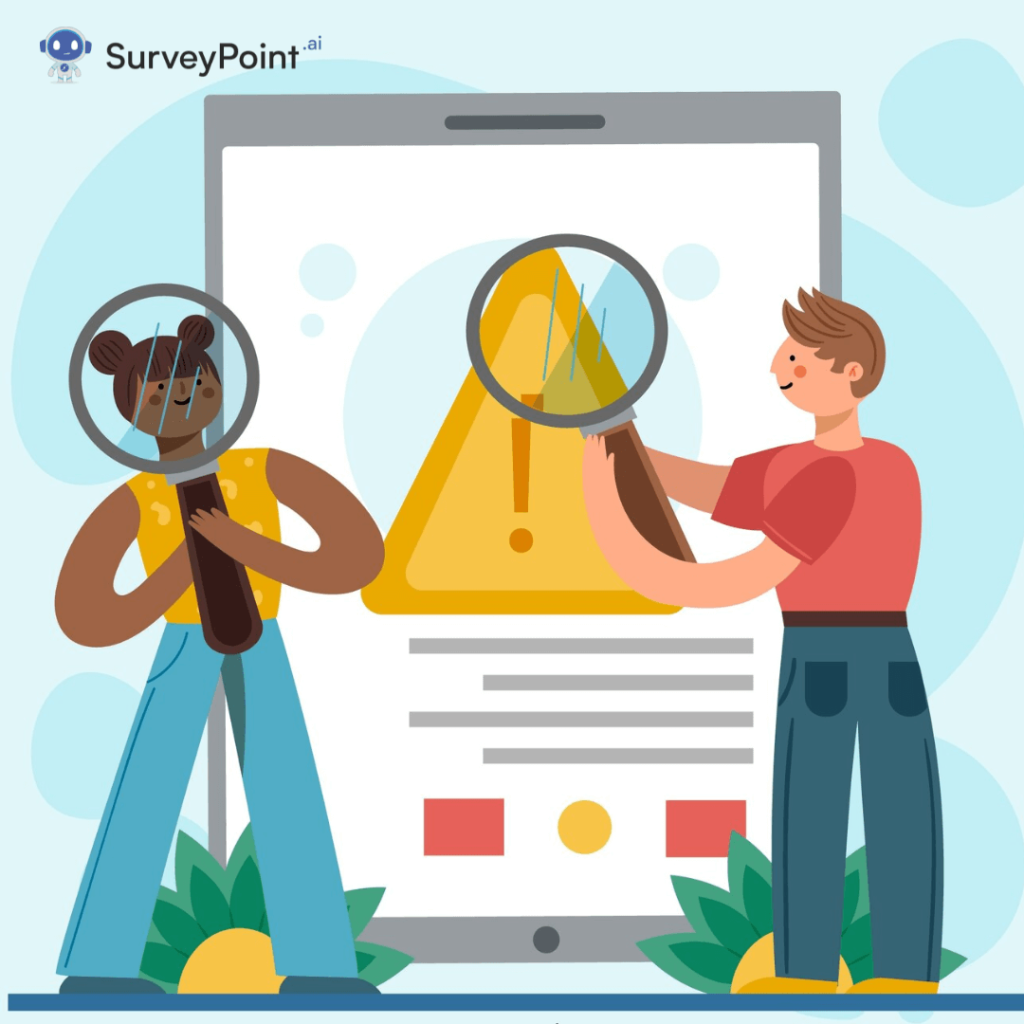
In statistics and data analysis, the margin of error (MOE) is a crucial concept that quantifies the amount of uncertainty associated with sample estimates of a population parameter. Whether you’re conducting a survey, analyzing experimental data, or evaluating a business metric, understanding how to calculate and interpret the margin of error is vital for making informed decisions.
This blog aims to provide a detailed guide to determining the margin of error, exploring the theoretical foundations, practical applications, and real-world implications of this statistical measure.
Understanding the Margin of Error
The margin of error is a statistical expression of the amount by which the sample estimate may differ from the true population value. It serves as a buffer zone around a sample statistic, often the mean or proportion, within which the true value is likely to lie.
For example, in a political poll stating that “Candidate A has 52% support with a margin of error of±3%,” this means the actual support for Candidate A is likely between 49% and 55%.
Key factors that influence the margin of error include:
- Sample Size: Larger samples typically result in smaller margins of error.
- Confidence Level: A higher confidence level increases the margin of error.
- Population Variability: Greater variability leads to larger margins of error.
The Formula for Margin of Error
The formula for calculating the margin of error depends on the parameter being estimated (mean or proportion) and the sampling method. The general formula is: Margin of Error=Z×σSE\text{Margin of Error} = Z \times \sigma_{\text{SE}}
Where:
- ZZ is the z-score corresponding to the desired confidence level.
- σSE\sigma_{\text{SE}} is the standard error of the estimate.
1. For Sample Means
When estimating a population mean (μ\mu) using a sample mean (xˉ\bar{x}), the margin of error is: MOE=Z×σn\text{MOE} = Z \times \frac{\sigma}{\sqrt{n}}
Here, σ\sigma is the population standard deviation, and nn is the sample size.
If σ\sigma is unknown, it is replaced by the sample standard deviation (ss). For smaller samples, the t distribution is used instead of the z distribution.
2. For Sample Proportions
When estimating a population proportion (pp) from a sample proportion (p^\hat{p}), the margin of error is: MOE=Z×p^(1−p^) n\text{MOE} = Z \times \sqrt{\frac{\hat{p}(1 – \hat{p})}{n}}
This formula accounts for the variability in categorical data.
Steps to Determine the Margin of Error
- Define the Confidence Level
Choose the confidence level based on the desired certainty, typically 90%, 95%, or 99%. A higher confidence level implies a larger z-score. - Calculate the Standard Error (SE)
The standard error measures the variability of the sampling distribution. Use the appropriate formula depending on whether you are analyzing means or proportions. - Find the Critical Value (Z or t)
Determine the critical value corresponding to the confidence level. Use the z-table for large samples or the t-table for smaller samples when the population standard deviation is unknown. - Multiply Z or t by SE
Finally, calculate the margin of error by multiplying the critical value by the standard error.
Practical Example
Scenario: A researcher surveys 400 people to estimate the average amount spent on groceries weekly. The sample mean is $150, with a standard deviation of $20. Determine the margin of error at a 95% confidence level.
Solution:
- Confidence Level: 95% implies Z = 1.96Z = 1.96.
- Standard Error:
SE=sn=20400=1\text{SE} = \frac{s}{\sqrt{n}} = \frac{20}{\sqrt{400}} = 1
- Margin of Error:
MOE=Z×SE=1.96×1=1.96\text{MOE} = Z \times \text{SE} = 1.96 \times 1 = 1.96
Thus, the margin of error is $1.96. The true average grocery expenditure is likely between $148.04 and $151.96.
Factors Affecting Margin of Error
- Sample Size: Larger samples reduce variability, decreasing the margin of error.
- Population Standard Deviation: Higher variability increases the standard error, leading to a larger margin of error.
- Confidence Level: A higher confidence level expands the range within which the true parameter lies.
Applications of Margin of Error
- Surveys and Polling: Ensures accuracy in public opinion polls.
- Business Analytics: Helps evaluate key performance metrics.
- Scientific Research: Validates experimental findings.
- Quality Control: Monitors product or service consistency.
Limitations of the Margin of Error
- Assumes Random Sampling: Non-random samples may introduce bias.
- Ignores non-sampling errors: Issues like survey design flaws or respondent bias are not accounted for.
- Depends on Correct Assumptions: Requires accurate estimation of population variability and a well-defined sampling frame.
Conclusion
The margin of error is an indispensable tool for quantifying uncertainty in statistical estimates. By understanding its determinants—sample size, variability, and confidence level—researchers can design more reliable studies and make better-informed decisions. Whether applied in academic, business, or practical contexts, the margin of error provides a critical measure of precision in data analysis.
Mastering the calculation and interpretation of the margin of error empowers analysts to communicate findings effectively and build trust in their conclusions.