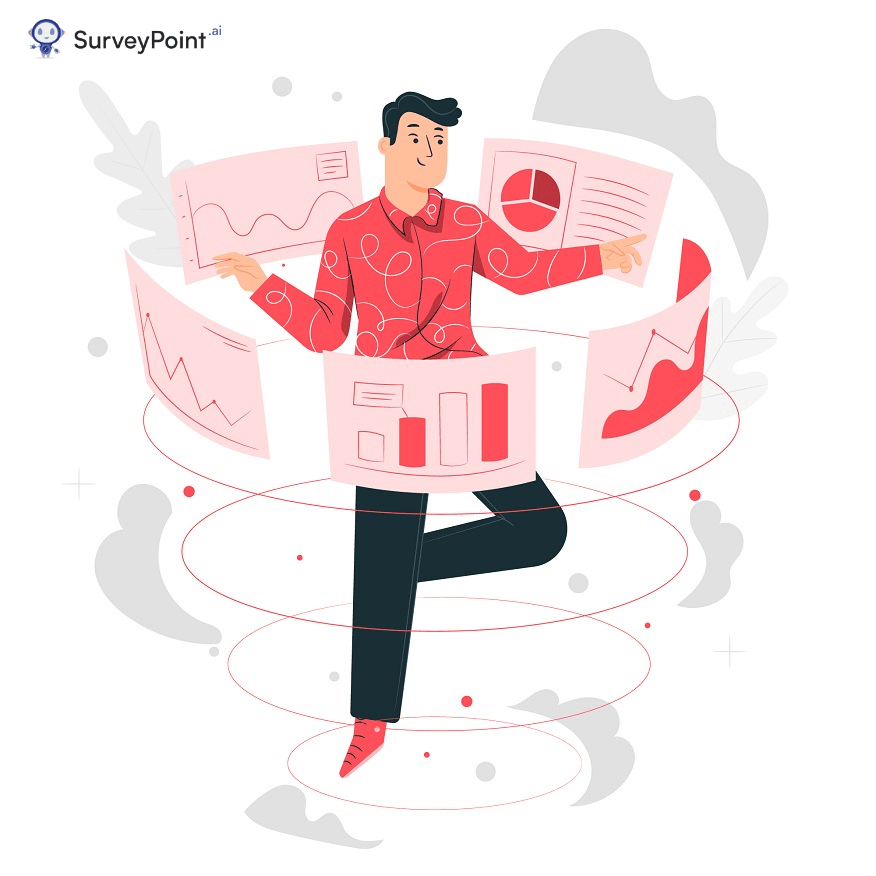
In the modern digital era, data is vital to companies and organizations. Data, however, are not all made equal. While some data may be incomplete, out-of-date, or purposefully falsified, other data may be precise, secure, and dependable. Data accuracy vs data integrity are two crucial aspects of data administration to guarantee that data is of excellent quality and suitable for use.
Despite their apparent similarity, they speak of various facets of data integrity. Data accuracy refers to how data accurately reflects what it depicts. Data integrity refers to maintaining data accuracy and consistency throughout its existence.
This article will examine the distinctions between these ideas and their significance, applications, and connections to data security and quality.
Data Accuracy vs Data Integrity
Although people occasionally use them interchangeably, data accuracy and data integrity represent two distinct ideas. Data accuracy refers to how close the data is to the true value or actual state of affairs. For instance, if the weather forecast calls for 25 degrees Celsius but the temperature only hits 24 degrees Celsius, then the data accuracy is 96%.
Contrarily, data integrity relates to the accuracy, uniformity, and completeness of data throughout its entire lifespan. Data integrity guarantees that information is not lost, corrupted, changed, or damaged during processing, storing, and transmission.
Miswriting a client’s name in a database can be considered a problem with data accuracy. However, a data integrity problem arises if a hacker gets illicit access to the information in the database and modifies the customer’s address.
ALSO READ: Popular Data Collection Methods To Draw Meaningful Conclusions
Data Integrity Examples
Let us understand the importance of the concept with data integrity examples.
Example 1:
Think about a medical facility that employs an electronic health record (EHR) system to keep track of patient data. For patient safety and high-quality treatment, the EHR system must guarantee that information about patients is accurate, complete, and uniform.
There could be severe consequences, including death if a doctor unintentionally inputs the incorrect medication dosage for a patient. Similarly, suppose a breach into the EHR system results in the compromise of patient data. In that case, that may constitute a privacy violation and harm the organization’s standing.
Example 2:
A bank’s customer database must be accurate, complete, and consistent to ensure that customer transactions are processed correctly. A customer’s incorrect account balance or the absence of their transaction history may result in financial losses and harm the bank’s image.
ALSO READ: The Impact of Big Data on Customer Experience in Banking
Data Accuracy Examples
To illustrate the importance of the concept, here are a few data accuracy examples –
Example 1:
Consider a financial institution that uses data analytics to identify fraudulent transactions. If the data used for analysis is inaccurate, the system may miss fraudulent activity. This can lead to financial losses for the institution and its customers. Conversely, the system can detect fraud quickly and prevent losses if the data is accurate.
Example 2:
Suppose data collection includes information on the median income in a specific region. In that case, it should be a reliable indicator of that area’s median income. Incorrect information may result in flawed research and decision-making.
ALSO READ: Are You Confident About Your Survey Data’s Margin Of Error?
Data Accuracy vs Data Integrity – Key Differences
Categories | Data Accuracy | Data Integrity |
Definition | It refers to how close the data is to the actual value. | It refers to data’s consistency, accuracy, and reliability over its entire cycle. |
Focus | Accuracy of individual data points | Overall consistency and reliability of the entire data set |
Importance | Important for decision-making and analysis | Essential for the effective functioning of an organization |
Issues | Inaccuracy may occur due to human error, system failures, or outdated data. | Data corruption, illicit access, and hardware or program failure can all jeopardize data integrity. |
Methods | It is measured using statistical methods such as mean and standard deviation. | It is maintained through access controls, data validation, error checking, and backup and recovery procedures. |
Goals | Real values and measured values should be as close as possible. | The objective is to guarantee that the data is correct, consistent, and whole across all applications and systems. |
Why is Data Integrity Important?
Data integrity is important for several reasons –
- It guarantees the accuracy and dependability of the data. This is crucial in healthcare, finance, and law, where choices can have severe repercussions.
- It aids in preventing data mistakes, omissions, and inconsistencies. This can result in greater effectiveness, lower costs, and superior decision-making.
- It guarantees adherence to rules and criteria. For instance, the FDA is very strict about data integrity during drug creation.
ALSO READ: Consequences of Poor Data Quality: Why It's Far Too Risky
Key Metrics of Data Integrity
Data Security
Despite similarities, data integrity and security are not the same. Data security is the defense of data against unauthorized entry, theft, or destruction. Firewalls, encryption, access limits, and backup systems may all be part of its defenses.
Data Quality
While the concept of data integrity is closely related to data quality, they are not identical. Data quality refers to the general suitability of data for a given goal. Accuracy, coherence, timeliness, relevance, and utility can impact data quality.
Data Consistency
Data consistency refers to maintaining uniformity of data across various platforms or sources. It is necessary to guarantee high-quality data, prevent mistakes and anomalies from entering data analysis, and make decisions based on that data.
Data Integrity Issues
Ensuring data integrity can be challenging, and many potential issues can arise. Some common data integrity issues include:
- Human error – Mistakes or misunderstandings may lead to incorrect entry of data.
- Software errors – Bugs or glitches in software can ruin data formatting
- Outdated data – Data may become obsolete or irrelevant over time, reducing reliability and consistency.
Summary
Data accuracy vs data integrity are crucial concepts in data management. They are essential for making informed decisions, conducting analysis, meeting regulatory requirements, and maintaining customer trust and brand reputation.
Data integrity issues can lead to costly consequences, including legal and financial liabilities. At the same time, data consistency and data security are also important for ensuring high-quality data.
Not Sure Where To Begin?
Explore our solutions to discover what is most important to your customers,
clients, and prospects. And best of all – it doesn’t take any coding!
Free Trial • No Payment Details Required • Cancel Anytime