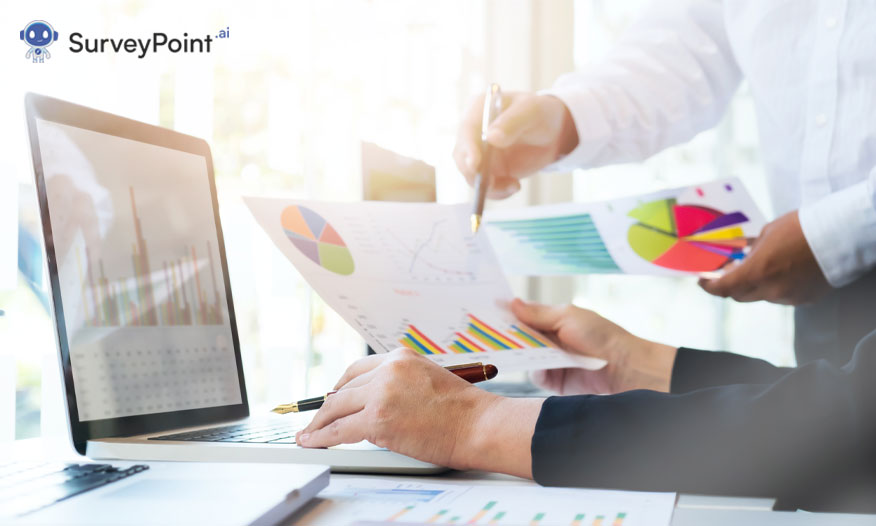
Did you know that there are a total of 4 sorts of data that offer different levels of measurement? These four types are ordinal, nominal, interval and ratio. In addition to covering the basics of working with raw data, this article will also provide an introduction to nominal data analysis.
Here’s an overview of different forms of data.
What are the four different types of data?
The four data types, nominal, ordinal, interval and ratio, helps you understand how a data point can be structured and recorded to make the most out of the information collected.
Nominal data is the least complex, whereas ratio data is the most difficult to navigate.
- Nominal Data: This data type demonstrates information that is divided into different labels or categories. These categories or labels do not have any quantitative value and are highly descriptive.
- Ordinal Data: This data form involves collecting and categorizing data in a specific hierarchical manner. Unlike nominal data analysis, where hierarchical data analysis is not required, ordinal has everything to do with placing all the categories in a particular way.
- Interval Data: Interval data include categorized and ordered information, which is similar to ordinal data. However, data with ordinal intervals do not include equally spaced intervals between the categories, while data with intervals does.
- Ratio Data: All ratio data points are spaced at equal intervals and are categorized and rated. Moreover, it has an absolute zero, so even if a data point is null or lacking in information, ratio data can still classify it or assign it a value of 0. Using this method, you can distinguish between valuable and useless data points.
How To Do Nominal Data Analysis?
It’s true that nominal data is purely descriptive. However, it can still be analyzed if you follow the steps below.
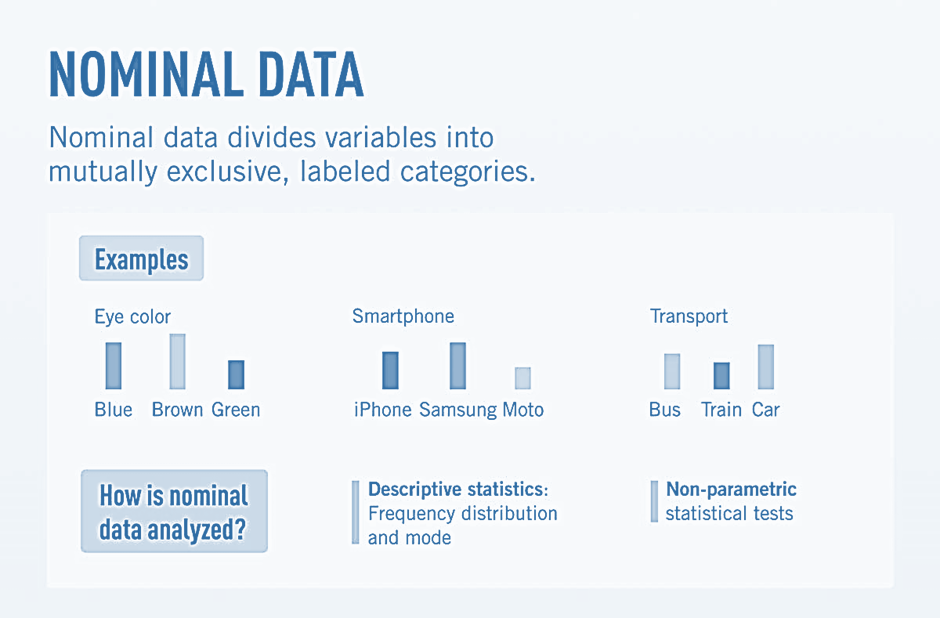
Step 1: Descriptive Statistics
Descriptive data helps you determine how to categorize or distribute the data. And when it comes to nominal data, you can use the following two statical techniques:
a. Frequency Distribution Tables
Let’s say the information relates to New Yorkers’ preferred form of public transportation. The raw data will consist of categories for the “Location” in New York and the “Preferred mode of transport.”
Bus, tram, etc., could fall within the first group. Another option is to live in the suburbs or the inner city. The data is unstructured since there is no mechanism to organize these in a hierarchy.
At first glance, you can’t see how the data is segmented out. Therefore, we can’t tell how many people would instead take a “bus” than a “tram,” making it impossible to compare the two and draw conclusions about which is better.
We must create a frequency distribution table in order to determine this.
b. Central Tendency Measurement (Mode)
As determined by the central tendency measure, or mode, the value that frequently appears in a dataset is the “center point” of the entire dataset.
The only appropriate measure of central tendency for nominal data is the mode. Look for the most common category in the distribution table to locate it. For instance, let’s say in our example, “Bus” received the most responses (11 out of 20). Thus, that is what we would use as our mode.
We can see how to use the mode and frequency distribution tables to get a general idea of our nominal dataset. Although it’s not a complete analysis, it gets the job done. Based on descriptive statistics, we can identify the most popular mode of transportation.
Step 2: Visualize The Nominal Data
The next step involves portraying the data collected in the form of visuals. Now it could be anything like a bar graph or even a pie chart. Visualizing the data allows you to understand the data more quickly and make effective decisions through easy navigation.
You can either access MS Office to make pie charts and bar graphs or choose an online survey builder tool like SurveyPoint to make the best quality visuals to keep things interesting.
Step 3: Statistical Analysis
The above two steps will only help you summarize the data for regular analysis. However, if you wish to dig deeper into the data and make it more useful, you can use statistical analysis techniques.
In the case of nominal or ordinal data, non-parametric statistical tests are generally preferred. You can do a chi-square goodness of fit test if there is only one variable. However, if you are working with two variables, you can run a chi-square test of independence. Here’s an overview of both techniques:
a. Chi-Square Goodness Of Fit Test
In this section, you will figure out whether the data you collected represents the whole population.
Let’s take a look at the above transportation example. If we assume that the most common transportation choice in New York is the Bus, the chi-square goodness of fit test will help you determine if the total audience shares this preference.
b. Chi-Square Test Of Independence
Use the chi-square test of independence to examine the relationship between two nominal variables.
Returning to our earlier example, we gathered information about the respondent’s location (inner city or suburbs). You can perform a Chi-square test of independence to examine a relationship between people’s distance from the city center and their preferred mode of transportation.
By examining the frequency of each category for the first nominal variable, we can compare the frequency of classes across the two nominal variables.
RELATED: Parametric and Nonparametric Tests: Overview, Difference, and Application
Example Questions For Collecting Nominal Data
On a nominal scale, there is only one possible classification for any given response or piece of data.
Nominal information may be written down or represented numerically. However, if your data has numerical labels, you cannot sort the labels in any meaningful way or perform mathematical operations on them.
Here are a few examples of nominal data you can use to hit a home run:
1. Where in the state do you now reside? (Following this will be a pull-down menu with the names of the individual states.)
2. Which of the following do you often select as your preferred pizza toppings?
- Pepperoni
- Sausage
- Olives
- Pepperoni
- Onions
- Tomatoes
- Spinach
- More cheese
3. How would you describe your nationality? (Kenyan, British, Chinese, etc.)
4. Do you have a smartphone? (iPhone, Samsung, Moto g etc.)
5. What is your native language? (Hindi, Spanish, Dutch etc.)
6. What is your favorite genre for a movie?
- Romance
- Action
- Animation
- Musical
- Comedy
- Dramatic
- Thriller
The Takeaway
Researchers can benefit from collecting survey replies in the form of nominal data. It’s a quick and easy method with minimal financial outlay. Additionally, it gives the respondent more leeway in making selections and filling out the form.
Learn to work smarter, not harder!
Explore our solutions that help researchers collect accurate insights, boost ROI, and retain respondents.
Free Trial•No Payment Details Required•Cancel Anytime
Heena Shah – Content Writer at Sambodhi