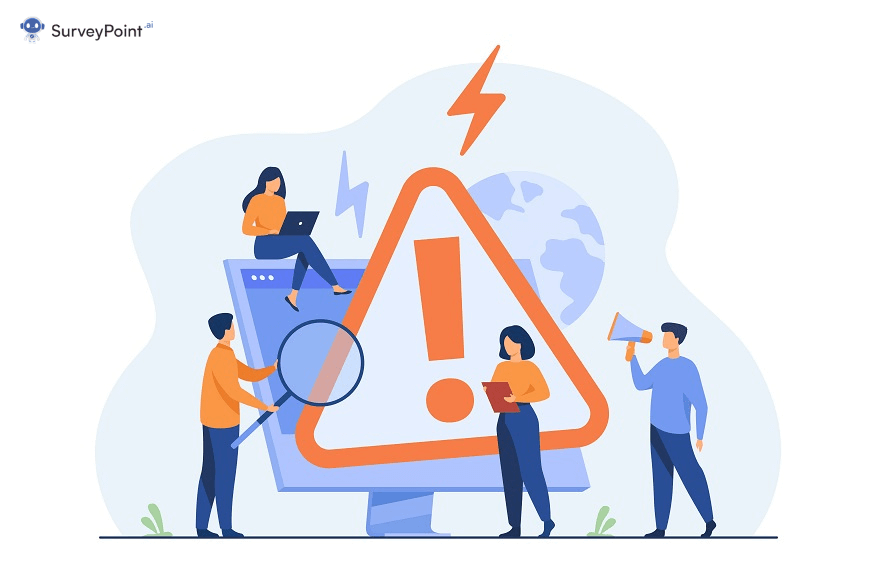
When conducting a survey, you must start by identifying your sample, which would represent the entire target audience. Sampling bias can be a pretty common issue.
It occurs when the sample picked does not represent your population, making them feel left out. Understanding such bias can be key to accurate research or survey analysis.
This article will navigate through different types of sampling bias and a few ways to avoid it.
What’s Sampling Bias?
Using an unrepresentative sample of the population in a survey can lead to sampling bias. In simpler terms, you identify a sample which some members having a higher or lower probability of getting surveyed.
This can affect the validity of your study and might offer inaccurate analysis. It can also limit the degree to which your study conclusion would match the patterns of your larger, targeted audience.
In most cases, sampling bias occurs unintentionally and is caused by specific sampling strategies that are convenient or purposeful.
RELATED: The Difference Between Sample Bias And Sampling Error
Some Common Causes
When it comes to sampling bias, there are two leading causes:
Poor Methodology
Let’s be honest: one of the best ways to identify your sample size is through random sampling. It is simple, easy and fast.
This method involves choosing samples from the entire population based purely on randomness. However, while setting other parameters, it is often common to insert their own bias, thus, disrupting the process.
Poor Execution
This happens when researchers develop the proper methods; however, the team working on identifying samples cuts corners. In such cases, researchers often select samples based on convenience rather than quality.
One of the best examples of sampling bias occurred in 1948 during the US presidential elections. During that time, a telephonic survey was conducted. However, they did not consider that telephones were a luxury at the time, and their sample was only made up of rich people.
Since the sample selected did not represent the entire US population, study results were inaccurate.
RELATED: Biases in Surveys: What You Need to Know
Different Types Of Sampling Bias
There is a wide variety of sampling biases. Here are some common types:
Undercover/Exclusion Bias
Whenever a portion of a population is ignored or inaccurately represented, this type of bias occurs. Undercover bias is evident in the above example of presidential elections.
In the present world, the same type of bias could occur if the state surveyed the population and didn’t include the elderly.
Similarly, convenience sampling may result in this problem. Participants who are easy to reach can be sampled using this method.
Voluntary Bias
This kind of bias occurs when study participants are more likely to exhibit certain features in respondents. In this instance, study participants give their consent to take part. Volunteers are more likely to have an opinion on the subject under investigation.
In contrast, some people choose not to volunteer to participate because they would rather not talk about the subject. As a result, too many people in the sample have strong opinions, and few don’t feel strongly about anything or don’t want to talk about it.
An instance of self-selection bias would be a survey asking people to rate products in which they have a choice. People are more likely to take part in the study if they have just had a significant emotional event, whether positive or negative. Omitting all possible client encounters skews the data. This bias is evident in the opinions of customers.
Survivorship Bias
In survivorship bias, the sample is concentrated on people who meet the requirements for selection. Those that fail are ignored, which results in underrepresentation.
For instance, if you simply ask existing customers to participate in your survey, their responses are more likely to lean favorably than if you also ask consumers who have stopped doing business with you.
Consumers must be pleased with their encounters because they have decided to keep engaging with your brand. For accuracy in your survey, you should include the perspectives of customers who no longer buy your items.
Non-Response Bias
Some respondents do not respond to studies or answer specific survey questions, resulting in non-response bias.
This can lead to inaccurate or incomplete data, as the responses may not represent the studied population. Some factors, such as survey design, respondent characteristics, or non-response follow-up procedures, can cause non-response bias.
To minimize non-response bias, researchers may use techniques such as weighting, imputation, or targeted follow-up to encourage participation from non-respondents.
Recall Bias
Recall bias is a measurement bias that occurs when participants in a research study have difficulty accurately remembering and reporting past events or experiences. This can result in systematic errors in the data, leading to inaccurate or biased conclusions.
Recall bias is often a concern in retrospective studies, where participants are asked to recall events or exposures that occurred in the past, such as in a case-control study or a survey.
An individual’s age, cognitive ability, and emotional state can contribute to recall bias, as can the period between the event and the recall.
Observer Bias
A systematic error made by researchers or observers during data collection, analysis, or interpretation is called experimenter bias.
This can happen when the researchers’ beliefs, values, or expectations influence their perception or recording of the data, leading to inaccurate or misleading results.
Researchers and participants can minimize observer bias by using blinding techniques, like double-blind experiments.
Exclusion Bias
Exclusion bias is a type of selection bias that occurs when specific individuals or groups are systematically excluded from a study or analysis.
This can happen when the selection criteria for participants or data sources are too narrow, leading to a biased sample.
Studies with excluded individuals may have different characteristics or experiences, affecting the generalizability and validity of the results.
Healthy User Bias
When participants in a study are healthier or have healthier behaviors than the general population, they are considered to be healthy user bias.
The health-conscious or proactive are more likely to participate in a study or seek medical treatment, which can lead to an overestimation of treatment effects or risk factor associations.
It can also affect the generalizability of study findings to the broader population.
Pro Tip: When conducting a survey, you can use an online survey-building tool like SurveyPoint to curate a survey within seconds. You can either use templates or start from scratch. A highly interactive dashboard and various custom analytical tools enable you to track the data in real time.
ALSO READ: Typeform VS SurveyMonkey: An Unbiased Comparison
8 Ways To Avoid Sampling Bias
While sampling bias is an involuntary phenomenon, here are eight tips you can follow to avoid it:
Define the population: Define the population that the study will represent. Sampling bias can occur when the sample is not representative of the population. Thus, it is essential to have a clear understanding of the population and the characteristics that are relevant to the study.
Use random sampling: Use random sampling techniques to select participants. Random sampling is a technique where each individual has an equal chance of being selected. Therefore, it is possible to ensure the sample is representative of the entire population.
Increase sample size: Increase the sample size to reduce sampling bias. Larger sample sizes help minimize the impact of outliers or unusual data points that can skew the results.
Use stratified sampling: Use stratified sampling to ensure that the sample includes individuals from different subgroups. This can help to ensure that the sample is representative of the population.
Blind sampling: Blind sampling is a technique where the researcher is unaware of the characteristics of the participants. Choosing participants this way can reduce the impact of bias.
Avoid voluntary sampling: Avoid voluntary sampling as it can result in a biased sample. This is because people who choose to participate may differ from those who do not.
Use multiple sampling methods: Use multiple sampling methods to ensure that the sample is diverse and representative of the population. As long as the sample includes individuals from different subgroups, bias can be reduced.
Consider sampling weights: Consider using sampling weights to adjust for differences in the population. Sampling weights are used to adjust each participant’s contribution to the final results based on their probability of selection.
Summing Up
In conclusion, sampling bias is a common issue in research that can significantly impact the accuracy and validity of the results. Researchers need to be aware of the different types of sampling bias and take steps to minimize their impact.
Researchers can minimize the risk of sampling bias by using appropriate sampling techniques, increasing sample size, and ensuring that the sample is representative of the population. It is essential to acknowledge and address any potential sources of bias in the research design to ensure the findings are reliable and applicable to the broader population.
Understanding and addressing sampling bias is critical in producing credible research that can inform decision-making and shape our understanding of the world.
Want to take the hassle out of your research analysis?
Explore our solutions that help researchers collect accurate insights, boost ROI, and retain respondents.
Free Trial • No Payment Details Required • Cancel Anytime