Sampling
Cluster sampling is particularly effective in cases where:
- The population is geographically dispersed. If the population is spread out, cluster sampling reduces the need for extensive travel and logistics.
- Resources are limited: Cluster sampling is often more cost-effective, as it involves sampling groups rather than individual members.
- Quick and efficient sampling is needed. Cluster sampling allows for fast data collection without compromising on sample size.
Advantages and Disadvantages of Cluster Sampling
Advantages:
- Cost-Effective: Cluster sampling is often less expensive, as it requires fewer resources and allows for efficient data collection.
- Reduced Time and Effort: By focusing on specific clusters, researchers can complete studies faster than if they surveyed individuals across a dispersed population.
- Convenience for Large Populations: Cluster sampling simplifies the process when dealing with large, geographically dispersed populations.
Disadvantages:
- Risk of Sampling Bias: If clusters are not representative of the population as a whole, the results may be biased.
- Lower Precision: Cluster sampling can be less precise than stratified sampling because there is less control over the representation of individual characteristics.
- Potential for High Variability: The results of cluster sampling may vary greatly depending on which clusters are chosen.
Key Differences Between Stratified and Cluster Sampling
While both stratified and cluster sampling involve dividing the population into groups, they differ significantly in purpose and approach. Here are some of the key distinctions between the two methods:
Purpose of Division
- In stratified sampling, the population is divided into strata to capture diversity and ensure representation.
- In cluster sampling, the population is divided into clusters for logistical reasons, such as location.
Sample Selection
- Stratified sampling selects individuals from each subgroup within the population, focusing on proportional representation.
- Cluster sampling selects entire clusters at random, often choosing a smaller number of groups but including all members within those groups.
Cost and Efficiency
- Stratified sampling can be more costly and time-consuming as it requires data collection from all strata, often dispersed widely.
- Cluster sampling is generally more cost-effective and faster, as researchers focus on specific clusters rather than individual sampling.
Accuracy and Precision
- Stratified sampling is often more accurate, especially when comparing subgroups as each segment is represented.
- Cluster sampling may be less precise due to potential variability within clusters and limited representation of all population characteristics.
Choosing Between Stratified and Cluster Sampling
When deciding between stratified and cluster sampling, researchers should consider factors like population diversity, cost, and research goals.
Strategic sampling is generally preferred when it’s crucial to compare specific subgroups and ensure all segments are represented proportionately. However, it requires more resources and may be complex to implement.
Cluster sampling, on the other hand, is more practical and economical for large-scale studies where geographic dispersion is a concern. Although it may involve some bias risk, it enables quick and efficient data collection, especially when the focus is on overall trends rather than subgroups.
Conclusion
Stratified and cluster sampling are powerful techniques that can greatly enhance research efficiency and data accuracy when applied correctly. By understanding the unique strengths, limitations, and applications of each method, researchers can select the right approach to suit their needs, ensuring that their findings are representative, reliable, and insightful. Whether it’s capturing diverse perspectives through stratified sampling or simplifying logistics with cluster sampling, both methods play vital roles in modern research across fields, from healthcare and marketing to education and social sciences.