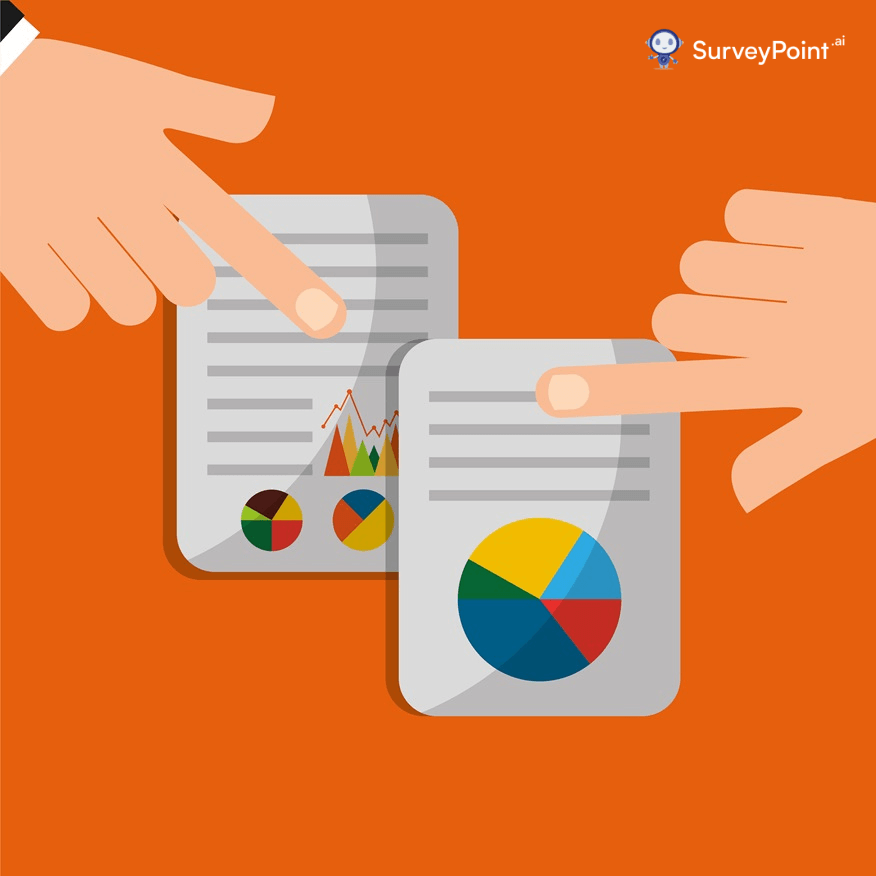
Significant data challenges researchers and analysts to extract valuable insights from various sources while providing data quality and reducing bias. Here’s where data sample blending comes in—a potent strategy that mixes various datasets to produce a cohesive analysis basis. But what does data mixing mean?
How can we guarantee the validity of the combined data and produce objective results? In this blog, we’ll explore data blending in the age of big data, its methods, and how researchers can extract valuable insights from multiple sources while ensuring data quality and reducing bias. Prepare to revolutionize your research efforts and demystify the technique of data blending.
Understanding Data Blending
Integrating various data sources to produce a single dataset for analysis is known as “data sample blending.” Researchers can get around the constraints of individual datasets and improve the quality and representativeness of their data by combining samples.
Integrating data from numerous sources, maintaining consistency, and removing potential biases are all part of the process. Researchers intend to get a complete and trustworthy dataset that offers a more precise grasp of the current survey question. Combining data samples allows them to use the advantages of many data sources and produce more reliable and insightful findings.
How to Ensure High-Quality Data?
You can use several strategies to ensure data quality during the blending process. Before combining the several datasets, thoroughly audit the quality and applicability of each dataset separately. Standardize variables and formats next to guarantee uniformity across the combined dataset.
Use data cleaning and preprocessing procedures to find and fix any errors, missing values, or outliers.
Perform data validation checks to confirm the combined data’s precision and reliability. Finally, to ensure transparency and promote reproducibility, record each stage of the blending procedure, including any modifications or changes. Together, these methods contribute to maintaining data quality throughout the blending process.
Strategies to Minimize Sample Bias During Data Blending
The systematic falsification of research findings caused by the non-random selection or representation of study participants is called sample bias. It might result in false or deceptive conclusions. Sample bias can be due to selection bias, underrepresentation of specific groups, or non-response bias.
When combining data, minimize sample bias to guarantee the validity and reliability of the results. The following are numerous methods to do this:
- Representative Sampling: Use representative sampling to ensure the blended dataset retains a proportional representation of various subgroups. For instance, when combining data from many regions, ensure each region’s data is appropriately weighted to reflect the size of its population.
- Stratified Sampling: Separately mix within each data stratum after dividing it into distinct groups (strata) based on pertinent criteria. For instance, stratify the dataset based on age groups or income levels when combining data on client preferences.
- Cross-Validation: To ensure that the blended dataset performs moderately across different data splits, use techniques like k-fold cross-validation to validate its performance.
- Techniques for Correcting Bias: Use statistical methods to fix biases in the combined data. For instance, use post-stratification weighting to match the sample with the demographic distribution of the population when integrating survey data with census data.
Best Practices for Data Sample Blending
You can combine several data sources through data blending to create a single dataset for analysis; You should follow the recommended practices throughout the data blending process to guarantee accurate and significant findings. Here are some important suggestions:
- Develop a Comprehensive Data Blending Strategy
Develop a solid data blending strategy to take advantage of the power of data sample mixing. Overcome obstacles, guarantee accuracy, and reduce biases. Use weighting, representative sampling, and preprocessing techniques.
Make wise decisions, uncover hidden trends, and produce ground-breaking outcomes to increase the credibility of your study findings.
- Select Appropriate Data Sources for Blending
For efficient data sample blending, select a variety of relevant data sources. Select trustworthy datasets to ensure they support your study aims.
Give quality, completeness, and compatibility of the data top priority. Combine datasets from different topics and viewpoints to maximize insights and generate solid conclusions.
- Validate the Blended Dataset
Thoroughly test the data sample blending’s blended dataset. Analyze the data’s dependability, accuracy, and consistency—Utilize statistical methods and visualization to find errors or outliers.
To guarantee validity, compare results with other datasets or the real world. The combined data results are assured, reliable and credible through rigorous validation.
- Maintain Transparency and Documentation
Maintain openness and thorough documentation throughout the data sample blending process. Clearly describe the data sources, blending strategy, and any changes used. Transparent documentation promotes trust, encourages teamwork, and allows others to verify and expand your work.
What are the Challenges of Data Blending?
Although data blending has many advantages, it also has several drawbacks and restrictions that researchers should be aware of:
- Data Integrity Problems
Every dataset utilized for blending could have problems with missing values, mistakes, or consistency. These problems may spread to the blended dataset and affect the accuracy and dependability of the final product.
- Sample Bias
The blending procedure may accentuate or introduce new biases into the combined dataset if the individual datasets are biased or not representative. This bias may result in erroneous or distorted conclusions.
- Privacy and Security Issues
They can arise when combining data from different sources, especially when dealing with sensitive or private information. Ensuring compliance with data protection laws and implementing suitable security measures is crucial.
- Interpretation Challenges
Combining datasets may provide new variables or combinations that are challenging to comprehend or interpret. Researchers must thoroughly analyze and evaluate the combined data to obtain relevant insights.
Conclusion
Unleash the power of data sample blending to unlock hidden potential and conquer biases! Dive into the realm of research, where combining diverse datasets is a game-changer. This article unveils the secrets to overcoming blending challenges and gaining invaluable insights.
With data blending, your conclusions will be laser-precise and objective due to impeccable data quality and intelligent sampling. Embrace the journey of reliable data, and let the art of data mixing no longer be a mystery!