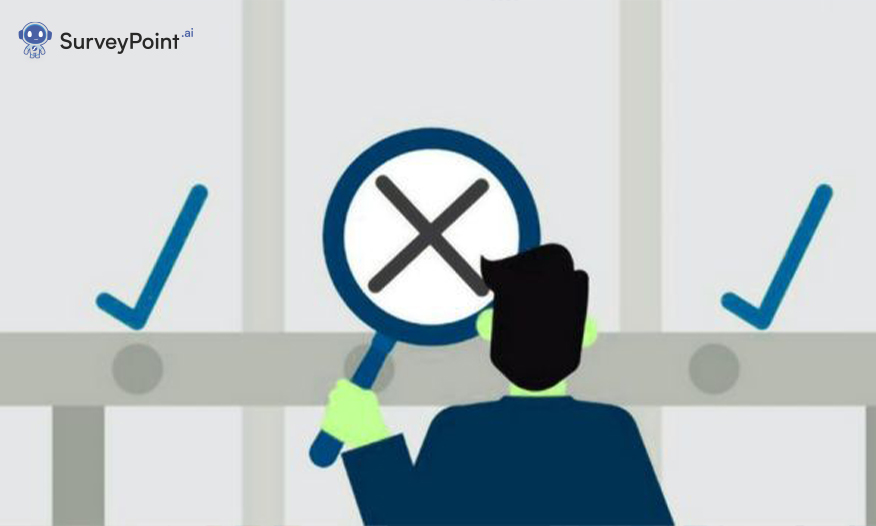
As part of scientific inquiry, researchers conduct statistical tests to determine whether or not to reject the null hypothesis. Parametric and nonparametric tests are the most commonly used inferential statistics measures.
We will explore the statistical test for hypothesis testing, touching on both parametric and nonparametric techniques, as well as highlight the primary distinctions between the two. Let’s start out by talking about parametric tests.
Parametric Tests
This method is employed when dealing with continuous or parameterized data. For example, blood pressure and temperature can be defined as continuous data because their vertices are on a scale. Typically, parametric tests are used when a normal distribution is assumed to apply to the data.
Testing the normal distribution of data before employing the parametric test
You can check normality using a histogram, simply a frequency plot of the frequency values you have for each point on a scale. Typically, if this is nearly symmetrical, you may assume that the data is generally normally distributed and perform a class of tests called parametric tests. A parametric test can be performed if you consider that the data within each group follows a normal distribution.
By assuming that the variable of interest is normally distributed, parametric tests provide more statistical power to estimate value and identify group differences. Sometimes, when the original variable has a non-normal distribution, the variable of interest is changed to make it appear more regular. In the next step, parametric statistics are applied to the modified variables.
Related: Proven Tips to Improve Quality of Research Surveys
Now, how can you modify or transform the data?
To prevent zero values, one can take the log of the data or the log plus a small value for a skewed distribution to the left. Sometimes, if there was an error in recording the data or something was off with that specific measurement.
If that’s the case, it’s reasonable to exclude potentially misleading data points from the analysis. Compare findings from studies with and without the outlier at least once to assess its significance. However, the tests may lead to misleading results if the data’s normality is uncertain.
Nonparametric Test
Now let’s discuss the nonparametric test. The intention behind nonparametric tests was to create a test which does not require any parameters. These techniques are often known as distribution-free or parameter-free techniques.
As a result, nonparametric approaches do not rely on estimating parameters to describe the distribution of the target variable in the population, such as central tendency and dispersion measures. A nonparametric test is used when data do not conform to the assumptions of a parametric method.
Related: Stratified Random Sampling: A Microscopic Introduction
Uses Cases
Nonparametric and parametric tests often yield similar results. Additionally, there is a parametric test for every nonparametric test. The t-test can be used when there is only one sample in a parametric study, which is equivalent to one nonparametric test. Chi-square tests, on the other hand, can be used for extremely small sample sizes in nonparametric studies.
Taking the example further, researchers can utilize repeated-measures ANOVA as the parametric test when there are more than two variables. If the variables in a sample do not have parametric distributions, Friedman’s two-way analysis of variance can be used.
Need help streamlining your research analysis?
Explore our solutions that help researchers collect accurate insights, boost ROI, and retain respondents.
Frequently Asked Questions – FAQs
Q-1: When could it be better to use a nonparametric test instead of a parametric one?
Ans. Nonparametric tests are more generalizable and more robust than parametric ones since they do not assume a particular distribution.
Q-2: In what ways are parametric tests beneficial?
Ans. Parametric tests are based solely on statistical evidence, so they are more likely to be accurate.
Q-3: When performing parametric and nonparametric tests, what value of central tendency should we consider?
Ans. Nonparametric tests use the median, while parametric tests use the mean.
Q-4: When should a parametric test be used?
Ans. The T-test and the Z-test are two common parametric tests in statistics.
Q-5: Give some instances of nonparametric tests.
Ans. Two-way ANOVA, Kruskal-Wallis, and Mann-Whitney.
Let Us Do The Maths for You
“Basic Statistics + Comparative Analysis = Tangible Solutions“
Seeing a difference between two numbers is easy, but determining whether that difference is statistically significant takes a little more effort. Especially if your question has several possible answers or you’re comparing findings from different groups of respondents, the process can be tricky.
Don’t refrain from investing in the proper technologies to alleviate the burden of manual analysis. Take the hassle out of the equation and let SurveyPoint do all the heavy lifting for you.
Kultar Singh – Chief Executive Officer, Sambodhi