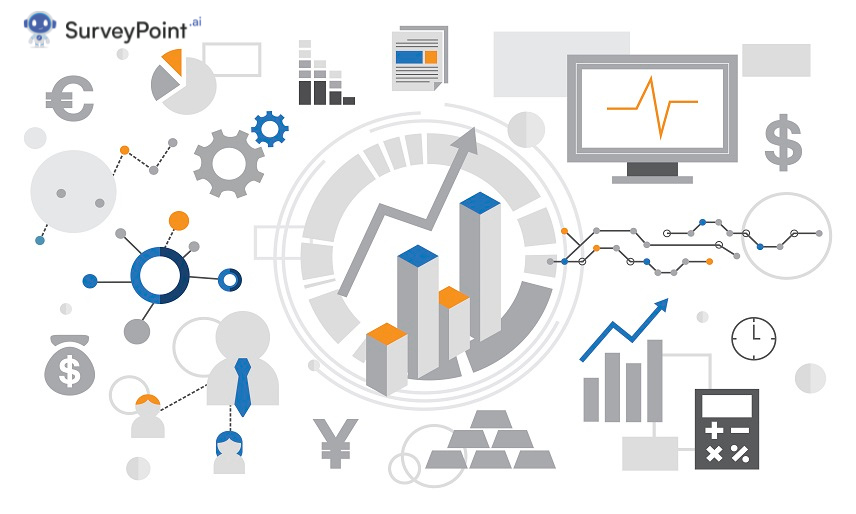
A descriptive analysis is the standard starting point for any severe statistical study. Seeing the connections between variables and thinking critically about using your data puts you at the forefront of future statistical analyses. Processing information allows it to be read, sorted, and analyzed in meaningful ways.
Descriptive analysis is one of the essential steps in analyzing statistical data. To prepare for more statistical analysis, it helps you conclude how the data is spread out, finds flaws and outliers, and shows how variables are related.
This blog will talk about descriptive analysis meaning, types, and descriptive analysis examples.
Descriptive Analysis: Meaning
Descriptive analysis, also called descriptive statistics or analytics, uses statistical methods to describe and summarize information. People like descriptive analysis to look at data because it gives easy-to-understand insights from raw data.
When looking at data, forecasting is more important than describing what the data means. Instead, it only looks at data from the past, which it then changes to find helpful information.
Descriptive analysis is a part of statistics that tries to help find patterns by explaining, illustrating, or summarizing the data points.
It’s the process of using both data sets to conclude the past and the present. It is often thought of as the most basic way to analyze data because it only looks for patterns and relationships and doesn’t go any further.
This kind of analysis is helpful when trying to explain something that happens over time. It uses observed regularities as a springboard for more investigation to guide deliberate choice-making. They are simple and convenient if completed in an organized manner.
Types of Descriptive Analysis
There are many different categories, traits, and indicators that may be used to describe descriptive analysis. According to some scholars, there are two distinct varieties. A few people claim four. We shall settle on four categories with the concept of using medians in mind.
- Distribution takes care of the frequency of occurrence of each value.
- It is the average of the numbers that make up the central tendency.
- Variability (or dispersion) measures how far values tend to fluctuate.
- Measures of position
Distribution (sometimes called Frequency Distribution)
Data sets include a collection of values or ratings. Statisticians use graphs and tables to describe the frequency of all potential values of a variable, expressed as percentages or numbers.
Statisticians often use either a graph or a table to display frequency distributions.
Measures of Central Tendency
The mean, median, and mode are all measures of central tendency that may be used to determine the average or center of a dataset.
Mean:
The mean, or “M,” is the most often-used statistic for calculating averages. The average is calculated by summing all the replies and then dividing by the total number of responses (the “N”). Let’s imagine somebody is trying to figure out how many hours they sleep every day over a week. The hours (e.g., 6, 8, 7, 10, 8, 4, and 9) would make up the data set, with a total of 52. N = 7, as there are seven comments. By dividing the total value, 52, by the number of digits in N, 7, we obtain the value of M, which is 7.3.
Mode:
The mode represents the most often reported value. Any range of modes, even “zero,” may exist in a dataset. Searching for the most frequent answer after sorting your data set from lowest to highest will help you locate the mode. According to the results of our sleep research from the previous section, then: 4, 6, 7, 8, 9, 10. Because of this, we may deduce that eight is the most common value.
Median:
The median, or midpoint, is the middle value in a data set. Find the median by sorting the data from lowest to highest.
Variability (also called dispersion)
The statistician can get a sense of how dispersed the responses are thanks to the measure of variability. The spread’s three components are the range, standard deviation, and variance.
Range:
To find out how far apart the two extreme numbers are, use the “range” function. To begin, divide the dataset’s minimum value by its maximum value. We refer back to our sleep research once more: 4,6,7,8,9,10. The result of taking six away from ten (the maximum) is four (the minimum).
Standard Deviation:
It will need more time to perfect this feature. You can see how far each score is from the mean by looking at its standard deviation (s), which is your dataset’s average level of variability. The more dispersed your data is, the higher your standard deviation will be.
Variance:
The variance measures the dispersion of the data. More dispersed data will have a higher variation from the average. The variance is just the square root of the standard deviation.
Measures of Position
Finally, the descriptive analysis may determine where something stands with other events or responses. Percentiles and quartiles are helpful metrics to apply in this situation.
You Must Read: Twitter Sentiment Analysis Online: Meaning, Importance, and Steps Involved
When Should You Consider Descriptive Analysis?
Descriptive analysis is often used to look at past or present data. This is because descriptive analysis gives much more targeted measurements than raw data, which can be hard to understand and analyze.
As an alternative, descriptive analysis can be done before diagnostics or predictive analysis to learn more about what happened in the past before trying to figure out why it happened or what will happen in the future.
How to Do a Descriptive Analysis—Step by Step
Like many other types of analysis, descriptive analysis leaves much room for interpretation. In other words, you can focus your analysis on the most important details. A descriptive analysis follows a set of steps that are always the same.
Collect data
Getting information is the first step in any data analysis. This can be done in several ways, but the most common are surveys and measurements.
Clean data
When using data for any study, the first step is always to clean the data. This is because the data may be stored in a way that makes statistics challenging. To clean data, you can reorganize it into smaller pieces that are easier to work with, add new categories, or get rid of any outliers.
Apply methods
Lastly, descriptive analysis uses your chosen statistical tools to get the desired results. The method you use will depend on the data you have and your goals.
Descriptive Analysis Examples
Consider an insurance provider that looks at its customers as an example of a descriptive analysis.
Insurers may collect demographic information from policyholders, including gender, age, and country of origin. The insurance firm might use descriptive analysis to learn more about its clientele.
- How many consumers are under a specific age can be determined using a measure of frequency.
- The majority of customers can be determined using a measure of central tendency.
- The range of ages their customers fall into can be determined using a measure of dispersion.
- Segments of customers can be compared using a measure of position.
Final Take
One common approach to analyzing data is called “descriptive analysis.” Since its sole purpose is to explain and summarize existing data, descriptive analysis is typically carried out before more involved forms of analysis, such as diagnostic or predictive analysis.
The descriptive analysis does this through several statistical tools, namely the central tendency, frequency, position, and dispersion measures. Your research questions will determine the specifics of your data collection, cleaning, and analysis for descriptive analysis.