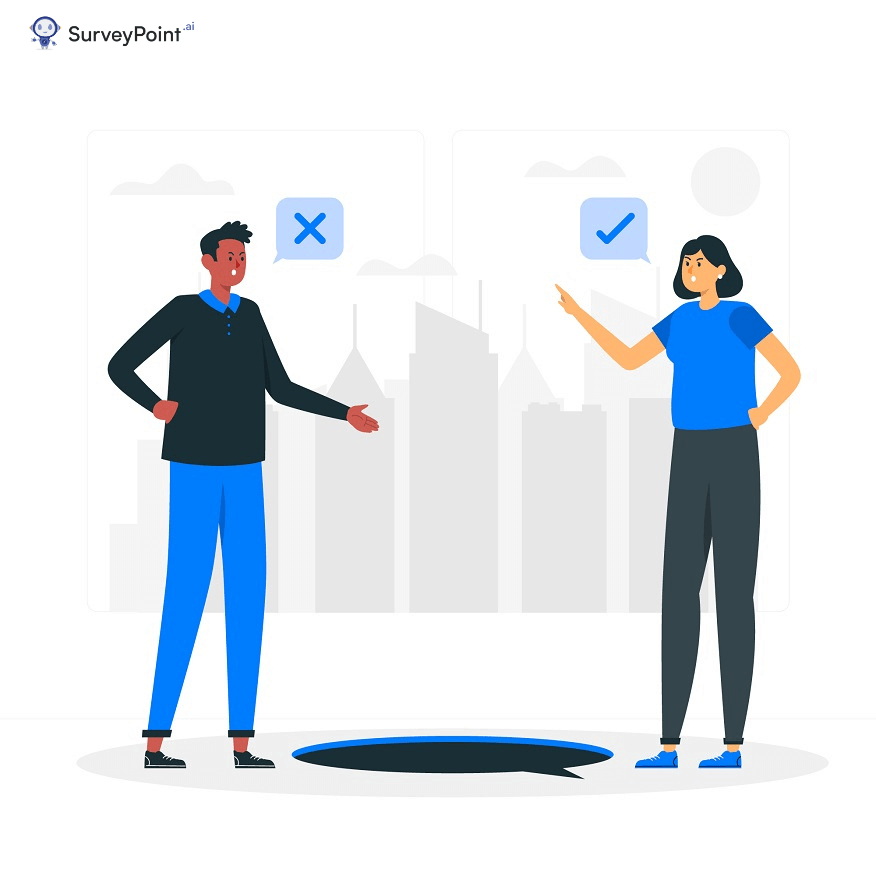
Sampling is a commonly used technique in statistical research. It involves selecting a subset of individuals from a larger population to represent it accurately.
However, things get tricky, as they always do when you analyze the results.
Errors such as sampling biases and sampling errors can pop up can turn your research or survey worthless if you’re not careful. Often these errors are thought to be the same and are used interchangeably.
Though these errors can produce unreliable results, the truth is they are poles apart. It raises forth questions such as, what is ‘sampling errors vs. sampling bias?’
And how can you prevent these errors?
Well, don’t worry. We’re here to help!
In this blog, we’ll go through the definitions of both terms, look at a couple of examples, and examine how these terms overlap and differ from each other.
Sampling Error Definition
Researchers use sampling errors to measure differences between a population’s characteristics and a sample’s.
You cannot avoid this error as you have a limited sample size. According to OECD, you’ll never be able to accurately represent the actual population as it is more diverse and larger than the sample size.
Example of Sampling Error
Assume that a researcher wants to estimate the average height of all individuals in a population using a sample of 100 people.
However, if this sample includes a higher number of taller individuals than the remaining population, it will cause an overestimation of the average height.
Sampling Bias Definition
Sampling bias in research results from choosing a sample that is not random or fails to represent the rest of the population. Similarly to sampling error, sampling bias also leads to inaccurate conclusions.
Sampling bias occurs when the research data is gathered unfairly. In such cases, certain individuals in the target population have a higher or lower chance of sampling probability than others.
This error often happens unintentionally and can be missed by the researcher. However, you can access the survey once it’s done.
ALSO READ: Representative Samples: Importance + Methods
Example of Sampling Bias
Suppose a researcher wants to study the level of physical activity among adults in a city. The researcher chooses to survey participants at a local gym during peak hours.
This sample, however, is likely to be biased.
Here’s why.
Because it targets individuals who are already active and have the time and resources to go to the gym. Moreover, this sample excludes other segments of the population like older adults, individuals with disabilities, and low-income individuals.
ALSO READ: The Right Time and Way To Do Quota Sampling
Types Of Sampling Bias
The table below mentions some of the key sampling bias types:
Type | Explanation | Example |
Self-Selection bias | People with particular characteristics are more likely than others to take part in the research. | People who like extreme sports or risk-taking are likely to participate in pain research studies. Thus, altering the research data. |
Undercoverage bias | When certain individuals are unfairly represented in the sample. | Any survey conducted on Twitter. As it underrepresents people who don’t have a Twitter account. |
Advertising or pre-screening bias | Pre-screening participants or the location where the research is advertised may bias a sample. | A political party advertising its polls in certain regions or to a specific demographic. It could result in a bias towards voters in those areas or belonging to those demographics. |
Non-response bias | When certain participants refuse to take part or drop out of the research. | A study on stress and workload. Employees with greater workloads are less likely to participate, thus skewing the data. |
Survivorship bias | Unsuccessful notions, people, or objects are less likely to be featured than their successful counterparts. | Ignoring the unsuccessful investments in favor of the successful ones. |
Healthy user bias | When a group of people in a study are healthier than the general population takes part in clinical trials. | People who take dietary supplements have a lower risk of developing cancer. However, this study might suffer from healthy user bias because people who take nutritional supplements are already health-conscious. |
Sampling Bias vs Sampling Error: Differences
The table below explains the differences between sampling bias and sampling error:
Sampling Bias | Sampling Error |
Occurs when the selected sample does not represent the entire population. | Occurs when you have a small sample size. Thus, resulting in poor precision. |
Occurs due to faulty or incorrect sampling methods. | Due to the fact that a sample never accurately represents the entire population. |
Cannot be measured precisely. | Can be estimated precisely only after the fact. |
Can be avoided by being extra careful. | Cannot be avoided unless you survey 100% of the population. |
Sampling Bias vs Sampling Error: Similarities
Now that you’re familiar with the differences between sampling bias and sampling errors, you must also understand their similarities.
- Both errors can lead to inaccurate conclusions and should be minimized to obtain reliable results.
- You can also reduce both errors by increasing the sample size and using proper sampling techniques.
ALSO READ: Biases in Surveys: What You Need to Know
How To Avoid Sampling Bias?
Completely avoiding sampling bias might just be a little too much. But you can control it to a certain degree. You can prevent bias sampling by following these tips:
- Clearly outline your target population and the sample frame
- Make sure that your sample frame accurately represents the target population
- Avoid long and complicated surveys
- Make your surveys accessible to the average individual
- Follow up on your initial survey
- Avoid convenience sampling as much as you can
- Define your survey goals
- Ensure your participants get an equal representation
Conclusion
Sampling error and sampling bias are two common pitfalls that are bound to occur in your research studies. While sampling error is due to chance, sampling bias is due to systematic errors in the sampling process.
But the good thing is you can minimize these errors through proper sampling techniques and careful consideration of the sampling frame.
By reducing these errors, you can obtain reliable results that accurately represent the population of interest.
Interested In Sending Your Own Surveys?
Explore our solutions that help researchers collect accurate insights, boost ROI, and retain respondents using pre-built templates that don’t require coding.