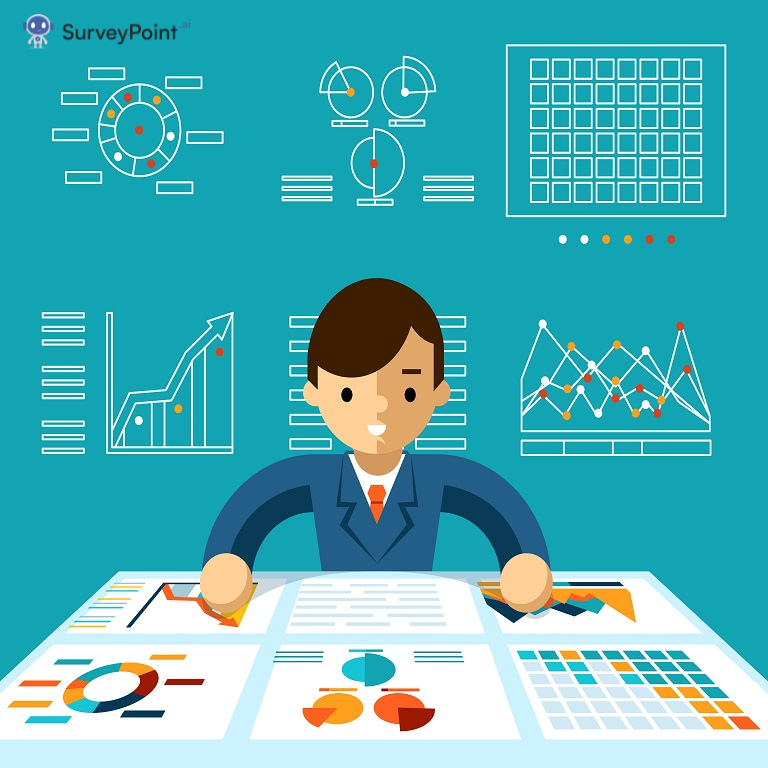
Cross Tabulation is a common technique to assess and gather data for a wide range of audiences. When it comes to swiftly examining and interpreting data, cross tabulations dominate. You can slice, dice, and otherwise organize your data to gain insightful information that will help you run your business more efficiently.
You’ll discover cross tabulation analysis in ways you never imagined after reading this article. We have thoroughly examined the advantages and disadvantages of cross tabulation for your research.
What is cross tabulation analysis?
Cross tabulation, sometimes known as cross-tabs, is a statistical technique used with categorical data. It is a mainframe statistical method that assists in your decision-making by seeing trends, patterns, and correlations between variables related to your research.
The raw data that comes with performing a study is frequently overwhelming. By identifying patterns, comparisons, and correlations between elements of your research that are mutually inclusive, cross tabulation assists you in developing a theory. A cross tabulation report demonstrates the relationship between two or more survey questions.
Why use Cross Tabulation Survey Analysis?
Researchers employ cross tabulation in survey analysis for the following reasons:
- Cross tabulation demonstrates how relationships between the variables differ between groups.
- Using cross tabulation, researchers can quickly spot patterns, trends, and probability in data sets.
- Cross-tabs allow researchers to examine connections within a specific data collection. If you use other methods of organizing the data, you can miss relations they can reveal.
- Cross tabulation presents both the survey findings and the results from subgroups.
Advantages of Cross Tabulation in Survey Analysis
Cross Tabulation is a preferred way for survey analysis because of the following reasons:
- Minimizes confusion. Large data sets can be made simpler by cross tabulation. It is simpler to conclude the data when it is organized in tables as a result. That’s one way it lessens the confusion of dealing with enormous data sets that most researchers face.
- Delivers data that can be acted on. You can find practical insights regarding your goals using cross-tabs. The insights may give you the encouragement you need to make adjustments. It can also provide information supporting your choices and the specifics you need to create more effective plans.
- Enables data insights. Having too much data might be overwhelming. Use cross-tabs to divide the data into more manageable sections to have better control over it. Cross-tabs make it simple for researchers to conclude the connections between categorical variables.
Disadvantages of Cross Tabulation in Survey Analysis
The cross-tab report has numerous limitations despite its effectiveness at summarizing data. Let’s look at a few of them.
- A tonne of information in the original grouping report needs to be displayed in the cross tabulation. The reason for this is that cross-tabs can only express summary computations.
- If the sample size is small, there may be fewer elements that can be cross-tabulated with one another.
- In the summary fields, only numbers can be printed. Text values are not permitted. This is due to the requirement that each cell computes a summary function, and summary functions can only produce numeric results.
- When there are several responses, cross tabulation can result in a vast number of tables. This is due to the various cross-tabulation methods that can be used with the variables.
Cross Tabulation Analysis Interpretation
Cross tabulation Analysis Interpretation talks about how you can report cross tabulation results. You can interpret the analysis with these two steps:
Step 1: Determine the statistical significance of the relationship between the variables.
To decide whether to accept or reject the null hypothesis (Ho), which claims that the variables are independent, use the p-value.
Compare the p-value to the significance level (also known as the alpha or α) to determine whether the variables are independent. A significance level of 0.10 indicates a 10% likelihood of drawing the incorrect conclusion that there is a relationship between the variables.
P-value ≤ α: There is a statistically significant correlation between the variables (Reject H0)
You reject the null hypothesis and conclude that there is a statistically significant relationship between the variables if the p-value is lower than or equal to the significance level.
P-value > α: cannot conclude that the variables are associated (Fail to reject H0)
If the p-value exceeds the significance level, the null hypothesis cannot be rejected since insufficient data support an association between the variables.
Step 2: Analyze the differences between the expected and observed numbers to identify the variable values that may affect the association most.
The exact number of observations in a sampling that are part of a category is known as the observed count. The average frequency anticipated in a cell if the factors were independent is known as the expected count.
By comparing the observed and expected cell counts, you can determine which factors have the most significant disparities, which may imply dependence. The variables with the highest discrepancy between expected counts and actual counts in relation to sample size can be identified by comparing the standardized residuals.
You Must Watch: A Comprehensive Guide to Race and Ethnicity Survey Questions
Cross Tabulation Analysis Example
Cross tabulation is frequently employed when categorical variables or data are present. It is helpful for material that may be categorized into categories that are exclusive of one another. Let’s look at some examples.
- Customer reviews broken down by region could be a category variable. You separate this data into reviews for each area of the country, such as the North, South, East, West, or individual states, and then you examine the connections between those evaluations.
- Product surveys are another situation where cross-tabulation is useful. 50 people could be asked, “Do you like our products?” to obtain a more insightful response, employ cross-tabulation.
You can include additional independent variables, such as gender, instead of simply collecting the 50 responses. Cross-tabulation is now a valuable tool for learning how respondents, who are both men and women, feel about your product.
Cross tabulation is an excellent tool for evaluating independent variables in market research or surveys. Finding the connection between two (or more) things that seem unrelated is easily accomplished by comparing data sets.
Conclusion
Many studies claim that cross tabulation is one of the most popular ways to analyze market research or survey data. It offers advantages, including more significant insights, by contrasting one data type to another. It is simple and practical in terms of interpretation.
Even a novice researcher can utilize cross tabulations in survey analysis because of their benefits and qualities. Utilize this analysis to increase clientele, broaden your service offerings, and elevate your output. Without a doubt, it is beneficial for revealing hidden connections in your raw data.