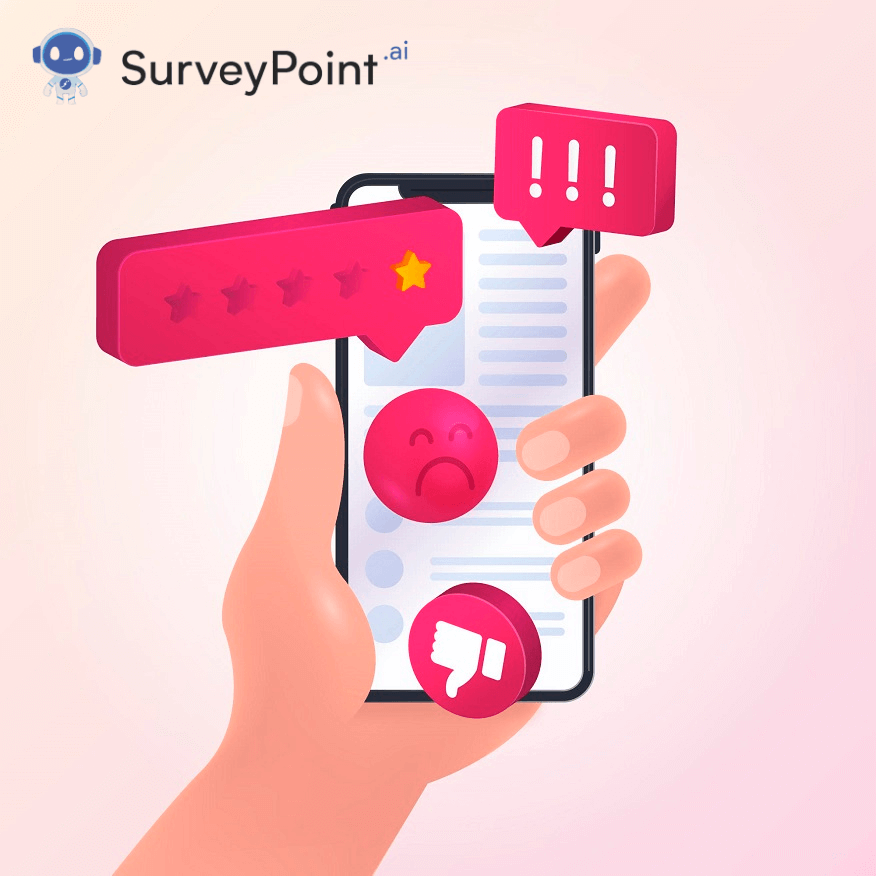
You’ve probably been there before if you do research. You and your team have spent weeks carefully planning the study, collecting data, reviewing results, and refining your conclusions. At some point, however, you will either need to pass up your work to other stakeholders or publish it publicly. As you exhale, you press the “Submit” button.
Sometimes things don’t go as planned, but usually, they turn out okay. After all, it’s not easy to ensure everything is perfect. The “what if” scenario is the most terrifying for a working professional. What if everything doesn’t go perfectly? But what if there’s a major problem in your work?
Since we are all fallible human beings, it is expected that people’s work occasionally includes defects and blunders. Due to the time and effort involved in research, you should only let the expense and effort of screening more participants cause you to collect accurate data. Online panels have a well-documented problem with data quality; the stakes are too high to proceed without rigorous respondent screening.
What Exactly Is “Poor Data Quality?”
If excellent quality data is defined as data that serve a particular goal, then poor quality data would serve no useful function. In other words, the data quality is insufficient to guarantee the desired results.
It’s possible that raw data is flawed information. As an example, Twitter data mined in its raw form is unstructured. Therefore not suitable for analysis or other analytical uses.
But it takes time to clean and process data, so starting with raw data can pay off in the long run.
RELATED: How To Fish Out Legitimate Surveys From The Scammed Ones?
The Repercussions of Inaccurate CDC Statistics
You likely don’t need to be reminded, but the beginning of the COVID-19 epidemic was very concerning. There were no reliable cures or vaccines because the virus was novel and research into it was limited. Amid soaring numbers of infectious diseases and fatalities, people had no idea how to protect themselves.
CDC published “Knowledge and Practices Regarding Safe Household Cleaning and Disinfection for COVID-19 Prevention” in June 2020 out of concern about how people could prevent COVID-19. The findings were very concerning. One month prior, 39% of Americans engaged in at least one high-risk behavior, as found in the study. In addition, four percent of people even use watered-down bleach, soap, or other disinfectants to clean their food.
Naturally, the results of this study received extensive coverage in the media. Many news sites reported within days that Americans were resorting to extreme measures against COVID-19, including swallowing bleach and gargling it. The news spread rapidly, but the conclusions were untrue.
The CDC hired a market research firm to compile their information. However, the vendor did not screen the respondents to verify their honesty or remove potentially problematic individuals who could skew the results.
Tips to Avoid Poor Data Quality
While considering the potential for professional shame is unpleasant, there are ways you can avoid such blunders. Including data quality metrics in your survey, doing a pilot study, and conducting a full-scale analysis are the three actions you may take.
Inquire about the steps your sample provider takes to address these concerns. You could also use a web-based survey tool.
1. Improve the Survey’s Results by Including Quality Data Metrics
If you haven’t already begun including data quality questions in your surveys, now is the time. However, most researchers consider including measures of attention and quality within surveys to be standard practice. The real question is not whether to add data quality measures for checks but whether to choose the right ones.
It is suggested that at least one open-ended question and a few brief measures of attention be included. Combining these numbers with other metrics of response patterns can help evaluate response quality.
2. Contact Your Sample Provider and Request Proof
Sample providers’ claims about their efforts to enhance data quality are all empty. What you need to know is whether or not your sample source has data to back up their claims about the efficacy of their methods.
The CDC research makes it evident that present industry standards need to be revised to guarantee data quality.
You may want to inquire about the following from your business associates or the provider of your sample:
- In whose hands does the sample rest?
- In what capacity is sample integrity ensured?
- Do we have to assume that some users responding are actually bots or scammers?
- How can we weed out respondents who aren’t paying attention?
- Exactly what type of screenings are you performing on respondents before they reach my survey?
- How can you know whether your screening procedures work?
3. Think About Utilizing SurveyPoint as a Means of Gathering Information
Both approaches can help, but preventing undesirable respondents from joining your survey is the most effective way to ensure high-quality results. Fortunately, SurveyPoint can help you accomplish this.
You can’t afford an error in your research. It can set a bad precedent for you and your company if respondents offer wrong, unreliable, or imprecise data, and these problems go unchecked. In order to prevent the repercussions of poor data quality, follow these three easy procedures.
RELATED: Proven Tips to Improve Quality of Research Surveys
Poor Data Quality FAQs
How do you define data quality?
Data quality is defined as the ability to produce up-to-date, accurate, consistent, and complete information.
How do typical data quality issues arise, and what are their root causes?
There are several potential causes for poor data quality, but the top three are human error, incompatible technology, and outdated or inaccurate information, all of which can have a significant impact on operational efficiency.
How do you know if your data needs to be fixed?
Some red flags for suspect information include:
- Various responses are given to the same inquiry.
- Opportunities are lost because of delayed insights.
- Performing simple tasks requires a lot of time and effort.
- Competing assessments of the company’s success.
- As a result of bad data, data migration projects consistently fail.
- Data warehouses and data lakes don’t provide reliable performance metrics.
- Many workers prefer to keep their own records because they don’t trust the central database.
- Important information is lacking.
- Data analysis is tricky.
Learn to work smarter, not harder!
Use our intuitive survey dashboard panel to identify respondents in even the most niche markets.
Free Trial • No Payment Details Required • Cancel Anytime