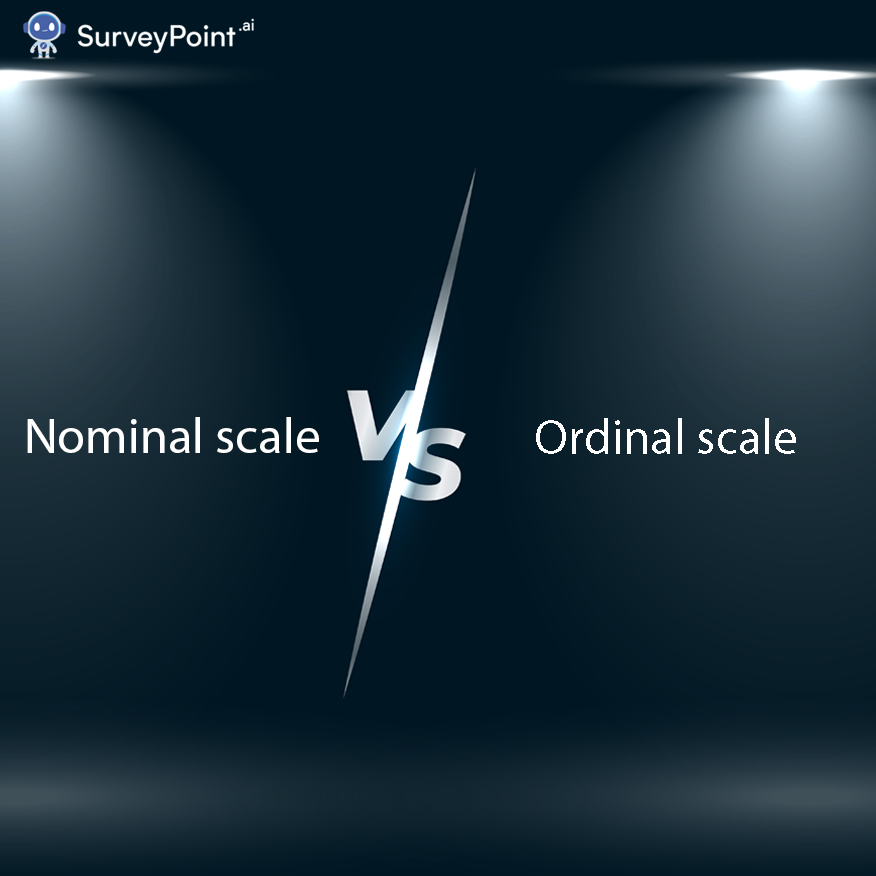
When it comes to analyzing your data, you must start by understanding its nature. In your dataset, it is possible to have a wide variety of variables. These variables can be calculated with different degrees of precision. This is what the level of measurement is called in Statistics.
Statistically, there are four primary levels of measurement: Nominal, Ordinal, Interval, and Ratio. And, if you are wondering about the Nominal VS Ordinal Scale debate, we are here to help you figure out what’s better with our points of difference.
The 4 Levels Of Measurement
As stated above, there are four levels of measurement in statistics.
- Nominal
- Ordinal
- Interval
- Ratio
Each measurement scale is based on one another. For instance, the ordinal scale includes whatever nominal scales include in addition to additional tactics. Thus, adding more precision to the measurement.
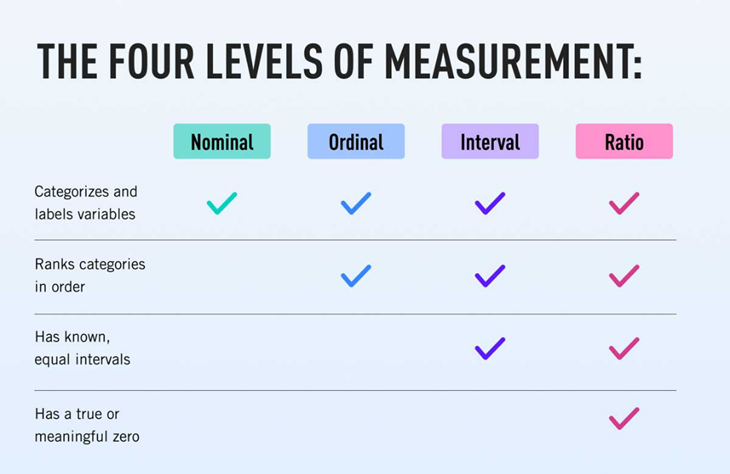
So, before we analyze the critical pointers of the Nominal VS Ordinal Scale, let’s briefly look at all four measurement scales.
Nominal Scale
These measurement scales categorize variables according to their names or qualitative labels. These groups don’t have any hierarchy or numerical value.
For instance, the grouping in a variable labeled “Hair Color” will be categorized into blonde, black, brown, red, etc.
Ordinal Scale
Along with categorizing the data based on their name, the ordinal scale also adds an element of the hierarchy. In short, it adds order to the data.
For example, researchers could measure a variable labeled as “Income” in an ordinal scale like low-income, medium-income, and high-income groups.
Interval Scale
In addition to categorizing the variables in a hierarchical form, the interval scale of measurement labels the variables with equally spaced intervals.
Here’s an example for a better understanding:
Let’s take a look at the interval data of converting temperature into Fahrenheit. On an interval scale, the difference between 10 and 20°F would be equal to the difference between 40 and 50 °F.
Ratio Scale
The ratio scale is just like the Internal Scale. The only difference, however, is the “True Zero.” Unlike the interval scale, this includes a Zero value, where the variable cited as Zero means nothing.
For example, when measuring weight, if something is 0 kg, it simply means that it weighs nothing.
Understanding Nominal Scale
Now that you have a basic understanding of the four types of measurement scales, let’s explore our main topic: Nominal VS Ordinal Scale.
Let’s start with the nominal measurement scale.
As a starting point, the nominal level of measurement is the simplest, clearest, and least difficult way to classify information. It simply divides the variables into a data set into different groups, depending upon their names.
In this variation, there is no quantitative meaning; the categorization is done simply based on qualitative labels.
In the above example of hair color, researchers can use “1” to represent blonde color and “2” for black.
Note that the groups can never be categorized hierarchically when dealing with nominal scale.
RELATED: Nominal, Interval, Ordinal Scale: A Guide to Measurement & Data Scales
Nominal Data Examples
Here are some examples of data that can be measured through a nominal scale:
- Hair Color
- Eye Color
- Nationality
- Gender
- Personality Type
Simply put, nominal data describes specific characteristics of a group.
RELATED: The Right Ethnicity Survey Questions For Your Questionnaire
Analyzing Nominal Data
You can use descriptive statistics like tables to analyze your nominal dataset. Besides tables, you can also use other statistical measures like the mode and frequency distribution table to summarize the responses for each grouping.
To analyze your nominal data through statistical tests, you can use the following two techniques:
- Chi-Square Test of Goodness Of Fit: this can be a good choice if you are dealing with a single variable. This test assumes the entire data is representative of the whole population.
- Chi-Square Test of Independence: This test is usually used to explore the relationship between two variables. For instance, analyzing hair color would be just one variable. However, studying hair color and texture would be two variables.
Understanding Ordinal Scale
Unlike nominal scale, ordinal scale is more than just categorizing the data set into different variables. In addition to doing this, this scale also ranks the variable, thus, creating a hierarchy.
Questions like Likert Scale are examples of an ordinal scale. As stated in the above income example, a researcher can use this scale to get an idea of who belongs to which income group. However, they can not determine the difference between the income of people belonging to the low-income group and the high-income group.
In short, no numerals are involved, making it a qualitative approach, like a Nominal scale.
Though it is more precise than the nominal scale, it still does not allow researchers to compare the inputs.
Ordinal Data Examples
Here are some examples of ordinal data:
- Happiness Scale
- Level of Satisfaction
- Academic Grades
- Income
- Level of Education
Analyzing Ordinal Data
Along with a frequency distribution table and mode, researchers can use other statistical measures like median and range to analyze ordinal data. Here’s a list of tests to analyze the ordinal dataset.
- Mood’s median test
- U-test
- Matched Pairs Signed-Rank Test
- Spearman’s Rho
Nominal VS Ordinal Scale: Key Differences
Factors | Nominal | Ordinal |
Meaning | In this scale, the data is grouped according to their names. There is no ranking on the nominal scale. | Along with grouping the data based on their qualitative labels, this scale also ranks the groups based on natural hierarchy. |
Quantitative Vale | There is absolutely no quantitative value in the variables. The grouping is done strictly on qualitative labels. | This scale includes quantitative values, however, to a limited level. The data is grouped according to a hierarchy but is not comparable. |
Ranking | The variables cannot be ranked. | The variables can be ranked in order. |
Examples | Gender, hair color, eye color, and religion. | Academic grades, social status, and education qualifications. |
The Takeaway
In conclusion, nominal and ordinal scales are both used to categorize data. Still, they differ in the level of measurement and the type of data they represent. Nominal scales are used for non-ordered categories, while ordinal scales are used for ordered categories.
Understanding the difference between nominal VS ordinal scale is crucial in data analysis, as it determines the appropriate statistical tests and the interpretation level that can be applied to the data. Properly identifying and utilizing the correct scale for your data can ensure accurate and meaningful analysis that yields valuable insights.
Learn to work smarter, not harder!
Explore our solutions that help researchers collect accurate insights, boost ROI, and retain respondents.
Free Trial • No Payment Details Required • Cancel Anytime